The model training task was shortened to two weeks, and the computing power and scalability were doubled.
Picture from JD.com
With the help of NVIDIA DGX SuperPOD, JD Research Institute trained a Vega-MT model with nearly 5 billion parameters, which shined at the 17th International Machine Translation Competition (WMT) in 2022. Vega-MT is available in Chinese-English (BLEU 33.5, chrF 0.611), English-Chinese (BLEU 49.7, chrF 0.446), German-English (BLEU 33.7, chrF 0.585), English-German (BLEU 37.8, chrF 0.643), Czech - English (BLEU 54.9, chrF 0.744), English-Czech (BLEU 41.4, chrF 0.651) and English-Russian (BLEU 32.7, chrF 0.584) won the championship in seven translation tracks.
As a large-scale AI infrastructure, NVIDIA DGX SuperPOD has a complete and advanced infrastructure. Compared with the original V100 cluster, DGX SuperPOD has not only achieved nearly twice the improvement in single-card computing power, but also has linear growth in computing power scalability, that is, DGX SuperPOD has also achieved twice the scalability compared to before. improvement. In the case of multiple nodes, a total improvement of about 4 times was obtained. Therefore, the training task that originally took several months to complete a similar model (of considerable size and complexity) was shortened to two weeks, and researchers also had more time to optimize the model.
Customer profile and application background
JD.com is a technology and service company based on the supply chain. JD Explore Academy adheres to the group mission of "being technology-based and committed to a more efficient and sustainable world". It is based on the technological development of each business group and business unit of JD Group and brings together the entire group. resources and capabilities, the established R&D department focusing on cutting-edge technology exploration is an ecological platform to achieve research and collaborative innovation. The Discovery Institute is deeply engaged in three major fields of pan-artificial intelligence, including "quantum machine learning", "trustworthy artificial intelligence" and "super deep learning", achieving disruptive innovation from the basic theoretical level to assist the development of digital intelligence industry and social change. Use original technology to empower JD Group’s entire industry chain scenarios such as retail, logistics, health, and technology, create a source of technological highlands, achieve leap-forward development from quantitative change to qualitative change, and lead the industry forward.
The International Machine Translation Competition (WMT) is recognized by the global academic community as the top international machine translation competition. It is organized by the International Association for Computational Linguistics (ACL) and is the top competition under the Association. Since 2006 to the present, every WMT competition has been a platform for major universities, technology companies and academic institutions around the world to showcase their machine translation capabilities, and has witnessed the continuous progress of machine translation technology.
This major achievement of JD Research Institute in the WMT competition further validates the superiority of large natural language processing models in understanding, generation, and cross-language modeling.
Customer Challenges
Machine translation faces many challenges: several common languages are widely used and rich in data resources, small languages are very necessary in cross-border e-commerce but the data is insufficient, and training of small data sets faces challenges; at the same time, it is also difficult to mine the relationship between languages One is because the complexity and ambiguity of language generation, the diversity of expressions, cultural backgrounds, and differences between languages are all unavoidable problems in machine translation competitions.
From the 110 million parameters of GPT-1 in 2018 to today's large-scale language models with trillions of parameters, the significant improvement in the accuracy of large models on multiple language tasks helps us build a richer understanding of natural language. smart system.
Vega-MT uses many advanced technologies, including multidirectional pre-training, Extremely Large Transformer, cycle translation and bidirectional self-training, to fully tap bilingualism Data, knowledge of monolingual data. In addition, strategies such as noise channel reordering and generalization fine-tuning are also used to enhance the robustness of the Vega-MT system and the level of trustworthiness of the translation.
However, we still face many difficulties when training large models. Previously, a single GPU was sufficient for model training for general tasks, but in large model scenarios, multi-node collaboration is required to complete the final training task, which also poses new challenges to existing GPU computing clusters. Take the well-known GPT-3 as an example. It uses 45 TB of training data and reaches a maximum of 175 billion model parameters. When using mixed precision, it occupies a total of about 2.8 TB of video memory and requires more than 35 GPUs to convert the model. Let it all go.
