The automatic focusing method based on image technology is completely different from the traditional automatic focusing technology. It directly uses image processing technology on the captured image, analyzes the image quality, obtains the current focus state of the system, and then adjusts the focal length of the imaging system lens through the driving mechanism to realize the automatic focusing process.
1 Focusing Algorithm Analysis
Whether an image is focused is reflected in the spatial domain by whether the edges and details of the image are clear, and the edge and detail information of the image can be obtained by differentiating the image. Therefore, the information is used as the criterion for focusing. This function that extracts the edge information of the image is called the focus value function, and the value obtained after the image is processed by it can reflect the clarity of the image.
The focusing*valence function should have the following characteristics: unbiasedness, unimodality, high sensitivity, high signal-to-noise ratio, and small amount of calculation.
Therefore, the key to achieve self-focusing by image processing is to find an ideal basis for image clarity value, so the core algorithm of this system is the image clarity value function implementation algorithm and the focusing implementation algorithm. In the image clarity algorithm, the image is mainly processed in three parts: image preprocessing, clarity value algorithm, and motor control algorithm.
Converting images from the spatial domain to the frequency domain for analysis is a common method of image processing. At the same time, since a clear image contains more image information and details than a blurred image, after analysis, it is found that the edge information of an image with higher clarity is clearly discernible, which corresponds to the high-frequency component of the image after Fourier transform is enhanced and the low-frequency component is reduced, while the blurred image has an increase in low-frequency component and a decrease in high-frequency component. In this way, the theoretical basis of the image clarity * valence function based on the power spectrum is generated.
For a continuous image f(x, y), its two-dimensional Fourier transform can be calculated when
For digital images, if we consider sampling f(x, y) in the x and y directions with sampling intervals △x, △y, then f(x0+m/M, y0+n/N)=f(m, n), M, N are the number of pixels in the horizontal and vertical directions (△x=I/M, △y=, I/N), m, n=0, ±1, ±2…
Assuming the above formula is periodic, we get
Since a clearly focused image has clearly discernible edge information, the image contains more high-frequency components. From the energy point of view, the increase in high-frequency components of the image means the increase in signal energy. In this way, the energy power spectrum function can be used to construct the image clarity function.
Among them, Pl(u, v) is the power spectrum function of the image, and L is the serial number of the image.
The difference between various clarity evaluation functions lies in the determination of the amount of high-frequency components of the image. Here, the method of weighting the high-frequency components of the image is adopted. At the same time, its weighting coefficient conforms to such a rule: as the frequency increases, its value also increases, which can reflect the amount of high-frequency components in the image. In the actual processing process, the distance from the pixel to the center pixel is used. Formula (4) is the value parameter that can reflect the image after weighting each high-frequency component in the spectrum of the image. Figure 3 is the comparison result of the clarity evaluation function based on the power spectrum described in C language and other methods. It can be seen from the figure that the image clarity evaluation function based on the power spectrum has a better judgment ability.
2 System Block Diagram
The block diagram of the FPGA-based automatic focusing system is shown in Figure 4. The image preprocessing process, the algorithm implementation process of the clarity * value function, and the algorithm implementation and focusing process of the motor control are all implemented in the FPGA and processed in real time. The module contains 5 modules and 3 parts, which are the input end, processing process and output end. SDRM and Flash chips are added in the processing process . The input and output use the DVI interface , which are TFP401 input DVI chip and TFP410 output DVI chip respectively . The FPGA core processing chip uses the Cycl ON e3EP3-C5F256C8N chip, which contains 100,000 logic gates, and 2 IS4232400 chips are used to provide buffering for image data.
From formula (4), it can be seen that for a 640×480 grayscale image, 1,228,800 multiplication operations, 307,200 square root operations, and 400 addition operations are required. Since the amount of calculation is particularly large and the changes in each image are not large, this system divides the image into 5 modules of 128×64 size. First, the 28×64 grayscale image is Fourier transformed, and then the power spectrum of the image is obtained. Then, the signal value is weighted to obtain the clarity value of a piece of the image instead of the clarity value of the entire image. At the same time, the "ping-pong" operation, dual butterfly processor multiplexing, and FPGA implementation of the radix 2FFT algorithm are adopted.
3 Focusing effect analysis
The analysis of the clarity*value algorithm and the multiplication implementation structure based on 2-FFT shows that the number of multiplication calculations of the clarity*value algorithm for this image is (53 248×3+64×32×3)=165 888 times, and the number of addition calculations required is (53 248×3+64×32×2-1)=163 839 times. From these data, it can be seen that the system delay during the focusing process is mainly the addition of the delays calculated in these two aspects, and there is also the delay of the circuit system, but this delay has been considered when designing the circuit and is limited to the minimum range. The "ping-pong" operation delay is used to add a delay of about 0.000 ls. When the system main frequency is 60 MHz, after the actual test system, the total delay is about 0.05s, and the acquisition time interval of 8 frames of images in the real-time processing system is required to be ×0.04=0.32s.
To meet the real-time requirements, the debugging of the above system is implemented in the Cyclone3EP3C5F256C8N chip, and the effect is quite satisfactory.
The focal length adjustment range of the lens is set to 60 segments, and the focus segment value range is [1, 60]. During the test, a group of images sent from the farthest end of the focal length are processed, and the search step number K is obtained to obtain the position that should be focused each time and the image clarity* value, as shown in Table 1. The position is where the image focusing effect is clearest.
