introduction
The traditional camera vision technology is relatively easy to implement in terms of algorithms, so it has been used by most existing car manufacturers for assisted driving functions. However, with the development of autonomous driving technology, algorithms based on deep learning have begun to emerge. In this issue, the editor will talk about the relevant technical information of deep vision algorithms. Let us learn together.
01. Overview of Deep Learning
Deep learning (DL) is a general term for a class of pattern analysis methods and is a new research direction in the field of machine learning (ML). By learning the inherent laws and representation levels of sample data, deep learning enables machines to have the ability to analyze and learn like humans, and can recognize data such as text, images and sounds, thereby realizing artificial intelligence (AI).
Figure: (artificial intelligence, machine learning, deep learning) relationship diagram
02. Significance of Deep Learning
Many of you may know that for a car to achieve autonomous driving, the three major systems of perception, decision-making, and control are indispensable. Among them, perception is placed first by us, because the vehicle first needs to understand the relationship between the vehicle and the three-dimensional changes in the real world in real time, that is, to accurately understand the position relationship and changes between the vehicle and the surrounding people, vehicles, obstacles, and road elements. Deep learning algorithms have effectively improved the "intelligence" level of sensors such as cameras and lidars, which to a large extent determines the reliability of autonomous vehicles in complex road conditions. Therefore, the application of deep learning has become the key. In addition, although there are many types of perception sensors for cars, the camera is the only sensor that can perceive the real world through images. Through deep learning, the ability to recognize images can be quickly improved, making our driving safer.
03. Differences between traditional camera vision algorithms and deep learning algorithms
Friends who have read the editor’s previous article about traditional camera vision algorithms will ask, since traditional camera vision algorithms can be used, why do we still need to study deep learning algorithms?
Because traditional vision algorithms have their own bottlenecks, whether it is a monocular camera or a multi-camera camera, traditional vision algorithms are based on artificial feature extraction to obtain sample feature libraries for identification and calculation. When the autonomous vehicle is driving, if it is found that the feature library does not have the sample or the feature library sample is inaccurate, the traditional vision algorithm will not be able to recognize it. In addition, the traditional vision algorithm also has poor segmentation in complex scenes. Therefore, the traditional vision algorithm based on artificial feature extraction has performance bottlenecks and cannot fully meet the target detection of autonomous driving.
Image source: Deep Learning vs. Traditional Computer Vision
The feature extraction advantage of the camera's deep learning vision algorithm is that it is based on a neural network algorithm, which simulates the human neural network and can perform semantic segmentation on information such as images input by the camera in autonomous driving (even the point cloud of lidar), effectively solving the problem of traditional vision algorithms having poor segmentation of complex actual scenes or sample feature libraries, allowing higher accuracy in tasks such as image classification, semantic segmentation, target detection, and simultaneous localization and mapping (SLAM).
Next, to make it easier for everyone to understand, I will first talk about what deep learning neural networks are, how they help cameras complete visual calculations such as image recognition, and how they are better than traditional camera visual algorithms.
04. Deep Learning Neural Network
It is easy to find that deep learning is accomplished by "depth" + "learning" from the literal meaning. "Depth" is to imitate the mode of information transmission and processing between neurons in the brain. Its model structure includes input layer, hidden layer and output layer. The input layer and output layer generally have only one layer, while the hidden layer (or middle layer) often has 5, 6 or even more layers. Multiple hidden layer (middle layer) nodes are called "depth" in deep learning; "learning" is to carry out "feature learning" or "representation learning", that is, through layer-by-layer feature transformation, the feature representation of the sample in the original space is transformed into a new feature space, and big data is used for learning and tuning, to establish an appropriate amount of neuron computing nodes and a multi-layer operation hierarchy, to get as close as possible to the actual correlation relationship, so as to make feature classification or prediction easier.
Figure: Schematic diagram of neural network structure
The above content is too abstract. In simple terms, the neural network has three layers:
Input: Each neuron in the input layer corresponds to a variable feature, and the neurons in the input layer are equivalent to containers containing numbers.
