Recently, two things happened in the autonomous driving circle, which are very interesting to compare: One happened on the other side of the ocean. Argo AI, a L4 autonomous driving unicorn that was once favored by two international auto giants (Ford and Volkswagen), suddenly announced its bankruptcy. Ford CEO Jim Farley said that the closure of Argo AI was because the commercial prospects of L4 autonomous driving (mainly Robotaxi) were unclear, the investment was large and the results were minimal, so he decided to stop burning money first and concentrate resources on L2 and L2+ autonomous driving to promote pre-installed mass production. This has attracted widespread attention from domestic autonomous driving practitioners. Do we still need to burn money for L4? Can driverless driving be realized? When will it be realized? Observers have raised a series of soul-searching questions.
Another incident happened in China. On the first day of November, the autonomous driving technology company QCraft officially named its autonomous driving solution "Driven-by-QCraft" as "QCraft Chengfeng". It covers multiple aspects such as autonomous driving vehicle software, vehicle hardware and data automation closed loop, and can provide QCraft with the latest perception, PNC (planning and control) and other capabilities. It is a very competitive autonomous driving pre-installed mass production solution, and its most prominent function is urban navigation assisted driving (urban NOA).
As a representative of the OEM, Ford has now officially embraced the mass production of pre-installed autonomous driving; as a representative of the solution supplier, Qingzhou Zhihang has also embraced the mass production of pre-installed autonomous driving. Car companies and autonomous driving companies in the industry are "running in both directions" in a sense. Putting aside the so-called "soul" and "body" dispute, the current stage is still the beginning of the large-scale popularization of autonomous driving functions. It requires the cooperation of both OEMs and suppliers to make the cake bigger, and dividing the cake is a later story. OEMs are also in need of intelligent driving suppliers: Jidu, BYD, and Dongfeng Lantu need Baidu Apollo; SAIC, Zhiji, and GM need Momenta; Arcfox and Avita need Huawei ADS; Volkswagen needs Horizon; and these car companies will never use only one supplier, they will definitely adopt a multi-solution strategy.
01
From L2 or L4? The trend is becoming clearer
In the past few years, the commercialization of autonomous driving technology has split into two paths: one is directly L4, developing Robotaxi and other unmanned driving scenarios; the other is the pre-installed mass production of intelligent assisted driving for passenger cars, which belongs to L2+ level autonomous driving. On the market, several Robotaxi companies have removed the main driver safety officer and started charging for operation; the Pilot system, navigation assisted driving system, and automatic parking system on various mass-produced cars have begun to be accepted by more consumers and become an important consideration for them to buy cars.
Especially recently, navigation-assisted driving functions have begun to enter cities, and this group of L2+ assisted driving has entered the home turf of Robotaxi, and the two forces are converging. The computing platform and sensors used by urban NOA are actually gradually converging with Robotaxi. Although the cost is slightly higher, it is within a controllable range, and the capability planning is also similar, including stable lane keeping, traffic light recognition, automatic left and right turns, automatic detours, etc. The biggest difference is whether there is a driver in the main car, but this is only formal. It seems that companies starting from pre-installed mass production are already ahead of companies starting from Robotaxi on the road to commercialization, and they are not inferior in technology. In the past two years, many L4 companies have also quickly or slowly entered the pre-installed mass production business of autonomous driving, hoping to obtain some cash flow and collect more road driving data, such as Baidu Apollo, Momenta, Qingzhou Zhihang, Yuanrong Qixing, WeRide, Zhixingzhe, etc., and they have not given up on deploying Robotaxi fleets, which is equivalent to walking on two legs instead of just hanging Robotaxi. Some of these companies are increasingly focusing on pre-installed mass production business, and Momenta is the most typical example, which has successively received cooperation orders from many OEMs.
Ford's decision to shut down Argo AI shows that at this stage, it is still necessary to do a good job in the pre-installed mass production of autonomous driving systems. OEMs currently need L2 and L2+ autonomous driving systems, not L4. In this way, the domestic L4 companies that have transformed in advance are quite foresighted. However, it is not easy for software companies to mass produce automotive-grade systems. The stability and maturity of the algorithm, the adaptability of the algorithm, the automotive-grade of the computing hardware, the matching of the algorithm and hardware, the compression of costs, the support of the team and tool chain, etc. are all difficult problems facing algorithm companies.
