Recently, the concept of "world model" was mentioned at the launch conferences of NIO IN and Ideal Intelligent Driving System.
Ren Shaoqing, vice president of intelligent driving R&D at NIO, believes that only the traditional end-to-end solution + "world model" can advance autonomous driving to the next stage - end-to-end is not the end of the autonomous driving technology route.
Ideal also believes that data-driven end-to-end can only achieve L3. To continue moving towards L4, it requires knowledge-driven visual language model/world model.
In fact, He Xiaopeng also put forward a similar view that end-to-end is "the best route for L3, but it is definitely not the preferred choice for L4. Only end-to-end + big model can ultimately achieve L4."
After more than half a year of exploration and initial practice, local players have released or mass-produced their own end-to-end solutions, and the value of the latter has been unanimously recognized by the autonomous driving industry. But it is not the end of the universe.
So, what exactly is the next “world model”? What stage has it reached? A more worthy question is, is it the optimal solution for the intelligent driving big model?
The Origin of the “World Model”
Tesla
At the 2023 CVPR conference (the IEEE International Conference on Computer Vision and Pattern Recognition), Tesla's head of autonomous driving, Ashok Elluswamy, spent 15 minutes introducing the FSD Foundation Model, which mainly focuses on lane networks and occupancy networks.
Ashok raised a thought-provoking question: Is it enough to combine lane networks and occupancy networks to fully describe the driving scenario? Can we plan safe, efficient and comfortable trajectories based on these scenario descriptions?
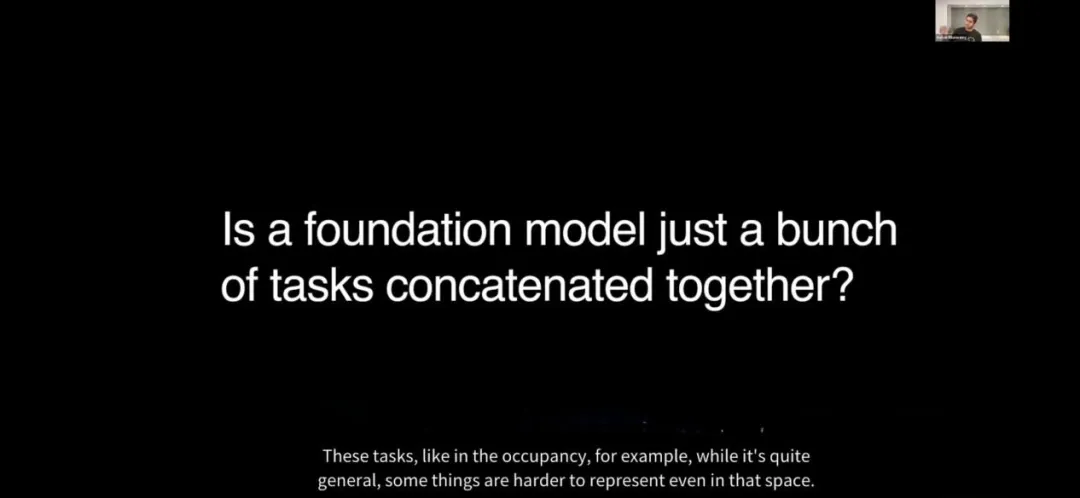
Image source: Tesla
The answer is of course no.
Because the granularity of OCC space is not fine enough at the spatial level, the algorithm cannot detect obstacles smaller than the grid unit size, and does not include semantic information such as weather, lighting, and road conditions that have a close impact on driving safety and comfort. At the temporal level, the planning algorithm performs information fusion and deduction at a fixed time interval, so the ability to automatically model long-term information is relatively scarce, making it difficult to accurately predict scene changes that are critical to car driving safety and efficiency in the future based on current scenes and vehicle actions.
what to do?
Tesla's answer is that it uses massive amounts of data to learn a neural network that can "predict the future based on the past or other inputs."
