2. Simulation verification of sensor functional model
In this paper, three sensor models are established respectively with reference to the parameters of Delphi ESR millimeter-wave radar, Ibeo four-line lidar and Delphi IFV300 monocular camera, and the models are simulated and verified as follows.
2.1 Millimeter-wave radar model
2.1.1 Functional Verification
The ESR millimeter wave radar model can sense various moving and stationary obstacles and represent the sensing results as particles. The millimeter wave functional model simulates the millimeter wave radar's perception of the radial relative distance, radial relative speed, and angle of the target.
Figure 5 shows the perception results of the millimeter-wave radar model in a complex traffic environment. The model detects both vehicles and stationary obstacles and identifies the vehicle in front as an ACC tracking target. In addition, this verification is an example of a picture after adding Gaussian white noise. The rest of the unmarked ones are noise points at that moment.
Figure 5 (a) is a screenshot of the virtual scene, which includes vehicles and roadside lampposts, and Figure 5 (b) shows the short-range and long-range millimeter-wave radar perception results. As can be seen from the figure, the millimeter-wave radar can detect the vehicle and lamppost in front, and mark the four vehicles in front of the lane as ACC tracking targets, while characterizing the occlusion relationship between vehicles. At this time, the main vehicle speed is 30 km/h and the target vehicle speed is 50 km/h.
Figure 6 shows a schematic diagram of the millimeter-wave radar model curve detection process. Figures 6 (a) and 6 (b) show the detection results when the oncoming vehicle has not entered the detection range of the main vehicle's millimeter-wave radar, and Figures 6 (c) and 6 (d) show the detection results when the oncoming vehicle enters the detection range of the main vehicle's millimeter-wave radar. In this figure, the detection display of the street light pole is turned off. At this time, the main vehicle speed is 0 and the target vehicle speed is 50 km/h.
Figure 7 shows the detection results of the millimeter wave radar when the front vehicle is changing lanes. Figure 7 (a) and Figure 7 (b) show the detection results when the front vehicle is changing lanes. At this time, vehicle 2 is changing lanes and blocking vehicles 3 and 4.
Figure 7 (c) and Figure 7 (d) show the detection results after the front vehicle has completed lane change. At this time, vehicle 2 has completed lane change and is in the same lane as vehicles 3 and 4. The unmarked points are lamp posts. At this time, the main vehicle speed is 0 and the target vehicle speed is 50 km/h.
2.1.2 Performance Verification
In order to verify the accuracy of millimeter-wave radar perception, a stationary vehicle with a length of 4.2m and a width of 1.8m was placed at 14.9, 34.9, 54.9, 84.9, 124.9 and 164.9m in front of the vehicle, with an angle of -0.2757°. The detection results of the millimeter-wave radar model are shown in Figure 8.
The ideal distance variance and ideal angle variance are both set to σ = 0.5/3 = 0.1667, the actual detection distance variance average is 0.1663, and the actual detection angle variance average is 0.1582. It can be seen that the millimeter wave radar established in this paper can simulate and detect obstacles more accurately, and the detection accuracy is high.
2.2 LiDAR Model
The lidar model established in this paper can sense various moving and stationary obstacles, calculate their geometric contours, estimate classification, and simulate the lidar's perception process of the target's relative position, speed, and geometric contour.
2.2.1 Functional Verification
Figure 9 shows the effective perception results of the lidar model on the surrounding vehicles in a complex traffic environment. The model simulates the occlusion effect and can only perceive nearby vehicles. Moreover, the geometric contours of the perceived vehicles are not the same. Vehicles that are close and located on both sides of the main vehicle can return a length that is closer to the actual value, while vehicles that are far away and located directly in front of the main vehicle can only return the width, and the length value is very small.
Figure 10 shows the detection results of the laser radar when the front car is blocked. Figure 10 (a) and Figure 10 (b) show that the front car 1 blocks the front car 2, making the position of the front car 2 detected by the laser radar
The length is less than the set threshold, so the two cars are not visible.
Figure 10 (c) and Figure 10 (d) show that car 1 in front continues to travel at a higher speed than car 2. At this time, the part of car 2 blocked by car 1 is decreasing. The part that can be detected by the laser radar is larger than the set threshold, but its side is not detected. Therefore, only the body width of car 2 is displayed but not the body length.
2.2.2 Performance Verification
To verify the perception accuracy of the laser radar, the main vehicle is placed at the origin, the target vehicle coordinates: X = 80m, Y = 0, Z = 0, specifications: 4.2m × 1.8m, radar installation position: X = 0, Y = 0, Z = 0, and the laser radar model detection data is shown in Table 1. As can be seen from Table 1, when the laser radar simulation established in this paper detects obstacles, the distance detection value and width detection value are highly consistent with the ideal value.
2.3 Camera Model
The camera model developed in this paper simulates the camera's perception of the vehicle's relative position, longitudinal speed, width, type, and lane markings.
2.3.1 Functional Verification
The camera model established in this paper can perceive the lane markings on the road (set to 4 in this paper, two on the left and right of the main vehicle), and is suitable for road conditions such as curves and ramps. The overall perception result is shown in Figure 11.
2.3.2 Performance Verification
In the case of noise, the target vehicle is 4.2m×1.8m, the camera installation position is X=+3m, Y=0, Z=0, the longitudinal distance detection accuracy is 2m, σxexep=2/3=0.6667, the lateral distance detection accuracy is 0.5m, σyexep=0.5/3=0.1667. The target width detection accuracy is 0.5m. The camera model detection data results are shown in Table 2.
It can be seen from Table 2 that when the camera model established in this paper simulates obstacle detection, the actual detection distance and width values are basically consistent with the ideal values, and the actual distance variance and width variance also deviate slightly from the ideal values.