Output: Output layer, one neuron for regression problems and multiple neurons for classification problems
Parameters: All parameters in the network, that is, the weights and biases of the neurons in the middle layer (or hidden layer). Each neuron represents the features learned by the neural network in that layer.
Here you just need to remember that no matter the size of the neural network, it is composed of stacked single neuron networks.
It doesn’t matter if it’s hard to understand. Let me give you an example to explain it.
Suppose we want to buy a house, then the final transaction price we can afford is the output layer;
The input layer may have many raw features (i.e., factors for buying a house, such as house size, number of rooms, number of nearby schools, school education quality, public transportation, and parking spaces);
The neurons in the middle layer (or hidden layer) are the features we can learn, such as family size, education quality, travel
The more input feature data we collect, the more sophisticated the neural network will be. And as the number of original feature neurons in the input layer increases, the middle layer can learn enough and more detailed combination features with different meanings from the original features. For example, the area of the house and the number of rooms can indicate the number of family members, and the number of schools and the quality of the schools indicate the quality of education. Through the classification, statistics and calculation of the features corresponding to each neuron, we finally get the "housing price" we want in the output layer.
For the deep learning of the camera, the input layer is the image obtained by the camera. The image can be regarded as a bunch of data streams for the camera deep learning algorithm. These data streams can also be divided into more original features, such as the sparseness and density of each pixel in the image, semantic and geometric information, as well as color, brightness, grayscale, etc.; the middle layer classifies and calculates the original feature information of the input layer, and can identify the objects contained in the image (such as lane lines, obstacles, people, cars, traffic lights, etc.), and finally outputs the real-time distance, size, shape, traffic light color and other elements of objects related to the autonomous driving vehicle, helping the autonomous driving vehicle to complete real-time perception of the surrounding environment, recognition, ranging and other functions.
Pictured: NavInfo - Camera Vision Recognition Sample
Pictured: NavInfo - underground garage mapping and real-time relocation system
From the above, we can see that the deep learning algorithm for camera vision based on neural networks is much more useful than the traditional camera vision algorithm based on artificial feature extraction. Therefore, the current mainstream camera vision algorithms will use deep learning to solve the accuracy, recognition rate and image processing speed of self-driving cars for image classification, image segmentation, object detection, multi-target tracking, semantic segmentation, drivable area, target detection and simultaneous localization and mapping (SLAM), scene analysis and other tasks. Deep learning vision algorithms also make it possible for self-driving cars to be mass-produced quickly.
05. Camera Deep Learning Algorithm
There are three common deep learning vision algorithms used in autonomous driving camera sensors:
(1) A neural network system based on convolution operations, namely a convolutional neural network (CNN). It is widely used in image recognition.
(2) Autoencoding neural networks based on multi-layer neurons, including autoencoders and sparse coding, which has received widespread attention in recent years.
(3) Pre-training is performed in the form of a multi-layer autoencoder neural network, and then the deep belief network (DBN) weights are further optimized by combining the identification information.
Figure: General process of deep learning
06. Deep learning is a black box
Although we have talked so much about how deep learning algorithms based on neural networks obtain input and output, in fact, the above cases and algorithm classifications only help us to simply understand the neural network of deep learning. In fact, deep learning is a "black box". "Black box" means that the intermediate process of deep learning is unknown, and the results of deep learning are uncontrollable. In fact, programmers do not know how the neural network they program learns. They only know that the final output result is to use the "Universal approximation theorem" to fit the relationship between input data and output results as accurately as possible.