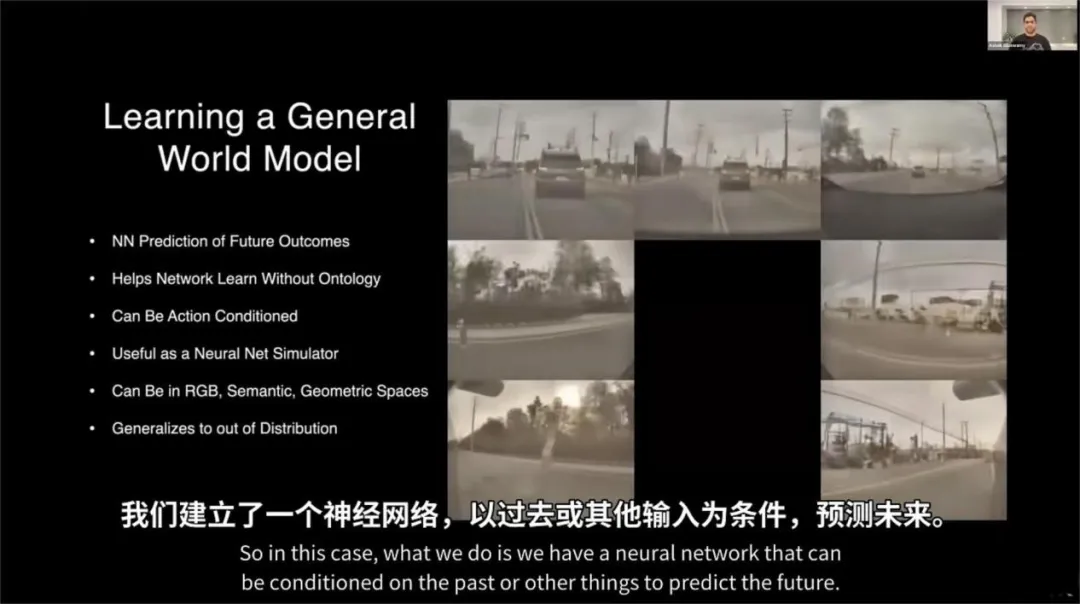
Image source: Tesla
Yes, the "world model" was formally proposed by Tesla a year ago.
However, Tesla, which no longer holds AI Days for fear of being learned frame by frame by its competitors, insists on maintaining a mysterious and vague style on the "world model", and only summarizes it with a philosophical phrase "predicting the future based on the past", making people feel like they are listening to its words.
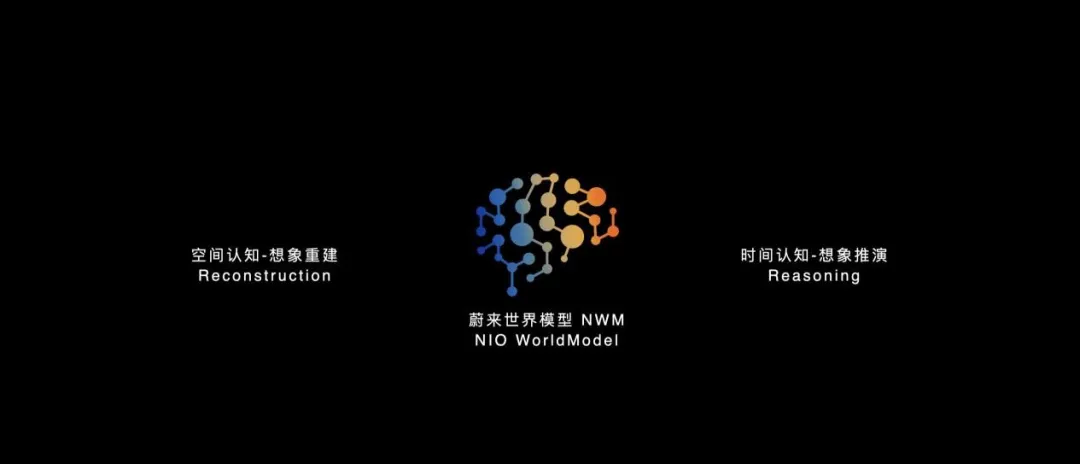
Image source: NIO
NIO made this concept clearer and more specific when explaining its world model NVM. In summary, its two core capabilities are spatial cognition and temporal cognition.
Spatial cognitive ability can understand the laws of physics and carry out imaginative reconstruction; temporal cognitive ability can generate future scenes that conform to the laws of physics and carry out imaginative deduction.
Therefore, in terms of spatial understanding capabilities, NVM, as a generative model, can fully understand the data, reconstruct the scene from the original sensor data, and reduce the information loss in the traditional end-to-end solution during the conversion from sensor data to BEV and OCC feature space.
In terms of time understanding, NVM has the ability to deduce and make decisions over long time series, and can automatically model long time series environments through autoregressive models, giving it stronger predictive capabilities.
