By: Dr. Gunar Lorenz Senior Director, Technical Marketing, Infineon Technologies
Proofreader: Ding Yue Chief Engineer of Consumer, Computing and Communications Business in Greater China, Infineon Technologies
Introduction
At Infineon, we have always believed that excellent audio solutions are essential to enhance the user experience of consumer devices. We are proud of our unwavering commitment to innovation and the significant advances we have made in active noise cancellation, voice transmission, studio recording, audio zoom and other related technologies. As a leading supplier of MEMS microphones, Infineon focuses its resources on improving the audio quality of MEMS microphones to bring excellent experience to a variety of consumer devices such as TWS and over-ear headphones, laptops, tablets, conferencing systems, smartphones, smart speakers, hearing aids and even cars.
Today, we live in an exciting time where AI is revolutionizing daily life and tools like ChatGPT are redefining productivity through intuitive text and voice interactions. As AI systems continue to advance, traditional business models, beliefs, and assumptions are being challenged. What role does voice play in the emerging AI ecosystem? As business leaders, do we need to rethink our beliefs? Will the rise of generative AI reduce the importance of high-quality voice input, or will high-quality voice input become a necessity for widespread adoption of AI services and personal assistants?
Artificial Intelligence: From Right-Hand Assistant to Best Friend
It’s natural for humans to tailor their responses not only to the content of a question, but also to the format in which it’s asked. The human voice provides a variety of clues that can be used to determine the age, gender, social and cultural background, and emotional state of the person asking the question. Additionally, recognizing the context (e.g., an airport, an office, in traffic, or a physical activity like running) can help determine the questioner’s intent and tailor answers accordingly, leading to a better conversation.
Despite the great advances in AI capabilities, it is still believed that AI-based assistance tools lack the ability to correctly predict the human intention of asking a question or how a specific message will be interpreted. To improve human-computer interaction, AI should consider three key factors when making rhetorical choices: understanding of the listener, the listener's emotional state, and the environmental context.
In many cases, the audio signal received alone is sufficient to extract useful information and respond appropriately. For example, consider a phone call or audio conference with someone you have never met. More importantly, consider how one's perception of another person develops and changes after repeated conversations without the opportunity to communicate in person.
Recent research has shown that even small changes in an AI’s verbal response style can lead to noticeable changes in the AI’s social abilities and personality. It is reasonable to assume that, given the right level of vocal input, future AI systems will be able to function as effective companions, exhibiting the behaviors of a human friend, such as asking questions and really listening to the answers, or simply listening and reserving judgment when appropriate.
How do humans experience audio signals?
Like any verbal communication, audio messages use words and text to convey thoughts, emotions, and ideas. In addition, other elements of communication such as pitch, speed, volume, and background noise can affect the overall perception of the message.
From a scientific point of view, the human ear perceives audio signals based on two key factors: frequency and sound pressure level. Sound pressure level (SPL) is measured in decibels (dBSPL) and represents the amplitude of sound pressure oscillating around the ambient atmospheric pressure. A sound pressure level of 100dBSPL is equivalent to the loud noise of a lawn mower or a helicopter. The lowest point in the sound pressure level range (0dB) is equivalent to a sound pressure oscillation of 20µPa, which represents the hearing threshold of a healthy young person with optimal hearing at a frequency of 1kHz. All human sounds related to speech fall into the frequency band of 100Hz to 8kHz. The corresponding human hearing thresholds according to the ISO 226:2023 standard are shown in Figure 1.
Figure 1: Hearing threshold: Sound level at which a person makes 50% correct detection responses in repeated trials according to ISO 226:2023
As shown in Figure 1, the human ear is particularly sensitive to frequencies in the range of 500Hz to 6kHz. Any frequency balance issues at these frequencies will have a significant impact on the perceived quality of sounds and instruments. Frequencies between 500Hz and 4kHz contain most of the information in human speech that affects speech intelligibility. Specifically, frequencies around 2 kHz are particularly important. Frequencies between 5kHz and 10kHz are very important for music. These frequencies add "liveliness" and "brightness" to the sound. However, these frequencies contain relatively little speech information, only sibilance, which is the hissing sound at the beginning of words such as "zhi", "chi" and "shi". Reducing the sibilance around 6-8kHz will have an adverse effect on speech intelligibility.
