Tesla will hold an autonomous driving investor day event on the 22nd of this month, and hopefully it will be able to provide some details about its autonomous driving research and development work at this event;
the realization of fully autonomous driving has huge financial implications, which makes certain technical issues particularly important;
for investors, the key question at present is whether Tesla can use its advantage of training large-scale data in the real physical world to achieve better autonomous driving than its competitors.
Recently, Tesla announced that it will hold an autonomous driving investor day on the 22nd of this month. The following is the content of its announcement:
“There are exciting developments coming to Tesla in the coming weeks and months that we can’t wait to share with you. We will be holding our Investor Day at our headquarters in Palo Alto, where we will provide a deeper dive into our autonomous driving technology and roadmap.
We are offering investors a test drive so they can experience our latest self-driving software, including some features and functions that are currently under active development. Elon Musk, VP of Engineering Stuart Bowers, VP of Hardware Engineering Pete Bannon, and Senior Director of Artificial Intelligence Andrej Karpathy will also be on hand to speak.”
Here are five questions I hope investors will ask Tesla at the Autopilot Investor Day:
1. It is clear that Tesla is using deep supervised learning for computer vision tasks. But for the actual driving tasks - route planning and driving strategy (to use Tesla's own terminology), is Tesla using imitation learning or reinforcement learning, or both? In imitation learning, the neural network learns to drive by observing human driving behavior and associating sensory information with driver behavior, while in reinforcement learning, learning is done through trial and error (usually through simulation).
2. Whatever approach Tesla uses for driving tasks (as opposed to computer vision tasks), why does Tesla think its approach works when companies like Google Waymo are still struggling? Why does Tesla have a unique advantage in implementing this approach? For example, if Tesla chose the imitation learning approach, then it has a unique advantage because of the relatively large number of cars it has sold, so we hope that Tesla can provide us with a proof of why imitation learning works. And why pure reinforcement learning in simulation alone will not work.
3. How far has Tesla come in solving the necessary computer vision tasks? How can Tesla set a standard to measure the completion of this task? Is the remaining work just adding more labeled data to the training set?
4. How accurate is Tesla's sensor suite in acquiring depth information about surrounding objects? LiDAR is sometimes touted as having an advantage in depth mapping. What are the advantages and disadvantages of Tesla's sensor suite compared to LiDAR?
5. Has Tesla discovered the potential of end-to-end learning? Or is it still far from the practical stage of end-to-end learning? How to combine end-to-end learning with unsupervised representation learning? In end-to-end learning, neural networks are trained through imitation learning or reinforcement learning to generate actions based on raw sensor input without the need for human labeling at any stage of the process. In unsupervised learning, neural networks learn to predict future data from past data without any manual labels.
At present, we know almost nothing about Tesla's technology strategy. We can certainly speculate on its technology strategy based on some evidence we have now, but we are not sure what Tesla is doing behind the scenes. Tesla may just hold a normal autonomous driving investor day this time and be cryptic about the technical issues I listed above, but I would rather use this event to openly explain to investors in detail what Tesla is doing now and talk about why the technology strategy it is executing is the right choice.
According to the financial model released by ARK Invest, if Tesla can launch a fully autonomous car in the next five years, its stock price will increase by about 8 to 14 times from the current level. Although different analysis companies have different financial forecast models for Tesla, many companies believe that the potential long-term value of autonomous driving far exceeds Tesla's current market value. If a company can successfully deploy fully autonomous driving technology, then investing in it blindly is a simple and crude logic. But the question now is whether fully autonomous driving can be achieved from the perspective of technical feasibility, and if so, which company can achieve it. This is why it is important for investors to understand Tesla's autonomous driving technology strategy.
The key question for Tesla investors now is how to draw a conclusion from the answers to the above five questions: can Tesla truly use the vast amount of training data from the 450,000 cars it has sold to achieve better self-driving performance than other companies? What makes Tesla different is its vast amount of training data. But this resource will only determine Tesla's competitive advantage if the winning technology requires a lot of training data, and this data does not require the bottleneck of expensive and slow human labeling. Imitation learning and end-to-end learning are such technology solutions. The opposite solution is to use hand-coded rules to tell the self-driving car what to do, which does not use any training data from real driving. Another opposite solution is pure reinforcement learning, which uses computers to generate training data in a simulated world instead of using data from the real world.
Therefore, whether Tesla is competitive depends on whether its huge amount of real-world training data is the decisive resource for the final winning solution. Whether Tesla's real-world data is the decisive resource depends on whether the winning solution is data-intensive (like imitation learning or end-to-end learning) or data-insignificant (like hand-coded or pure reinforcement learning in the simulated world).