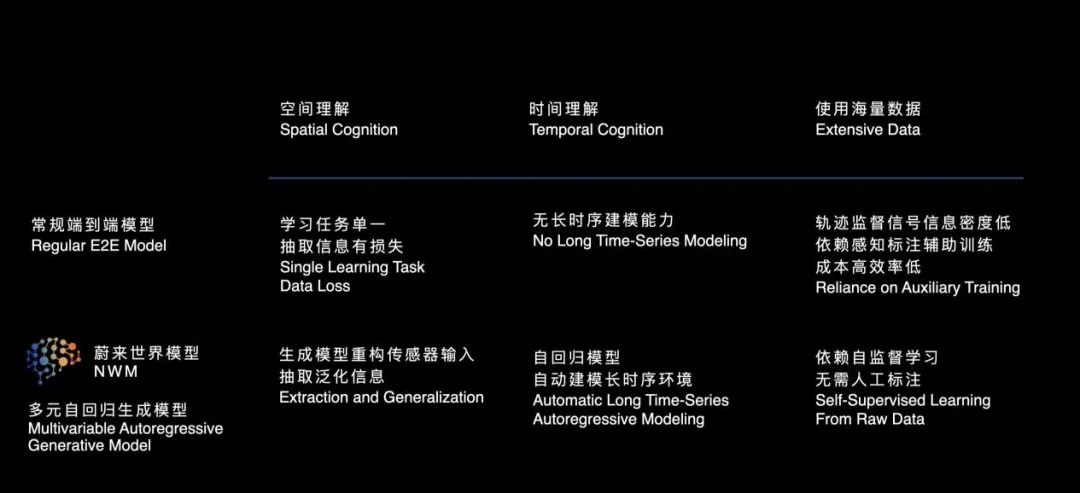
Image source: NIO
In layman's terms, in terms of spatial understanding, the "world model" adopts a generative model architecture, which naturally has the ability to fully extract sensor input information. It can extract generalized information closely related to driving, such as rainy, snowy, windy and frosty weather, dark light, backlight and glare lighting conditions, snow, potholes and bumpy road conditions, avoiding the loss of information extracted by BEV and occupied networks.
In terms of time understanding, the "world model" is an autoregressive model. The next frame of video (at time t+0.1) can be generated from the current (at time t) video and vehicle action, and then the next frame of video (at time t+0.2) can be generated based on the next frame of video (t+0.1) and the action at that time. This cycle repeats itself, through a deep understanding and simulation of future scenarios, the planning and decision-making system performs deductions in possible scenarios and finds the optimal path that maximizes the three elements of safety, comfort, and efficiency.
What stage has the “world model” reached?
In fact, the prototype concept of "world model" can be traced back to 1989. However, since it is deeply tied to the development history of artificial intelligence and neural networks, it is too long-winded to talk about it, so there is no need to extend the time so far.
We fast forward to February 2024, when OpenAI released Sora, a powerful machine learning algorithm that can generate long-term and highly consistent videos, which has caused a lot of controversy.
Supporters believe that Sora has the ability to understand the laws of the physical world, which marks the beginning of OpenAI's capabilities moving from the digital world to the physical world, and from digital intelligence to spatial intelligence.
Opponents, represented by Yang Likun, believe that Sora only conforms to "intuitive physics". The videos it generates can deceive the human eye, but it cannot generate videos that are highly consistent with the robot's sensors. Only the world model has the ability to truly understand the laws of physics, reconstruct and deduce the external world.
Musk, who eventually broke up with OpenAI because he did not get control of OpenAI, certainly would not miss this big debate. He proudly stated that Tesla was able to generate real-world videos with precise physical laws about a year ago. Moreover, Tesla's video generation ability far exceeds OpenAI because it can predict extremely accurate physical properties, which is crucial for autonomous driving.
According to Musk's speech and the introduction at the 2023 CVPR conference, Tesla's "world model" can generate driving scenarios for model training and simulation in the cloud. More importantly, it can also be compressed and deployed on the vehicle side, upgrading the FSD basic model running on the vehicle side to a world model.
Combined with the news that Tesla will release Robotaxi in October, which theoretically should have L4 capabilities, and the important judgment of domestic automotive industry leaders that end-to-end + big models are the only way to achieve L4, Tesla's world model is likely to have been deployed in mass production on the vehicle side.
However, the world models trained by most domestic autonomous driving players are still deployed in the cloud and are used for the generation of autonomous driving simulation scenarios.
For example, the ideal world model uses a 3D Gaussian model for scene reconstruction and a diffusion model for scene generation, combining reconstruction and generation to form the test plan for the Ideal Auto autonomous driving system.
Huawei and Xiaopeng are exploring the use of large models to generate simulation scenarios, which is also in line with the concept of the autonomous driving world model.
However, none of the three companies have disclosed specific figures on the temporal consistency of the scenes generated by their world models, nor how long they last.