Previous article:SAIC's self-developed fuel cell system technology performance is comparable to the world's leading level
Next article:Which electric car company is the best? You will understand after understanding the solutions of these semiconductor manufacturers
Recommended ReadingLatest update time:2024-11-15 08:02
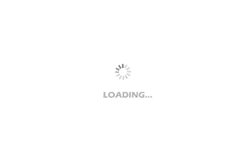
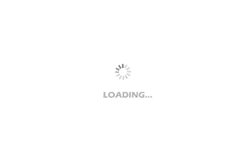
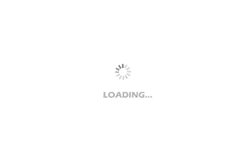
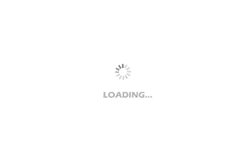
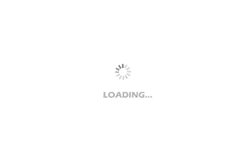
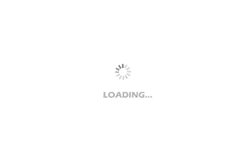
- Popular Resources
- Popular amplifiers
-
LiDAR point cloud tracking method based on 3D sparse convolutional structure and spatial...
-
GenMM - Geometrically and temporally consistent multimodal data generation from video and LiDAR
-
Comparative Study on 3D Object Detection Frameworks Based on LiDAR Data and Sensor Fusion Technology
-
A review of deep learning applications in traffic safety analysis
- Red Hat announces definitive agreement to acquire Neural Magic
- 5G network speed is faster than 4G, but the perception is poor! Wu Hequan: 6G standard formulation should focus on user needs
- SEMI report: Global silicon wafer shipments increased by 6% in the third quarter of 2024
- OpenAI calls for a "North American Artificial Intelligence Alliance" to compete with China
- OpenAI is rumored to be launching a new intelligent body that can automatically perform tasks for users
- Arm: Focusing on efficient computing platforms, we work together to build a sustainable future
- AMD to cut 4% of its workforce to gain a stronger position in artificial intelligence chips
- NEC receives new supercomputer orders: Intel CPU + AMD accelerator + Nvidia switch
- RW61X: Wi-Fi 6 tri-band device in a secure i.MX RT MCU
Professor at Beihang University, dedicated to promoting microcontrollers and embedded systems for over 20 years.
- LED chemical incompatibility test to see which chemicals LEDs can be used with
- Application of ARM9 hardware coprocessor on WinCE embedded motherboard
- What are the key points for selecting rotor flowmeter?
- LM317 high power charger circuit
- A brief analysis of Embest's application and development of embedded medical devices
- Single-phase RC protection circuit
- stm32 PVD programmable voltage monitor
- Introduction and measurement of edge trigger and level trigger of 51 single chip microcomputer
- Improved design of Linux system software shell protection technology
- What to do if the ABB robot protection device stops
- CGD and Qorvo to jointly revolutionize motor control solutions
- CGD and Qorvo to jointly revolutionize motor control solutions
- Keysight Technologies FieldFox handheld analyzer with VDI spread spectrum module to achieve millimeter wave analysis function
- Infineon's PASCO2V15 XENSIV PAS CO2 5V Sensor Now Available at Mouser for Accurate CO2 Level Measurement
- Advanced gameplay, Harting takes your PCB board connection to a new level!
- Advanced gameplay, Harting takes your PCB board connection to a new level!
- A new chapter in Great Wall Motors R&D: solid-state battery technology leads the future
- Naxin Micro provides full-scenario GaN driver IC solutions
- Interpreting Huawei’s new solid-state battery patent, will it challenge CATL in 2030?
- Are pure electric/plug-in hybrid vehicles going crazy? A Chinese company has launched the world's first -40℃ dischargeable hybrid battery that is not afraid of cold
- Has anyone tried the baud rate adaptation of LIN communication?
- Analog Electronics Design
- Will adding a nonlinear link to the RC bridge sine wave oscillation circuit cause waveform distortion?
- Introduction to TI_DSP link command file (*.cmd)
- [N32L43x Review] 2. Turn on the lights, blink, blink, blink...
- EEWORLD University Hall - Talking about the development creativity of electronic products and using network resources to help development
- The output of the op amp in the active integrator circuit oscillates. What should I do?
- Developing Minitel Applications with ESP32 and MicroPython
- This circuit can adjust the voltage under no-load condition, but the voltage is only about 2V after the load is installed.
- Tmall Murata official flagship store, spend 1000-200, get a gift! What do you want to buy this Double 11?