Most of us know that the human hearing threshold decreases with age, as shown in Figure 2.
Figure 2: This graph shows the hearing threshold loss of normal males at different ages under mono headphone listening conditions. Note that there is a similar graph for females, where the hearing loss decreases slightly with age (ISO7029:2017)
It is important to note that even mild hearing loss (which occurs in most people between the ages of 40 and 50) can have a significant impact on an individual's life. For example, someone with mild hearing loss may have trouble following group conversations in a noisy environment. In addition, they may miss important auditory cues such as warning signs or alarms.
Is current audio hardware sufficient for the needs of future AI?
Now that we have a better understanding of how humans perceive audio signals, let’s revisit our original question of what quality of audio input current and future AI will need to perform at a level indistinguishable from that of humans.
Most consumer devices on the market today use MEMS microphones to record audio signals. MEMS microphones are the primary audio capture technology for AI personal assistants, and devices using AI assistant technology are now available on the market.
The recording quality of a MEMS microphone depends on its dynamic range. The upper limit of the dynamic range is determined by the acoustic overload point (AOP), which defines the distortion performance of the microphone at high sound pressure levels. The self-noise of the microphone determines the lower limit of its dynamic range. The method to measure the self-noise of a microphone is the signal-to-noise ratio (SNR), which defines the ratio between the self-noise of the microphone and the signal it captures (sensitivity). However, for our discussion, the signal-to-noise ratio is somewhat inappropriate because the self-noise of the signal-to-noise ratio uses A-weighting, which is actually defined based on the human ability to perceive audio signals.
If the intended recipient of the audio signal is an artificial intelligence, the equivalent noise level (ENL) of the associated microphone is a more appropriate parameter to measure performance, as it ignores the human perception factor of the recorded sound. The equivalent noise level (ENL) refers to the signal produced by the microphone in the absence of an external sound source. The equivalent noise level (ENL) is measured in decibels (dBSPL), which represents the sound pressure level of the same voltage as the microphone's self-noise.
It is worth noting that any sound information below the equivalent noise level ENL is essentially lost and cannot be recovered, regardless of the sound processing method used later. Therefore, if there are no other components in the audio chain that introduce noise before the signal reaches the AI algorithm, the microphone ENL can be regarded as the hearing threshold of the AI algorithm. It should be noted that this is a highly simplified assumption, as there are usually many other components in the audio chain, including the sound channel, waterproof protective membrane, and audio processing chain.
Please refer to Figure 3 for a direct comparison of the ENL curves of two MEMS microphones and the human hearing threshold.
Figure 3: Comparison of 1/3 octave equivalent noise level ENL and typical male hearing threshold for mid-range and high-end MEMS microphones
The red line is the equivalent noise level ENL curve of a microphone with a signal-to-noise ratio of 65dB(A), and the microphone has an integrated dust-proof design. The corresponding MEMS microphone is currently used in many high-end smartphones produced by multiple suppliers.
The purple line below shows the ENL curve for Infineon's latest high-end digital microphone, which features an innovative protective design to provide dust and water resistance. This microphone represents the current state of the art and was only released in high-end tablets this year. We expect that microphones with comparable performance will appear in high-end smartphones by the end of this year. It is worth noting that reducing the self-noise of a microphone by 5-10dB is a significant achievement, especially considering that sound pressure is expressed using a logarithmic scale.
While Infineon has made significant progress in reducing the self-noise of high-end MEMS microphones, there is still a large gap in the ability of microphones to discern low sound pressure levels compared to the human ear. This is especially true around 2kHz, which is critical to ensure a high level of sound intelligibility for human listeners. The gap between the hearing ability of a young person and Infineon’s most advanced microphones is more than 12dBSPL. Compared to the microphones used in current high-end mobile phones, the gap is significantly larger, at 17dBSPL. It is important to point out again that this assessment only considers the self-noise of the MEMS microphone and does not take into account additional noise sources in the audio chain that would further degrade the overall performance.
