Spatial Division Multiplexing (SDM) MIMO processing can significantly improve spectrum efficiency and thus greatly increase the capacity of wireless communication systems. Spatial Division Multiplexing MIMO communication systems have recently attracted widespread attention as a means to significantly improve wireless system capacity and connection reliability.
The best hard-decision detection method for MIMO wireless systems is the maximum likelihood (ML) detector. ML detection is very popular because of its excellent bit error rate (BER) performance. However, the complexity of a straightforward implementation increases exponentially with the number of antennas and modulation schemes, making ASICs or FPGAs limited to low-density modulation schemes using only a few antennas.
In MIMO detection, the best approach that can maintain BER performance comparable to the best ML detection while significantly reducing computational complexity is sphere detection. This approach can reduce the detection complexity of SDM and space division multiple access systems while maintaining BER performance comparable to the best ML detection. There are many ways to implement sphere detectors, and each method has many different algorithms, so designers can find the best balance between multiple performance indicators such as throughput of the wireless channel, BER, and implementation complexity.
While the algorithm (e.g., K-best or depth-first search) and hardware architecture clearly have a huge impact on the final BER performance of a MIMO detector, the channel matrix preprocessing that is typically performed prior to spherical detection also has a huge impact on the final BER performance of a MIMO detector. Channel matrix preprocessing can be complex or simple, such as prioritizing the processing of spatially multiplexed data streams based on variance calculations of the channel matrix, or using very complex matrix factorization methods to determine a more ideal (in terms of BER) data stream processing priority.
Signum Concepts, a San Diego-based communications systems development company, has been working with Xilinx and Rice University to design a MIMO detector for spatial division multiplexing MIMO in 802.16e broadband wireless systems using FPGAs. The processor uses a channel matrix preprocessor to implement a continuous interference cancellation process similar to the Bell Labs Layered Space-Time (BLAST) architecture, ultimately achieving near maximum likelihood performance.
System Considerations
Ideally, the detection process requires the computation of ML solutions for all possible combinations of symbol vectors. The sphere detector aims to reduce the computational complexity by using simple arithmetic operations while maintaining the numerical integrity of the final result. The first step of our approach is to decompose the complex numerical channel matrix into an expression with only real numbers. This operation increases the matrix dimension but simplifies the computation of processing the matrix elements. The second aspect of reducing computational complexity is to reduce the optional symbols analyzed and processed by the detection scheme. Among them, QR decomposition of the channel matrix is a crucial step.
Figure 1 shows how the mathematical transformations are performed to arrive at the final expression for the computational part of the Euclidean distance metric. The Euclidean distance metric is the basis for the spherical detection process. R represents a triangular matrix for the iterative method of processing the optional symbols starting with the matrix element rM,M. Here, M represents the dimension of the channel matrix expressed in real numbers. The solution defines a traversal tree structure through M iterations, where each level i of the tree corresponds to the processed symbols of the i-th antenna.
Figure 1. Partial Euclidean distance metric equation for MIMO detection of spherical detectors
The order in which the sphere detector processes antennas has a significant impact on the BER performance. Therefore, our design uses a channel reordering technique similar to the V-BLAST technique before performing sphere detection.
There are several options for implementing tree traversal. In our implementation, we use breadth-first search because it uses the popular feed-forward structure and is hardware-friendly. At each level, we select only the K surviving nodes with the smallest distance to calculate the expansion.
The order in which the sphere detector processes antennas has a significant impact on the BER performance. Therefore, our design uses a channel reordering technique similar to the V-BLAST technique before performing sphere detection.
The method calculates the row norm of the pseudo-inverse matrix of the channel matrix through multiple iterations, and then determines the optimal column detection order of the channel matrix. Depending on the number of iterations, the method can select the row with the largest or smallest norm. The row of the inverse matrix with the smallest Euclidean norm indicates the strongest antenna influence, while the row with the largest Euclidean norm indicates the weakest antenna influence. This novel method processes the weakest data stream first, and then iterates to process the data streams from high to low power.
