As a field engineer at Xilinx, I often ask the question: Can we provide a DSP core with features that meet all of a customer's unique design requirements? Sometimes a core is too big, too small, or not fast enough. Sometimes we develop a core that does exactly what a customer needs and quickly release it under the CORE GeneratorTM brand. But even in these cases, the customer still wants a specific set of DSP features, and they can't wait. In these cases, I often recommend that they customize their DSP functions using the interpolation lookup tables in our devices.
A lookup table (LUT) is essentially a storage element that "looks up" the output for any given combination of input states, ensuring that there is an exact output for each input. Using a LUT to implement DSP functions has some significant advantages:
You can change the LUT contents using a high-abstraction-level programming language such as MATLAB® or Simulink®.
You can design a DSP function to run mathematical functions that would be extremely difficult using discrete logic operations, such as ly=log(x), y=exp(x), y=1/x, y=sin(x), etc.
LUTs also make it easy to implement complex math functions that might require too many FPGA resources in terms of configurable logic blocks (CLB)l chips, as well as embedded multiplication units or DSP48 programmable multiply-accumulate (MAC) units.
However, there are of course some drawbacks to using LUTs in this way. When you use LUTs to implement DSP functions, you must use block RAM (BRAM) elements. If you implement the function y=sqrt(x) (where x represents the 16-bit input and y represents the 18-bit output), you will need about 64 18KB BRAM cells per variable. If, for example, your goal is to implement a small Spartan® device, or you have too many operations to perform to spare 64 BRAM cells per variable, it is recommended that you abandon this approach that requires so many BRAM cells, which is too costly from a system architecture perspective.
The interpolation LUT approach has all the advantages of the LUT approach in implementing DSP functions without using so many BRAM cells. With this approach, you can use the continuous output from a smaller LUT (for example, a 1000-word LUT) and interpolate it linearly to simulate a larger LUT. In this way, you can achieve higher numerical resolution than a 1000-word LUT. In addition, with this approach, only 1 BRAM, 1 embedded multiplier (or DSP48), and a few CLB chips are needed to implement the control logic, so the cost of using LUTs becomes more reasonable. And from the perspective of signal-to-noise ratio, its numerical accuracy is also very satisfactory.
Of course, applying the interpolation LUT (ILUT) method requires some skill. For example, the performance of the ILUT in terms of area usage, timing, and numerical accuracy can be clearly demonstrated when the method is used to implement the y=sqrt(x) function. Let’s take a look at this example first, and then I will go through some examples of how this method can be used to meet very different customer needs, such as linearizing a sensor with a nonlinear transfer function and implementing an adaptive finite impulse response (FIR) filter to remove speckle noise on a synthetic aperture radar (SAR) image.
Figure 1. Top-level block diagram of the interpolation lookup table in System Generator for DSP.
Designing with System Generator for DSP
To implement the DPS algorithm on a Xilinx FPGA, I used the System Generator for DSP design and synthesis tool, which uses the MathWorks Simulink model-based design methodology. System Generator, which benefits from Xilinx’s DSP blockset in the Simulink environment, automatically calls CORE Generator to generate highly optimized netlists for DSP building blocks. Simulink is a double-precision floating-point design tool, while System Generator is a fixed-point arithmetic tool. Regardless, using the two tools together, you can define the total number of bits per signal and the binary position of each signal to manipulate fractions in a smart way in fixed-point arithmetic. The simulation results are cycle-accurate and bit-true, so you can easily compare them to floating-point reference values generated by MATLAB scripts or Simulink blocks to check for quantization errors.
Figure 1 shows the top-level block diagram of the ILUT solution in System Generator. To make this approach as general as possible, assume that the input variable x in nx=16 bits has a value range of 0≤x<1, so its format is "unsigned 16 bits plus 16 bits to the right of the binary point", also known as Ufix_16_16 format. The most significant bit (MSB) and least significant bit (LSB) modules correspond to the highest bit of the input data nb=10 and the lowest bit of nx-nb=6, respectively. These signals are named x0 and dx. The output y=sqrt(x) is represented by a ny=17-bit binary number in the format: Ufix_17_17.