In other words, Tesla's competitiveness actually depends on two aspects. First, what is the technical route that Tesla is currently implementing? Second, is the technical solution it has chosen correct? Then, the corresponding information that investors need to get from Tesla is, first, an explanation of the current technical route, and second, a demonstration of the correctness of the selected technical solution.
Based on a series of investigative reports, I believe Tesla is using "imitation learning solutions" to advance autonomous driving tasks. I am optimistic about this solution for two main reasons. First, DeepMind's AlphaStar project has strikingly demonstrated the power of pure imitation learning and using imitation learning to enhance reinforcement learning. Second, experts from Waymo and Uber ATG have also publicly emphasized that imitation learning is a very promising solution for autonomous driving tasks.
Furthermore, I am skeptical of the hand-coded approach because, so far, it has had very few successes because humans
Sometimes it is difficult to formalize complex tasks into a set of established rules, so I am on the fence about this. In addition, I am skeptical about using pure reinforcement learning in simulation, because in my opinion, a realistic model of human driving behavior is necessary for simulation so that the neural network can learn how to interact with other drivers in complex situations. And to create a realistic model of human driving behavior is equivalent to building a fully autonomous car, so there is a chicken and egg problem here.
If we can get enough information at Tesla's Autopilot Investor Day to prove the advantages of imitation learning solutions, and Tesla can prove why its solution works and other solutions don't work, then this event will be a very important event. Personally, I think it is much more important than Tesla announcing its latest quarterly earnings or how many vehicles it delivered.
Previous article:Musk plans to produce more than 500,000 Tesla vehicles in the next 12 months
Next article:How to make electric vehicles profitable and profitable?
Recommended ReadingLatest update time:2024-11-16 03:36
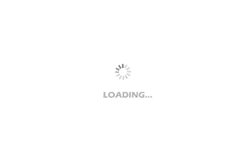
- Popular Resources
- Popular amplifiers
- Huawei's Strategic Department Director Gai Gang: The cumulative installed base of open source Euler operating system exceeds 10 million sets
- Analysis of the application of several common contact parts in high-voltage connectors of new energy vehicles
- Wiring harness durability test and contact voltage drop test method
- Sn-doped CuO nanostructure-based ethanol gas sensor for real-time drunk driving detection in vehicles
- Design considerations for automotive battery wiring harness
- Do you know all the various motors commonly used in automotive electronics?
- What are the functions of the Internet of Vehicles? What are the uses and benefits of the Internet of Vehicles?
- Power Inverter - A critical safety system for electric vehicles
- Analysis of the information security mechanism of AUTOSAR, the automotive embedded software framework
Professor at Beihang University, dedicated to promoting microcontrollers and embedded systems for over 20 years.
- LED chemical incompatibility test to see which chemicals LEDs can be used with
- Application of ARM9 hardware coprocessor on WinCE embedded motherboard
- What are the key points for selecting rotor flowmeter?
- LM317 high power charger circuit
- A brief analysis of Embest's application and development of embedded medical devices
- Single-phase RC protection circuit
- stm32 PVD programmable voltage monitor
- Introduction and measurement of edge trigger and level trigger of 51 single chip microcomputer
- Improved design of Linux system software shell protection technology
- What to do if the ABB robot protection device stops
- Allegro MicroSystems Introduces Advanced Magnetic and Inductive Position Sensing Solutions at Electronica 2024
- Car key in the left hand, liveness detection radar in the right hand, UWB is imperative for cars!
- After a decade of rapid development, domestic CIS has entered the market
- Aegis Dagger Battery + Thor EM-i Super Hybrid, Geely New Energy has thrown out two "king bombs"
- A brief discussion on functional safety - fault, error, and failure
- In the smart car 2.0 cycle, these core industry chains are facing major opportunities!
- The United States and Japan are developing new batteries. CATL faces challenges? How should China's new energy battery industry respond?
- Murata launches high-precision 6-axis inertial sensor for automobiles
- Ford patents pre-charge alarm to help save costs and respond to emergencies
- New real-time microcontroller system from Texas Instruments enables smarter processing in automotive and industrial applications
- Free application: RTT & Renesas ultra-low power MCU RA2L1 development board
- Where to go during the May Day holiday?
- The 5G era is within reach! Qorvo RF Fusion has been successfully applied to many new smartphone designs
- I want to make a game console recently, but in order to prevent others from copying it, what is the best way to do it?
- The firmware development kit has been upgraded, ONSemiconductor.BDK.1.18.0.pack
- Linux builds RVB2601 development environment
- Wi-Fi 6 and Wi-Fi 6E: The key to the Internet of Things
- Two boards can be cascaded, but not placed on the same layout.
- [ATmega4809 Curiosity Nano Review] How to use RTC
- EEWORLD University Hall----Set up UCD3138 for JTAG communication