FPGA Hardware Applications
To implement the system, we used Xilinx Virtex®-5 FPGA technology. The design flow used Xilinx System Generator for design capture, simulation, and verification. To support a variety of antennas/users and modulation orders, the detector was designed for the most demanding 4x4, 64-QAM case.
Our model assumes that the receiver has good knowledge of the channel matrix, which can be achieved by traditional channel estimation methods. After channel reordering and QR decomposition, we start using the sphere detector. In preparation for using soft-input, soft-output channel decoders (such as turbo decoders), we generate soft outputs by computing the log-likelihood ratios (LLRs) of the detected bits.
The main architectural elements of the system include data subcarrier processing and system submodule management functions to process the required number of subcarriers in real time while minimizing processing latency. A channel matrix estimate is performed for each data subcarrier, limiting the processing time available for each channel matrix. For the selected FPGA, the target clock frequency is 225MHz, the communication bandwidth is 5MHz (equivalent to 360 data subcarriers in a WiMAX system), and the number of processing clock cycles available for each channel matrix interval is 64.
We exploit the sophisticated pipelining and time division multiplexing (TDM) capabilities of hardware functional units to achieve the real-time requirements of WiMAX OFDM symbols.
In addition to high data rates, controlling submodule latency is an important issue in guiding the architectural design process. We address the latency issue by introducing TDM of continuous channel matrices. This approach allows for longer processing time between elements of the same channel matrix while maintaining high data throughput. The number of channels that make up the TDM group varies from submodule to submodule. In the TDM scheme, 5 channels are used in the channel matrix inversion process, while 15 channels are time-division multiplexed in the real QR decomposition module. Figure 2 is a high-level flow chart of the system.
Figure 2. High-level flow chart of a MIMO 802.16e broadband wireless receiver.
Channel matrix preprocessing
The channel matrix preprocessor determines the optimal order for detecting each layer of the spatially multiplexed composite signal. The preprocessor is responsible for calculating the norms of the pseudo-inverse matrix of the channel matrix and, based on these norms, selecting the next transmission stream to be processed. The row with the smallest norm in the pseudo-inverse matrix corresponds to the strongest transmission stream (with the smallest noise amplification after detection), while the row with the largest norm corresponds to the worst quality layer (with the largest noise amplification after detection). Our implementation detects the weakest layer first and then checks each layer in order from lowest noise amplification to highest noise amplification. For each step in the sorting process, the corresponding column in the channel matrix is then cleared and the simplified matrix enters the next level of the antenna sorting processing pipeline.
Among the preprocessing algorithms, the pseudo-inverse matrix is the most computationally demanding. The core of this process is the matrix inversion, which is usually achieved by QR decomposition (QRD) with Givens rotation. Commonly used angle estimation and plane rotation algorithms (such as CORDIC) will cause severe system latency, which is unacceptable for our system. Therefore, our goal is to find an alternative solution for vector rotation and phase estimation using the embedded DSP resources of FPGAs (such as the DSP48E in Virtex-5 devices).