Extreme cost control and extreme compression of development cycle, the current mass production of autonomous driving is so "competitive". Even if "pre-embedded hardware" has helped many OEMs and suppliers to buy time, consumers are definitely unwilling to use the functions they paid for 1-2 years after picking up the car. Suppliers must ensure the delivery of the system quickly and well. Whoever can do all of this well, constantly build strengths and make up for weaknesses, will have a better chance of running out. Just in time for the release of the "Qingzhou Chengfeng" plan, we will take Qingzhou Zhihang as a blueprint to see what qualities an autonomous driving technology supplier needs to have in order to do a good job in L2+ systems, urban NOA, and pre-installed mass production services.
02
"Dual Engine" drive, "Ride the Wind" released
In the past year, QINGZHOU Zhihang has completed the adjustment of its corporate development strategy, expanding its business from L4 only to L4 and pre-installed mass production "dual engines".
Founded in 2019, QINGZHOU Zhihang initially moved towards the research and development goal of L4 autonomous driving technology. Its main vehicle models are the unmanned minibus Robobus, as well as the Robotaxi fleet that is being tested and operated in cooperation with T3 Travel. At the beginning, it also aimed high in order to accumulate its own autonomous driving software and hardware algorithms, construct its own database, scenario library, model library, and create a complete set of autonomous driving algorithm factories.
In 2020, QINGZHOU Zhihang launched the first generation of autonomous driving solutions "Driven-by-QCraft" focusing on complex urban traffic scenarios. It is suitable for a variety of complex road conditions on urban public roads and can be efficiently deployed on a variety of vehicle models. After almost 3 years of accumulation, when these technologies gradually matured and their versatility and portability were very high, QINGZHOU Zhihang decided to develop new businesses and enter the pre-installed mass production of high-level autonomous driving systems. In May 2022, QINGZHOU Zhihang launched the latest generation of "Driven-by-QCraft", a car-grade pre-installed mass production autonomous driving solution, to help automakers achieve the full implementation of the urban NOA solution. Because this solution has strong software adaptability to hardware, it can do from L2+ to L4 autonomous driving.
Basically, QINGZHOU Zhihang has taken a path starting from L4 - accumulating technical capabilities (including perception, PNC technology, data-driven, algorithmic large models, etc.) - expanding pre-installed mass production business - L4 business and pre-installed mass production business feed back each other technically, and form a "dual engine" road for autonomous driving development. Its goal is to empower OEMs with easy-to-use autonomous driving capabilities and ultimately allow consumers to use such products. On November 1, 2022, QINGZHOU officially named its autonomous driving solution "Driven-by-QCraft" as "Ride the Wind", which is a complete set of software and hardware solutions that are adapted to computing platforms such as Horizon Journey 5 and NVIDIA Orin, as well as different numbers of lidar and camera perception hardware combinations. This set of algorithms includes core technologies such as fusion perception, predictive planning control, data-driven, and algorithmic large models.
The arrival of "Qingzhou Chengfeng" makes Qingzhou Zhihang's corporate and market positioning clearer: an autonomous driving technology company, a new TIer 1 for smart cars. Qingzhou Zhihang believes that urban NOA is the ceiling of assisted driving and the entry threshold for unmanned driving, so their current goal is to allow more car owners to enjoy the convenience brought by urban NOA.
03
QINGZHOU Zhihang cures the algorithm anxiety of car companies
How to solve algorithmic problems?
Since urban NOA is the ceiling of assisted driving, it is also the ceiling in terms of technical difficulty. How difficult is it? Wu Xinzhou, vice president of autonomous driving at Xiaopeng Motors, gave a set of data to quantify it: Xiaopeng's urban NGP code volume is 6 times that of high-speed NGP, the number of perception models is 4 times that of high-speed NGP, and the code volume of prediction/control/planning (PNC module) is 88 times that of high-speed NGP. From this set of data, the PNC module is the most difficult part of autonomous driving in the city. Why is it difficult?