Therefore, the training challenges focus on single-card computing power and multi-card multi-node communication, and training will also span multiple nodes. At this time, aspects such as data transmission, task scheduling, parallel optimization, and resource utilization are particularly important.
application solution
When building an AI infrastructure, we will face challenges from all aspects, such as computing resources, networks, storage, and even the top-level software used for task scheduling. However, these aspects are not independent and need to be considered comprehensively.
The NVIDIA DGX SuperPOD used by JD Discovery Research Institute is a comprehensive and complete high-performance solution. SuperPOD AI cluster is based on DGX server, HDR InfiniBand 200G network card and NVIDIA Quantum QM8790 switch. The computing network and storage network are isolated, which not only ensures optimal computing power, but also ensures efficient interconnection between nodes and cards, maximizing distribution. efficiency of training.
In terms of computing power, the computing power of a single node is as high as 2.4 PFLOPS. Using a single node for training, BERT only takes 17 minutes to complete training, Mask R-CNN only takes 38 minutes, and RetinaNet only takes 83 minutes. For Transformer XL Base, training can be completed in 181 minutes. At the same time, relying on Multi-Instance GPU (MIG) technology, the GPU can be divided into multiple instances. Each instance has its own independent video memory, cache and streaming multi-processor, and fault isolation between each other. This can further improve GPU utilization and meet tasks requiring different computing power.
At the network level, through Scalable Hierarchical Aggregation and Reduction Protocol (SHARP) technology, aggregation computing can be migrated from the CPU to the switch network, eliminating the need to send data multiple times between nodes, greatly reducing the network traffic reaching the aggregation node, thereby significantly It reduces the time to execute MPI and at the same time makes the communication efficiency no longer directly related to the number of nodes, further ensuring the scalability of computing power. In addition, it frees the CPU from the task of processing communications, allowing valuable CPU resources to focus on computing, further improving the overall cluster processing capability.
At the storage level, when training a model, it is often necessary to read the training data from the storage multiple times, and the time-consuming reading operation will also affect the timeliness of the training to a certain extent. DGX SuperPOD uses a high-performance multi-tier storage architecture to balance performance, capacity and cost requirements. With the help of GPU Direct RDMA technology, you can bypass the CPU and directly connect to the GPU, storage and network devices for high-speed and low-latency data transmission.
At the software level, in order to build a cluster and ensure the persistence and smooth operation of the cluster, upper-layer monitoring and scheduling management software is indispensable. Base Command Manager is a cluster management system that can perform a series of configurations on the cluster, manage user access, resource monitoring, log recording, and job task scheduling through slurm. At the same time, NGC covers a large number of AI, HPC, and data science-related resources. Users can easily obtain powerful software, container images, and various pre-trained models.
At the same time, the Discovery Institute team monitors and manages the cluster 24/7 to ensure the smooth operation of training tasks for a long time. Monitoring resource utilization also ensures that the computing resources on each node are fully utilized. Under the complete scheduling and monitoring work and the high reliability quality assurance of DGX SuperPOD, all the training nodes used did not have any problems within 20 days of model training (2 weeks of pre-training + 5 days of fine-tuning). The training was finally completed successfully.
Use effect and impact
Vega-MT was successfully used in the Omni-Force AIGC applet released by JD.com during the National Day. The application of the mini program is that users input text to generate corresponding pictures. With the support of Vega-MT, the mini program can support text input in multiple languages, such as Chinese, English, Spanish, etc.
JD Discovery Research Institute said: “With the support of NVIDIA DGX SuperPOD, JD Discovery Research Institute can quickly iterate models and help high-accuracy models to be implemented quickly, further improving user experience, reducing costs, and improving effects and business benefits. This time NVIDIA DGX SuperPOD supports us in winning the WMT competition, which not only increases the company's visibility, but also helps JD.com become a more trusted brand by users."