Figure 2 shows the steps for implementing a small 1000-word LUT using a dual-port RAM module. Since this module is a read-only memory, the Boolean constant module We_const forces writes to zero. The signals X0 and X0+1 are used as the next two addresses on the ROM table. The zero constant of the Data_const module defines the size of any ROM word (ny in this case).
The following formula shows how to interpolate a point with coordinates (x, y) between two known points (x0, y0) and (x1, y1), with x0 being the most significant bit of x:
Note that X1 and X0 are adjacent addresses of this small capacity LUT, separated by only one least significant bit. Since the address space of this small capacity LUT is nb bits, the value of the LSB is 2-nb.
Figure 2 Small-capacity LUT diagram in System Generator for DSP
Figure 3 Linear inset diagram of System Generator for DSP
The interpolation steps are shown in Figure 3. The “Reinterpret” block changes the dx=x-x0 signal without changing the binary representation. It resets the binary point (from UFix_6_0 to UFix_6_6 format) and outputs a fraction of nx-nb binary digits, thus calculating the value of (x-x0)/2-nb.
From a hardware perspective, these blocks take up nothing. In general (and depending on the type of function we apply via the ILUT method), if y1=0 and y0=0, we can force y1- y0=1, so that we get 1/2-nb instead of 0. We use the Mux, Rational, Constant, and Constant1 blocks to perform this work. The remaining Mult, Add, and Sub blocks implement the linear interpolation formula. In this case, I forced the output signal of the Mult block to have a 17-bit resolution instead of the theoretically required 23 bits, because the overall numerical accuracy is sufficient for this experiment. In addition, since the y-sqrt(x) function is monotonically increasing, all results are unsigned. In other words, different functions require different careful adjustments to the data type, but they will not deviate far from the principle shown in Figure 3.
Assuming that we use Spartan-3E 1200 (fg320-4) as the target device, we now use the ISE design suite and System Generator for DSP 10.1 SP3 version tools to place and route it. The overall situation of the FPGA resources occupied is as follows:
The design is fully pipelined and can provide new outputs on any clock cycle. The latency is 10 clock cycles and the maximum data rate is 194.70MSPS (million samples per second). In terms of numerical accuracy, the ratio of the quantization error of the reference floating-point result to the fixed-point output of System Generator for DSP, i.e., the signal-to-noise ratio, is 71.94dB or 77.95dB for a 1000 or 2000-word ILUT, respectively.
In addition to ILUT, we can also apply the CORDIC SQRT block from the Reference Math Blockset provided by Xilinx System Generator for DSP. In this example, the total latency is 37 clock cycles, the maximum data rate is 115.18 MSPS, the area resource usage is 940 flip-flops, there are a total of 885 four-input LUTs, 560 occupied chips, and two MULT 18x18 embedded multipliers. The signal-to-noise ratio is 40.64dB. These results show that CORDIC is an ideal method for implementing fixed-point math operations, but ILUT is better in many ways.
Linearizing nonlinear sensors
Currently, many companies use "smart sensors" in industrial control systems to meet requirements such as low footprint, low power consumption, high performance, lowest cost, and shortest development time. A general smart sensor can be considered as a functional component consisting of a sensor and its signal control circuit, an analog-to-digital converter (ADC), and an associated DSP subsystem with or without an embedded processor, all of which are integrated on the same device, as shown in Figure 4.
The purpose of a smart sensor is to convert a physical quantity, such as the current in a motor, into a digital signal that can be processed by digital circuits. The technology used to build these sensors and certain characteristics of the components often lead to errors such as offset, gain, and nonlinearity, which in turn lead to a nonlinear overall transfer function.
Typically, customers will correct for the above errors in the DSP subsystem running in their products. If y=f(x) is the digital output signal from the sensor and ADC cascade, then the DSP must perform its inverse function g(y)=f-1(y) to compensate for the nonlinear function, so that the overall output z is:
This is the equation of a line with slope m and y-intercept b.
Figure 4. Block diagram of a smart sensor
The simplest linearization method is the LUT method, which uses sensor calibration points stored in ROM. However, for a 16-bit ADC, the ROM is too large and requires 64 BRAM cells. The interpolation LUT is not the case and is a good solution.
