After Tesla's "sledgehammer on the door", Nvidia released a chip called "Orin".
Regardless of what the "ethical issues" are, Huang Xiaoming gave several technical parameters at GTC China 2019:
17 billion transistors
8 core 64-bit CPU
200 TOPS deep learning computing power
Compatible with L2-L5 autonomous driving development
Achieve ISO 26262 ASIL-D and other system safety standards
2022 SOP
Interpretation——
Compared with the previous generation system-level chip Xavier, Orin's computing power has increased by nearly 7 times and is backward compatible with Xavier.
Orin supports the simultaneous operation of its own new generation GPU architecture and Arm Hercules CPU core to improve fault tolerance
Provide a low-cost version for OEMs, which can meet the needs of L2 autonomous driving with a single camera and can also use the software stack of the entire autonomous driving product line
"Leather Yellow" highly condenses three key words: "scalable", "programmable" and "software defined".
However, compared with the nuclear bomb-level release speed of "a new product every few minutes" in previous years, such a small SoC chip could not stop the more than 6,100 people present from asking: "How come Nvidia, which makes chips, only showed a hardware product?"
This made Huang Renxun, who was dressed exactly the same as last year on stage, look hardcore but not sexy enough.
What's more, in the company's just released Q3 quarterly financial report, the data from the automotive business is not good. Although the media friends generally kept their mouths shut during the concentrated interviews, it still could not cover up the decline in performance caused by the overall weakness of the automotive industry: public data showed that Nvidia's automotive business stopped growing in Q3 2019 after continuing the growth momentum in the first seven quarters, with a year-on-year decline of 6%.
You should know that the company's automotive business has seen a year-on-year increase of 63% in the past three years. At a time when automakers are slowing down their investment in automotive electronics and autonomous driving due to cost cuts, a report from JD Power Survey shows that by 2034, the budget for this aspect is expected to account for only about 10% of the overall auto sales share.
Is Nvidia, the biggest beneficiary of the explosive growth in automotive electronics, about to bid farewell to the century-old automotive industry?
Computing power = hardcore?
From a technical perspective, NVIDIA has always insisted that its own GPU is the perfect architecture for deep learning.
(“With the end of Moore’s Law, it is now generally accepted that GPU-accelerated computing will be the future.”
“Great chips are just the starting point.”)
Having said that, perhaps due to the power consumption problem after the chip is "installed on the car", Nvidia plans to build its own CPU architecture from scratch based on the ARM architecture license. Future products will also be gradually iterated from suppliers to self-research.
After all, when Tesla's FSD chip was first released, Nvidia brought out the 320 TOPS of the AGX Pegasus dual chip to compete. But the more realistic problem is that compared with the FSD chip, which consumes about 200W of power, the layout of Nvidia's two chips consumes up to 500W of power. Some media calculated that this is equivalent to an electric car consuming half a kilowatt-hour more per hour.
This provides a good answer to our question: For autonomous driving chips, does strong computing power mean super hardcore?
The answer is obviously no. Computing power cannot be used as the only indicator to measure the performance of AI chips. In practical use, in the case of multi-service switching, especially when measuring the performance of node-side chips and cloud-side chips, the performance of the computing core should theoretically be regarded as an important criterion.
In addition, AI acceleration hardware is also very dependent on memory bandwidth. Therefore, under the same algorithm and computing workload, the efficiency of bandwidth usage by the computing core also determines the overall performance of the system. According to reports, Nvidia's own NV Link 2.0 multi-chip data transmission standard can currently provide a bandwidth of 100GB/s, while Tesla has a 2TB bandwidth SRAM.
In other words, the Nvidia AGX Pegasus with 320 TOPS computing power is of no value to Tesla. After all, the latter is struggling to reduce the operating costs of the Robotaxi project for fully autonomous vehicles. Musk also directly said: "The key is how many TOPS in the chip can actually be used for image processing and behavior prediction related to autonomous driving?"
Compared with Tesla's FSD chip, which is tailor-made for itself and has precisely controlled various indicators, Nvidia's voice in the competition is obviously much weaker. To some extent, Tesla represents the real needs of most OEMs in the market.