3. Model calculation efficiency verification and conclusion
The sensor model established in this paper improves computing efficiency while meeting real-time computing requirements, and can be used for multiple smart cars in simulation scenarios.
To test the three sensor models established in this paper, 10 sensor model instances with exactly the same parameters are run simultaneously for each sensor on an ordinary PC. The average time taken for each sensor model instance to run 10,000 times is counted. The results are shown in Table 3.
The computer processor model is Intel (R) Core (TM) i7-4790, with a main frequency of 3.6 GHz. The simulation results show that the sensor function model established in this paper not only reflects the necessary physical characteristics of the sensor, but also has a very high computational efficiency, with a time of less than 0.2 ms, and supports concurrent operation, creating conditions for concurrent simulation of multiple intelligent vehicles on a virtual test platform.
in conclusion
Based on the above modeling framework and key methods, and referring to the parameters of vehicle-mounted sensor products, the millimeter-wave radar, lidar, and camera models established in this paper can simulate physical characteristics such as occlusion between objects and sensor perception errors. Dozens of sensor models can be run concurrently on a computer, and the calculation cycle of each model is at the sub-millisecond level, which can support real-time simulation of complex test scenarios involving multiple intelligent vehicles at the same time.
Previous article:The development environment and future market space of Internet of Vehicles
Next article:BMW is developing new forms of lithium batteries, but internal combustion engines will remain the mainstream product for a short time
Recommended ReadingLatest update time:2024-11-15 21:57
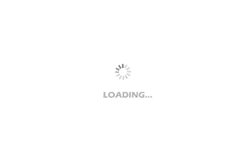
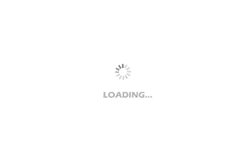
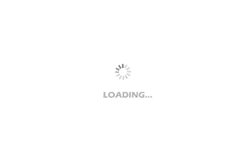
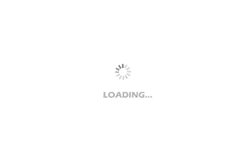
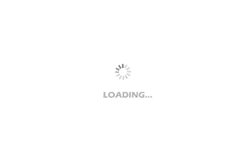
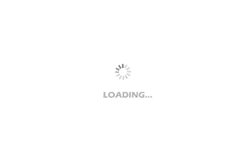
- Popular Resources
- Popular amplifiers
-
LiDAR point cloud tracking method based on 3D sparse convolutional structure and spatial...
-
GenMM - Geometrically and temporally consistent multimodal data generation from video and LiDAR
-
Comparative Study on 3D Object Detection Frameworks Based on LiDAR Data and Sensor Fusion Technology
-
A review of deep learning applications in traffic safety analysis
- Huawei's Strategic Department Director Gai Gang: The cumulative installed base of open source Euler operating system exceeds 10 million sets
- Analysis of the application of several common contact parts in high-voltage connectors of new energy vehicles
- Wiring harness durability test and contact voltage drop test method
- Sn-doped CuO nanostructure-based ethanol gas sensor for real-time drunk driving detection in vehicles
- Design considerations for automotive battery wiring harness
- Do you know all the various motors commonly used in automotive electronics?
- What are the functions of the Internet of Vehicles? What are the uses and benefits of the Internet of Vehicles?
- Power Inverter - A critical safety system for electric vehicles
- Analysis of the information security mechanism of AUTOSAR, the automotive embedded software framework
Professor at Beihang University, dedicated to promoting microcontrollers and embedded systems for over 20 years.
- LED chemical incompatibility test to see which chemicals LEDs can be used with
- Application of ARM9 hardware coprocessor on WinCE embedded motherboard
- What are the key points for selecting rotor flowmeter?
- LM317 high power charger circuit
- A brief analysis of Embest's application and development of embedded medical devices
- Single-phase RC protection circuit
- stm32 PVD programmable voltage monitor
- Introduction and measurement of edge trigger and level trigger of 51 single chip microcomputer
- Improved design of Linux system software shell protection technology
- What to do if the ABB robot protection device stops
- Allegro MicroSystems Introduces Advanced Magnetic and Inductive Position Sensing Solutions at Electronica 2024
- Car key in the left hand, liveness detection radar in the right hand, UWB is imperative for cars!
- After a decade of rapid development, domestic CIS has entered the market
- Aegis Dagger Battery + Thor EM-i Super Hybrid, Geely New Energy has thrown out two "king bombs"
- A brief discussion on functional safety - fault, error, and failure
- In the smart car 2.0 cycle, these core industry chains are facing major opportunities!
- The United States and Japan are developing new batteries. CATL faces challenges? How should China's new energy battery industry respond?
- Murata launches high-precision 6-axis inertial sensor for automobiles
- Ford patents pre-charge alarm to help save costs and respond to emergencies
- New real-time microcontroller system from Texas Instruments enables smarter processing in automotive and industrial applications
- China's fast charging standard released! Download and study
- Anqu David starts updating: motor drive development learning and communication, starting from the system and basics
- [Top Micro Intelligent Display Module Review] 9. Touch screen input value (PIP keyboard application)
- A brief discussion on the "layered thinking" in single-chip computer programming
- 【AT32F421 Review】+ ADC Data Collection and Display
- High-definition pictures to understand the high-end precision HDI board PCB architecture
- [Perf-V Evaluation] Build and apply Hummingbird open source SOC based on Perf-V development board
- 【GD32307E-START】03- Schematic diagram of expansion board
- Classification of DSP processors
- When BLE meets MEMS——Device Selection