Previous article:Infineon Technologies Unveils TRAVEO™ T2G-C Microcontroller Family and Altia CloudWare™ Software Platform at CES 2023
Next article:FG25 sub-GHz SoC with long transmission distance, large memory and high security is now generally available
Recommended ReadingLatest update time:2024-11-15 12:04
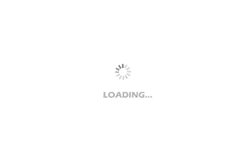
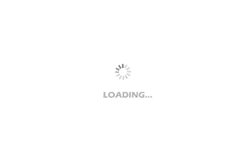
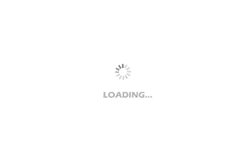
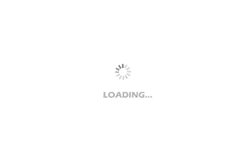
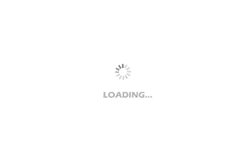
- Popular Resources
- Popular amplifiers
-
Virtualization Technology Practice Guide - High-efficiency and low-cost solutions for small and medium-sized enterprises (Wang Chunhai)
-
Semantic Segmentation for Autonomous Driving: Model Evaluation, Dataset Generation, Viewpoint Comparison, and Real-time Performance
-
Design and application of autonomous driving system (Yu Guizhen, Zhou Bin, Wang Yang, Zhou Yiwei)
-
ASPEN: High-throughput LoRA fine-tuning of large language models using a single GPU
- Molex leverages SAP solutions to drive smart supply chain collaboration
- Pickering Launches New Future-Proof PXIe Single-Slot Controller for High-Performance Test and Measurement Applications
- CGD and Qorvo to jointly revolutionize motor control solutions
- Advanced gameplay, Harting takes your PCB board connection to a new level!
- Nidec Intelligent Motion is the first to launch an electric clutch ECU for two-wheeled vehicles
- Bosch and Tsinghua University renew cooperation agreement on artificial intelligence research to jointly promote the development of artificial intelligence in the industrial field
- GigaDevice unveils new MCU products, deeply unlocking industrial application scenarios with diversified products and solutions
- Advantech: Investing in Edge AI Innovation to Drive an Intelligent Future
- CGD and QORVO will revolutionize motor control solutions
- LED chemical incompatibility test to see which chemicals LEDs can be used with
- Application of ARM9 hardware coprocessor on WinCE embedded motherboard
- What are the key points for selecting rotor flowmeter?
- LM317 high power charger circuit
- A brief analysis of Embest's application and development of embedded medical devices
- Single-phase RC protection circuit
- stm32 PVD programmable voltage monitor
- Introduction and measurement of edge trigger and level trigger of 51 single chip microcomputer
- Improved design of Linux system software shell protection technology
- What to do if the ABB robot protection device stops
- Microchip Accelerates Real-Time Edge AI Deployment with NVIDIA Holoscan Platform
- Microchip Accelerates Real-Time Edge AI Deployment with NVIDIA Holoscan Platform
- Melexis launches ultra-low power automotive contactless micro-power switch chip
- Melexis launches ultra-low power automotive contactless micro-power switch chip
- Molex leverages SAP solutions to drive smart supply chain collaboration
- Pickering Launches New Future-Proof PXIe Single-Slot Controller for High-Performance Test and Measurement Applications
- Apple faces class action lawsuit from 40 million UK iCloud users, faces $27.6 billion in claims
- Apple faces class action lawsuit from 40 million UK iCloud users, faces $27.6 billion in claims
- The US asked TSMC to restrict the export of high-end chips, and the Ministry of Commerce responded
- The US asked TSMC to restrict the export of high-end chips, and the Ministry of Commerce responded
- Share: A summary of the most comprehensive answers to various questions in the 2019 e-sports competition
- Live broadcast at 10:30 am today | A brief discussion on Microchip's FPGA products and intelligent embedded vision solutions
- Wi-Fi&BLE SoC NANO main control board (WBRU) development board XANWE has not been evaluated yet 01--Photography Appreciation
- Prize-giving event | Visit the Avnet Artificial Intelligence Cloud Exhibition, unlock AI information, and win prizes!
- [Qinheng RISC-V core CH582] 6 BLE_UART routine evaluation
- Protection circuit design issues
- Design and implementation of fast-plug low intermodulation RF connector
- AM5708 DSP-side waveform simulation
- Servo controller
- Design of linear array CCD driving timing circuit based on FPGA