After all, there are too many dedicated chips for fixed functions in the automotive industry. They are small in size, low in cost, and low in power consumption, and these characteristics are almost exactly what car manufacturers want.
But in Nvidia's eyes, this is synonymous with "low programmability". Dedicated chips cannot handle the complex workload required for advanced high-level autonomous driving, which includes diversified data processing required by the fusion of more than ten cameras, millimeter-wave radars, lidars and other sensors, and leaves enough safety redundancy, which requires at least several hundred TOPS of computing power.
"When it comes to deep learning training through neural networks, there are only two artificial intelligence supercomputers. One is from Tesla and the other is from Nvidia," said Danny Shapiro, senior director of Nvidia's automotive business unit.
According to him, NVIDIA is the only company that has submitted test applications for all categories and ranked first in all categories. Its full software stack can support all mainstream AI frameworks. You know, in order to achieve autonomous driving, in addition to software and hardware, a lot of pre-processing and post-processing work is also required.
Taking the "new face" Orin as an example, its unique design lies in the use of multiple processors, including GPU, ARM architecture CPU, programmable processor, and codec. Since both Orin and Xavier can be programmed through open CUDA, TensorRT API and various libraries, developers can use products across multiple generations after a one-time investment.
Obviously, compared with OEMs that strictly control costs and deliver immediately after mass production, Nvidia's current customer base is probably more focused on technology companies dedicated to high-level autonomous driving. The latter can only maximize Nvidia's value by continuously completing software iterations on the same development board.
Huawei is also trying to reach the same wave of customers. Just four months ago, Xu Zhijun released two AI chips, Ascend 910 and Ascend 310, under the big screen of "The Strongest Chip on Earth". Even the MDC in-vehicle technology unit launched on this basis also pointed to Nvidia's monopoly in the chip industry.
According to the description at the time, the Ascend 910 has a computing power of 256 TOPS, while the Ascend 310 has a weaker computing power, but it takes into account power consumption and is more suitable for self-driving cars. "The Ascend 910 is benchmarked against Google and Nvidia's AI computing chips for training AI models. The specific pricing has not yet been determined, but it will definitely be lower than Nvidia and Google." Xu Zhijun said with a smile.
Ecology: Business Acumen
In fact, what Xu Zhijun wants to target is not just computing power, but Nvidia’s highest moat.
In Q1 2020, MindSpore will be officially open source and "build its own ecosystem like NVIDIA."
This explains why Huang Renxun only brought one hardware product but was still not in a hurry. Within the company, the number of software developers has far exceeded the number of hardware engineers. Danny Shapiro also mentioned that the significance of NVIDIA Drive system is to provide customers with pre-trained models, thereby opening up the ecosystem to join the GPU cloud.
By 2019, Nvidia, which has sold 1.5 billion GPUs, no longer needs to hide its ambitions. Every GPU in use on the market is compatible with CUDA, and behind this platform are rich libraries, tools, and applications. In 2018, Nvidia released more than 500 SDKs and libraries, improving GPU performance by optimizing the software stack, allowing deep learning training to increase fourfold in three years and deep learning reasoning to increase twofold in one year.
Today, NVIDIA has once again decided to open source NVIDIA DRIVE deep neural networks for autonomous vehicle development to the transportation industry.
In other words, with NVIDIA's open source pre-trained AI models and training codes, as long as autonomous driving car developers are willing to join this ecosystem, they can freely expand and customize models through a set of NVIDIA AI tools to improve the robustness and capabilities of their autonomous driving systems.
This product runs on the deep neural network core of the DRIVE AGX platform and is composed of dozens of deep neural networks that can handle redundant and different tasks to ensure accurate perception, positioning and path planning, and complete tasks such as traffic light and traffic sign detection, target detection (vehicles, pedestrians, bicycles), path perception, and on-board eye tracking and gesture recognition.
In addition to open sourcing deep neural networks, NVIDIA has also released a set of advanced tools that allow developers to customize and enhance NVIDIA's deep neural networks with their own datasets and target feature sets. This set of tools uses active learning, federated learning, and transfer learning to train deep neural networks.