For example, let's assume that the nonlinear transfer function is a parabola. The next MATLAB code snippet shows how to generate the m and b parameters of the final line, and how to calculate g(y), the inverse function of f(x). Figure 5 shows three different curves in three colors. Please note that some values are lost in the process of calculating the inverse function g(y) of f(x). This is because there are several points with the same y value corresponding to different x points. Therefore, g(y) needs to be smoothed to fill in all the missing points. (For the sake of accuracy, I did not include this part of the operation in the MATLAB code snippet)
Figure 5. The black parabola represents the curve of the nonlinear sensor transfer function f(x); the green straight line represents the final linear sensor transfer function curve obtained by linearizing the DSP subsystem; and the blue parabola represents the curve of the inverse function g(y).
Using a design very similar to that shown in Figures 1-3, I ran a fixed-point cycle-based simulation in System Generator for DSP and obtained a 92.48 dB SNR over the full output range of the nonlinear sensor
.
Tracking high-speed moving systems, such as missiles, is a challenging task that requires very complex DSP algorithms and a variety of different types of detection media, such as synthetic aperture radar (SAR). As a typical coherent electromagnetic source (such as laser), SAR imagers are also affected by speckle noise. Therefore, the first stage of any SAR-based DSP chain is a two-dimensional (2D) adaptive FIR filter to reduce this noise (but it is impossible to completely eliminate it). Figure 6 shows a MATLAB simulation of speckle noise. This noise has a comprehensive adverse effect on the image quality on the left. The image on the right is the output of the 2D FIR filter golden model.
Figure 6. Speckle noise affects the image quality on the left, and the image on the right is filtered.
Speckle noise is a multiplicative noise with an exponential distribution, which is completely determined by its variance value σ. Therefore, the widely used method to combat speckle noise is the Frost filter (named after the inventor VSFrost). VSFrost discussed this phenomenon in a paper published in 1981. In a 3x3 matrix, it can be modeled with the following formula:
Where xij and yij represent the input and output samples of the Frost filter, respectively. K is the gain factor that controls the strength of the filter (for convenience, I assume K=1 below), μ1 and σ are the mean and variance values of the 2D kernel, respectively, and Tij is the distance matrix between the center output pixel (coefficient ij=22) and all surrounding pixels. The following equation shows that the key factor in implementing this filter is R1, which is the ratio between the first-order μ1 and the second-order μ2 in the 3x3 matrix:
The value range of R1 is between 0 and 1. According to experiments, it is found that to achieve good numerical accuracy, R1 can be represented by a 16-bit to 20-bit binary number.
After I designed the R1 calculation steps in the system Generator for DSP, I decided to implement the normalization of the filter coefficients through an interpolation LUT. The content of the LUT is represented by the following MATLAB code:
Figure 7 shows the curves of the normalized coefficients along the R1 input signal. There are only three curves here because the Tij matrix is symmetrically distributed around the center pixel with coefficient ij=22. According to the curves, the numerical results show a signal-to-noise ratio between 81.28 and 83.38 dB compared to the pure floating-point reference model. For the interested reader, the following MATLAB code fragment illustrates the 2D filter process (the ILUT function is not included for simplicity).
Figure 7 Normalized coefficients along the distribution of speckle noise denoising filter parameter R1
In short, these examples show that interpolation lookup tables are a simple and powerful way to implement DSP functions in Xilinx FPGAs. Interpolation lookup tables can help you achieve very high numerical accuracy (SNR) and high data rates while keeping the area footprint relatively low.