Previous article:Microcontrollers that combine Hi-Fi, intelligence and USB multi-channel features – ushering in a new era of digital audio
Next article:ROHM develops the second generation of MUS-IC™ series audio DAC chips suitable for high-resolution audio playback
Recommended ReadingLatest update time:2024-11-21 17:52
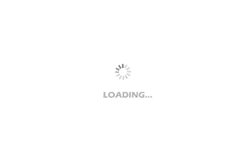
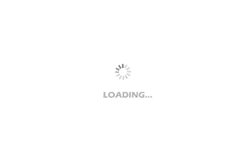
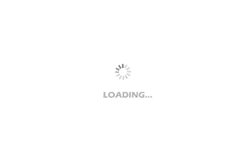
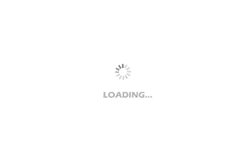
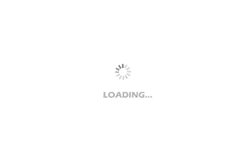
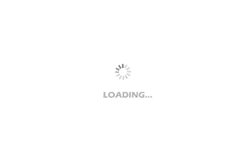
- ROHM develops the second generation of MUS-IC™ series audio DAC chips suitable for high-resolution audio playback
- ADALM2000 Experiment: Transformer-Coupled Amplifier
- High signal-to-noise ratio MEMS microphone drives artificial intelligence interaction
- Advantages of using a differential-to-single-ended RF amplifier in a transmit signal chain design
- ON Semiconductor CEO Appears at Munich Electronica Show and Launches Treo Platform
- ON Semiconductor Launches Industry-Leading Analog and Mixed-Signal Platform
- Analog Devices ADAQ7767-1 μModule DAQ Solution for Rapid Development of Precision Data Acquisition Systems Now Available at Mouser
- Domestic high-precision, high-speed ADC chips are on the rise
- Microcontrollers that combine Hi-Fi, intelligence and USB multi-channel features – ushering in a new era of digital audio
- Intel promotes AI with multi-dimensional efforts in technology, application, and ecology
- ChinaJoy Qualcomm Snapdragon Theme Pavilion takes you to experience the new changes in digital entertainment in the 5G era
- Infineon's latest generation IGBT technology platform enables precise control of speed and position
- Two test methods for LED lighting life
- Don't Let Lightning Induced Surges Scare You
- Application of brushless motor controller ML4425/4426
- Easy identification of LED power supply quality
- World's first integrated photovoltaic solar system completed in Israel
- Sliding window mean filter for avr microcontroller AD conversion
- What does call mean in the detailed explanation of ABB robot programming instructions?
- Shenzhen Institute of Advanced Technology and BYD will cooperate in the development of solid-state batteries
- Honda's all-solid-state battery demonstration production line is unveiled for the first time and will be put into use in January 2025
- WPG Group launches automotive ambient lighting solution based on Fudan Microelectronics and ams OSRAM products
- WPG Group launches automotive ambient lighting solution based on Fudan Microelectronics and ams OSRAM products
- Why is the vehicle operating system (Vehicle OS) becoming more and more important?
- Car Sensors - A detailed explanation of LiDAR
- Simple differences between automotive (ultrasonic, millimeter wave, laser) radars
- Comprehensive knowledge about automobile circuits
- Bourns Launches Two Thick Film Resistor Series with High Power Dissipation Capabilities in Compact TO-220 and DPAK Package Designs
- Bourns Launches Two Thick Film Resistor Series with High Power Dissipation Capabilities in Compact TO-220 and DPAK Package Designs
- Allegro board layer color configuration
- Detailed explanation of series/parallel resonant circuit
- RISC-V MCU IDE MRS (MounRiver Studio) development: Print FLASH and RAM usage information after compilation
- Zhuge Liang's true military command ability
- Newbie, please help! Urgent! Urgent!!!
- EEWORLD University - Solar System Design Made Simple
- Resistance matching problem when transistor is used as switch!
- EEWORLD University ---- Medical monitoring and wearable devices
- Photodetector Array Signal Processing
- Can cam350 export individual component packages based on gerber files?