The popularity of Nvidia products among startups in the past may not have been convincing, but the fact that internet giant Didi Chuxing has bought into them says it all.
Previous article:Neptune has landed! Nvidia releases autonomous driving chip Orin
Next article:Nexeon obtains key silicon anode patent to enhance or replace traditional graphite anode with silicon
Recommended ReadingLatest update time:2024-11-15 08:50
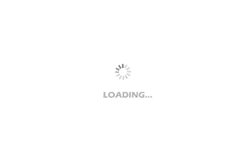
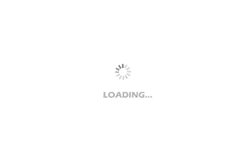
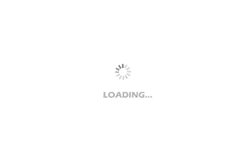
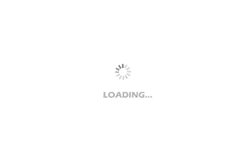
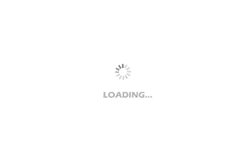
- Popular Resources
- Popular amplifiers
-
Semantic Segmentation for Autonomous Driving: Model Evaluation, Dataset Generation, Viewpoint Comparison, and Real-time Performance
-
A Practical Tutorial on ASIC Design (Compiled by Yu Xiqing)
-
Design and application of autonomous driving system (Yu Guizhen, Zhou Bin, Wang Yang, Zhou Yiwei)
-
ASPEN: High-throughput LoRA fine-tuning of large language models using a single GPU
- A new chapter in Great Wall Motors R&D: solid-state battery technology leads the future
- Naxin Micro provides full-scenario GaN driver IC solutions
- Interpreting Huawei’s new solid-state battery patent, will it challenge CATL in 2030?
- Are pure electric/plug-in hybrid vehicles going crazy? A Chinese company has launched the world's first -40℃ dischargeable hybrid battery that is not afraid of cold
- How much do you know about intelligent driving domain control: low-end and mid-end models are accelerating their introduction, with integrated driving and parking solutions accounting for the majority
- Foresight Launches Six Advanced Stereo Sensor Suite to Revolutionize Industrial and Automotive 3D Perception
- OPTIMA launches new ORANGETOP QH6 lithium battery to adapt to extreme temperature conditions
- Allegro MicroSystems Introduces Advanced Magnetic and Inductive Position Sensing Solutions
- TDK launches second generation 6-axis IMU for automotive safety applications
- LED chemical incompatibility test to see which chemicals LEDs can be used with
- Application of ARM9 hardware coprocessor on WinCE embedded motherboard
- What are the key points for selecting rotor flowmeter?
- LM317 high power charger circuit
- A brief analysis of Embest's application and development of embedded medical devices
- Single-phase RC protection circuit
- stm32 PVD programmable voltage monitor
- Introduction and measurement of edge trigger and level trigger of 51 single chip microcomputer
- Improved design of Linux system software shell protection technology
- What to do if the ABB robot protection device stops
- Detailed explanation of intelligent car body perception system
- How to solve the problem that the servo drive is not enabled
- Why does the servo drive not power on?
- What point should I connect to when the servo is turned on?
- How to turn on the internal enable of Panasonic servo drive?
- What is the rigidity setting of Panasonic servo drive?
- How to change the inertia ratio of Panasonic servo drive
- What is the inertia ratio of the servo motor?
- Is it better for the motor to have a large or small moment of inertia?
- What is the difference between low inertia and high inertia of servo motors?
- What is the special purpose of inductors wound with silk wire?
- Domestic 51 single chip microcomputer
- When using a transistor in the amplification area, which thermal resistance should be considered after considering the power?
- Design of breathing light based on FPGA
- TMS320C6678 device configuration pins and power-on timing
- Does anyone know what the diode in the picture does?
- Op amp selection
- Have you replaced your instrument with a touch screen today?
- [Event Posting Summary] Prize-giving Event: Show off your electronic collection
- Enable TI 15.4-Stack to support 470M frequency band