4 Conclusion
The input port of this module directly inputs DVI signals, not a direct image acquisition port. In practical applications, the entire focusing process needs to be completed, a control circuit module for controlling the motor needs to be added, and the real-time performance of the entire focusing process needs to be comprehensively evaluated. In addition, an important application prospect of the automatic focusing method based on image technology is integration with C MOS image sensors . Since CMOS image sensors and FPGAs use the same manufacturing process, they can be integrated. After the CMOS image sensor is integrated with the automatic focusing function, it can not only simplify the design of the automatic focusing part of the imaging system, but also improve its competitiveness with CCD image sensors .
Previous article:Power consumption in FPGA design
Next article:Software and Hardware Design of Ozone Power Supply Control System Based on CPLD
Recommended ReadingLatest update time:2024-11-16 22:35
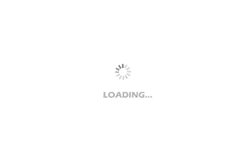
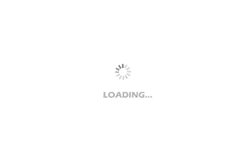
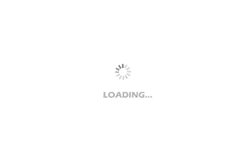
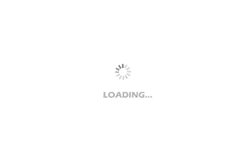
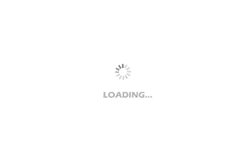
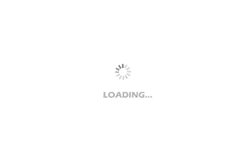
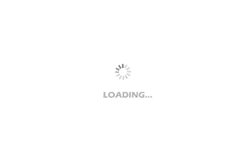
- Popular Resources
- Popular amplifiers
-
Analysis and Implementation of MAC Protocol for Wireless Sensor Networks (by Yang Zhijun, Xie Xianjie, and Ding Hongwei)
-
MATLAB and FPGA implementation of wireless communication
-
Intelligent computing systems (Chen Yunji, Li Ling, Li Wei, Guo Qi, Du Zidong)
-
Summary of non-synthesizable statements in FPGA
- Huawei's Strategic Department Director Gai Gang: The cumulative installed base of open source Euler operating system exceeds 10 million sets
- Analysis of the application of several common contact parts in high-voltage connectors of new energy vehicles
- Wiring harness durability test and contact voltage drop test method
- Sn-doped CuO nanostructure-based ethanol gas sensor for real-time drunk driving detection in vehicles
- Design considerations for automotive battery wiring harness
- Do you know all the various motors commonly used in automotive electronics?
- What are the functions of the Internet of Vehicles? What are the uses and benefits of the Internet of Vehicles?
- Power Inverter - A critical safety system for electric vehicles
- Analysis of the information security mechanism of AUTOSAR, the automotive embedded software framework
Professor at Beihang University, dedicated to promoting microcontrollers and embedded systems for over 20 years.
- Innolux's intelligent steer-by-wire solution makes cars smarter and safer
- 8051 MCU - Parity Check
- How to efficiently balance the sensitivity of tactile sensing interfaces
- What should I do if the servo motor shakes? What causes the servo motor to shake quickly?
- 【Brushless Motor】Analysis of three-phase BLDC motor and sharing of two popular development boards
- Midea Industrial Technology's subsidiaries Clou Electronics and Hekang New Energy jointly appeared at the Munich Battery Energy Storage Exhibition and Solar Energy Exhibition
- Guoxin Sichen | Application of ferroelectric memory PB85RS2MC in power battery management, with a capacity of 2M
- Analysis of common faults of frequency converter
- In a head-on competition with Qualcomm, what kind of cockpit products has Intel come up with?
- Dalian Rongke's all-vanadium liquid flow battery energy storage equipment industrialization project has entered the sprint stage before production
- Allegro MicroSystems Introduces Advanced Magnetic and Inductive Position Sensing Solutions at Electronica 2024
- Car key in the left hand, liveness detection radar in the right hand, UWB is imperative for cars!
- After a decade of rapid development, domestic CIS has entered the market
- Aegis Dagger Battery + Thor EM-i Super Hybrid, Geely New Energy has thrown out two "king bombs"
- A brief discussion on functional safety - fault, error, and failure
- In the smart car 2.0 cycle, these core industry chains are facing major opportunities!
- The United States and Japan are developing new batteries. CATL faces challenges? How should China's new energy battery industry respond?
- Murata launches high-precision 6-axis inertial sensor for automobiles
- Ford patents pre-charge alarm to help save costs and respond to emergencies
- New real-time microcontroller system from Texas Instruments enables smarter processing in automotive and industrial applications
- Prize-giving event: Blind box delivery | Murata’s Pet Pink Month is officially launched!
- AD packaging for self-use HR911105A 100M and HR911130A 1000M network ports
- Serial port expansion
- HR made inappropriate remarks in the circle of friends, made a public apology, and was fired!
- 【AT-START-F425 Review】No.02 AT32F425 Development Environment Construction
- About the use of AWR1642BOOST development system
- Excellent "Internet of Things" (IoT) video recommendation, it explains it very thoroughly!
- Design of TPS53355 ripple injection circuit
- How to realize serial communication between DSP and PC
- DIY GPS Tracker