Previous article:Analysis of the technical principles of NIO's next-generation electronic and electrical architecture
Next article:Graphical introduction to foreign electric vehicle triads - electric drive systems
Recommended ReadingLatest update time:2024-11-16 19:49
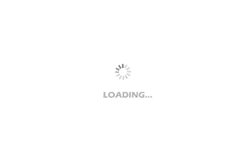
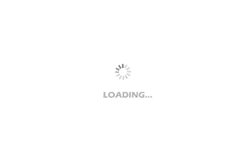
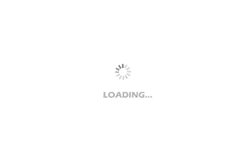
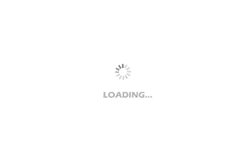
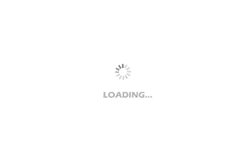
- Popular Resources
- Popular amplifiers
-
A review of deep learning applications in traffic safety analysis
-
Dual Radar: A Dual 4D Radar Multimodal Dataset for Autonomous Driving
-
A review of learning-based camera and lidar simulation methods for autonomous driving systems
-
Multimodal perception parameterized decision making for autonomous driving
- Huawei's Strategic Department Director Gai Gang: The cumulative installed base of open source Euler operating system exceeds 10 million sets
- Analysis of the application of several common contact parts in high-voltage connectors of new energy vehicles
- Wiring harness durability test and contact voltage drop test method
- Sn-doped CuO nanostructure-based ethanol gas sensor for real-time drunk driving detection in vehicles
- Design considerations for automotive battery wiring harness
- Do you know all the various motors commonly used in automotive electronics?
- What are the functions of the Internet of Vehicles? What are the uses and benefits of the Internet of Vehicles?
- Power Inverter - A critical safety system for electric vehicles
- Analysis of the information security mechanism of AUTOSAR, the automotive embedded software framework
Professor at Beihang University, dedicated to promoting microcontrollers and embedded systems for over 20 years.
- Innolux's intelligent steer-by-wire solution makes cars smarter and safer
- 8051 MCU - Parity Check
- How to efficiently balance the sensitivity of tactile sensing interfaces
- What should I do if the servo motor shakes? What causes the servo motor to shake quickly?
- 【Brushless Motor】Analysis of three-phase BLDC motor and sharing of two popular development boards
- Midea Industrial Technology's subsidiaries Clou Electronics and Hekang New Energy jointly appeared at the Munich Battery Energy Storage Exhibition and Solar Energy Exhibition
- Guoxin Sichen | Application of ferroelectric memory PB85RS2MC in power battery management, with a capacity of 2M
- Analysis of common faults of frequency converter
- In a head-on competition with Qualcomm, what kind of cockpit products has Intel come up with?
- Dalian Rongke's all-vanadium liquid flow battery energy storage equipment industrialization project has entered the sprint stage before production
- Allegro MicroSystems Introduces Advanced Magnetic and Inductive Position Sensing Solutions at Electronica 2024
- Car key in the left hand, liveness detection radar in the right hand, UWB is imperative for cars!
- After a decade of rapid development, domestic CIS has entered the market
- Aegis Dagger Battery + Thor EM-i Super Hybrid, Geely New Energy has thrown out two "king bombs"
- A brief discussion on functional safety - fault, error, and failure
- In the smart car 2.0 cycle, these core industry chains are facing major opportunities!
- The United States and Japan are developing new batteries. CATL faces challenges? How should China's new energy battery industry respond?
- Murata launches high-precision 6-axis inertial sensor for automobiles
- Ford patents pre-charge alarm to help save costs and respond to emergencies
- New real-time microcontroller system from Texas Instruments enables smarter processing in automotive and industrial applications
- Operational issues of XDS100V3.0 emulation motoware built-in project
- GD32E231 Learning 3: SPI driving OLED
- What documents does the patch welding factory need?
- Pnp transistor collector connected to power supply problem
- Record the implementation of a cross-platform MQTT client based on Socket and make it into an RTT software package
- AI cooling requires a "core" under the scorching sun. Infineon tests your knowledge of AI chips
- [Review of Arteli Development Board AT32F421] 3. FreeRTOS porting [with source code]
- Sallen-Key low-pass filter reference ground
- STLM75 evaluation board STEVAL-MKI204V1K data
- TMS320F28335——SPI usage notes