Previous article:Advantages of Using USB for Test and Measurement
Next article:Electromagnetic Compatibility (EMC) Design of Hybrid Integrated Circuits
Recommended ReadingLatest update time:2024-11-16 19:27
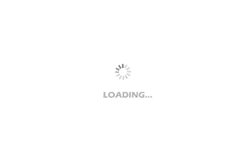
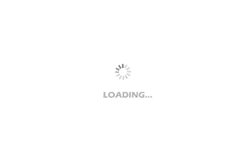
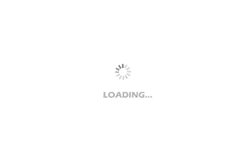
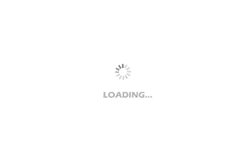
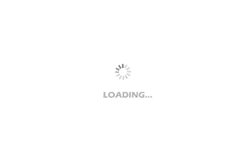
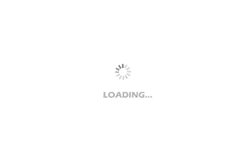
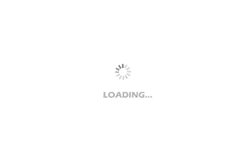
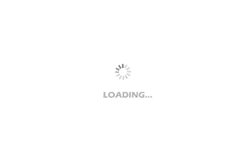
- Popular Resources
- Popular amplifiers
-
Analysis and Implementation of MAC Protocol for Wireless Sensor Networks (by Yang Zhijun, Xie Xianjie, and Ding Hongwei)
-
MATLAB and FPGA implementation of wireless communication
-
Intelligent computing systems (Chen Yunji, Li Ling, Li Wei, Guo Qi, Du Zidong)
-
Summary of non-synthesizable statements in FPGA
- High signal-to-noise ratio MEMS microphone drives artificial intelligence interaction
- Advantages of using a differential-to-single-ended RF amplifier in a transmit signal chain design
- ON Semiconductor CEO Appears at Munich Electronica Show and Launches Treo Platform
- ON Semiconductor Launches Industry-Leading Analog and Mixed-Signal Platform
- Analog Devices ADAQ7767-1 μModule DAQ Solution for Rapid Development of Precision Data Acquisition Systems Now Available at Mouser
- Domestic high-precision, high-speed ADC chips are on the rise
- Microcontrollers that combine Hi-Fi, intelligence and USB multi-channel features – ushering in a new era of digital audio
- Using capacitive PGA, Naxin Micro launches high-precision multi-channel 24/16-bit Δ-Σ ADC
- Fully Differential Amplifier Provides High Voltage, Low Noise Signals for Precision Data Acquisition Signal Chain
- Innolux's intelligent steer-by-wire solution makes cars smarter and safer
- 8051 MCU - Parity Check
- How to efficiently balance the sensitivity of tactile sensing interfaces
- What should I do if the servo motor shakes? What causes the servo motor to shake quickly?
- 【Brushless Motor】Analysis of three-phase BLDC motor and sharing of two popular development boards
- Midea Industrial Technology's subsidiaries Clou Electronics and Hekang New Energy jointly appeared at the Munich Battery Energy Storage Exhibition and Solar Energy Exhibition
- Guoxin Sichen | Application of ferroelectric memory PB85RS2MC in power battery management, with a capacity of 2M
- Analysis of common faults of frequency converter
- In a head-on competition with Qualcomm, what kind of cockpit products has Intel come up with?
- Dalian Rongke's all-vanadium liquid flow battery energy storage equipment industrialization project has entered the sprint stage before production
- Allegro MicroSystems Introduces Advanced Magnetic and Inductive Position Sensing Solutions at Electronica 2024
- Car key in the left hand, liveness detection radar in the right hand, UWB is imperative for cars!
- After a decade of rapid development, domestic CIS has entered the market
- Aegis Dagger Battery + Thor EM-i Super Hybrid, Geely New Energy has thrown out two "king bombs"
- A brief discussion on functional safety - fault, error, and failure
- In the smart car 2.0 cycle, these core industry chains are facing major opportunities!
- The United States and Japan are developing new batteries. CATL faces challenges? How should China's new energy battery industry respond?
- Murata launches high-precision 6-axis inertial sensor for automobiles
- Ford patents pre-charge alarm to help save costs and respond to emergencies
- New real-time microcontroller system from Texas Instruments enables smarter processing in automotive and industrial applications
- What are the advantages of machine vision defect detection systems?
- Happy New Year to everyone!
- How can a car's keyless entry system be hacked?
- What is a battery monitoring system (BMS)? What are its main functions? What is the BMS architecture? This article explains
- I want to learn quantum mechanics by myself. What kind of mathematics books should I read first? Thank you.
- What books are there on the desk for reading? [Show it]
- Actual development and experience of the second phase
- Problems with using modelsim
- Teach you step by step to learn 51 single chip microcomputer tutorial from scratch
- Quick test! Accurate interpretation! Kickstart software platform now open for free trial for 60 days!