Previous article:Design of Laser Marking Controller Based on TMS320F2812 DSP
Next article:Electromagnetic compatibility design at circuit board level for high-speed DSP systems
Recommended ReadingLatest update time:2024-11-16 16:43
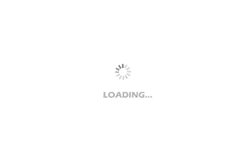
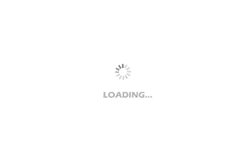
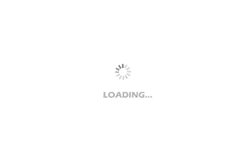
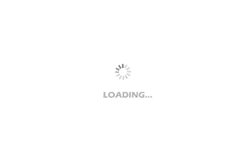
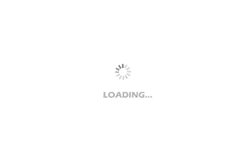
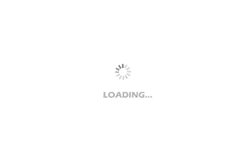
- Popular Resources
- Popular amplifiers
-
Analysis and Implementation of MAC Protocol for Wireless Sensor Networks (by Yang Zhijun, Xie Xianjie, and Ding Hongwei)
-
MATLAB and FPGA implementation of wireless communication
-
Modern arc welding power supply and its control
-
Intelligent Control Technology of Permanent Magnet Synchronous Motor (Written by Wang Jun)
- Huawei's Strategic Department Director Gai Gang: The cumulative installed base of open source Euler operating system exceeds 10 million sets
- Analysis of the application of several common contact parts in high-voltage connectors of new energy vehicles
- Wiring harness durability test and contact voltage drop test method
- Sn-doped CuO nanostructure-based ethanol gas sensor for real-time drunk driving detection in vehicles
- Design considerations for automotive battery wiring harness
- Do you know all the various motors commonly used in automotive electronics?
- What are the functions of the Internet of Vehicles? What are the uses and benefits of the Internet of Vehicles?
- Power Inverter - A critical safety system for electric vehicles
- Analysis of the information security mechanism of AUTOSAR, the automotive embedded software framework
Professor at Beihang University, dedicated to promoting microcontrollers and embedded systems for over 20 years.
- Innolux's intelligent steer-by-wire solution makes cars smarter and safer
- 8051 MCU - Parity Check
- How to efficiently balance the sensitivity of tactile sensing interfaces
- What should I do if the servo motor shakes? What causes the servo motor to shake quickly?
- 【Brushless Motor】Analysis of three-phase BLDC motor and sharing of two popular development boards
- Midea Industrial Technology's subsidiaries Clou Electronics and Hekang New Energy jointly appeared at the Munich Battery Energy Storage Exhibition and Solar Energy Exhibition
- Guoxin Sichen | Application of ferroelectric memory PB85RS2MC in power battery management, with a capacity of 2M
- Analysis of common faults of frequency converter
- In a head-on competition with Qualcomm, what kind of cockpit products has Intel come up with?
- Dalian Rongke's all-vanadium liquid flow battery energy storage equipment industrialization project has entered the sprint stage before production
- Allegro MicroSystems Introduces Advanced Magnetic and Inductive Position Sensing Solutions at Electronica 2024
- Car key in the left hand, liveness detection radar in the right hand, UWB is imperative for cars!
- After a decade of rapid development, domestic CIS has entered the market
- Aegis Dagger Battery + Thor EM-i Super Hybrid, Geely New Energy has thrown out two "king bombs"
- A brief discussion on functional safety - fault, error, and failure
- In the smart car 2.0 cycle, these core industry chains are facing major opportunities!
- The United States and Japan are developing new batteries. CATL faces challenges? How should China's new energy battery industry respond?
- Murata launches high-precision 6-axis inertial sensor for automobiles
- Ford patents pre-charge alarm to help save costs and respond to emergencies
- New real-time microcontroller system from Texas Instruments enables smarter processing in automotive and industrial applications
- Please help me with the trip zone configuration of TI's 28034!!!
- Is the threshold for electromagnetic wave and antenna major getting lower and lower? Is the reliance on simulation software getting higher and higher?
- EV-HC32F460_1. Unboxing
- The input capture of stm8s cannot enter the interrupt. Please help? ? Thank you!!!
- 10 bit serial controlled analog to digital converter (MS1549)
- [NXP Rapid IoT Review] I2C Program Small Bug
- EEWORLD University Hall ---- e-Network Lecture Hall
- GD32E231 DIY Part 4: Software Architecture
- What is SiC (Silicon Carbide)? The difference between SiC-MOS and Si-MOS
- Senior maker Chunyang shares tips: Electronic product development creativity & clever use of online resources to help electronic product development