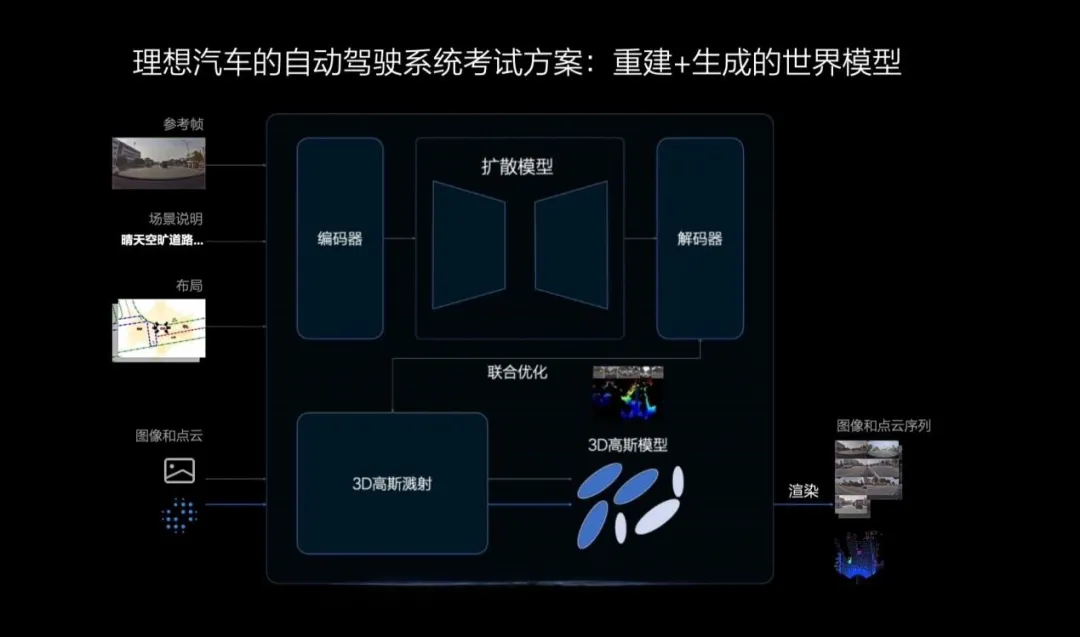
Image source: Ideal Auto
NIO has chosen the technical path of simultaneously tackling the cloud and vehicle sides.
In the cloud, Weilai's Nsim can deduce thousands of parallel worlds and assist in accelerating the training of NVM with real data. Currently, NVM can generate predictions up to 120 seconds long. In comparison, OpenAI's much-hyped Sora can only generate 60 seconds of video.
And unlike Sora which only has a simple camera movement, the scenes produced by NIO's NVM are richer and more varied. It can give multiple command actions and deduce thousands of parallel worlds.
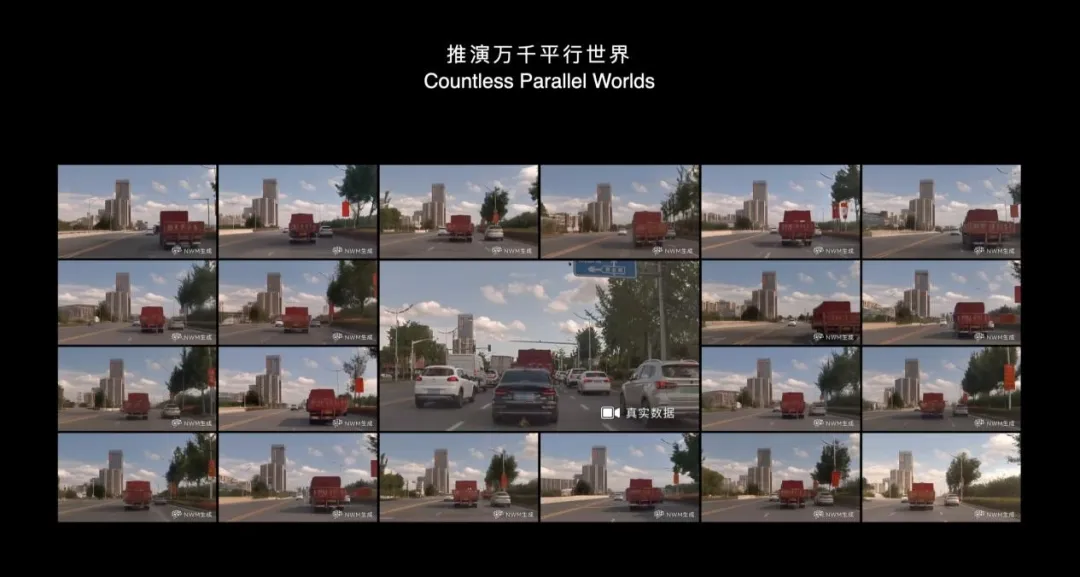
Image source: NIO
On the vehicle side, NIO NVM can deduce 216 parallel worlds under different trajectories within 0.1 seconds and select the optimal path. Then, in the next 0.1 second window, it will re-update the internal space-time model based on the external world’s input, predict 216 possible trajectories, and repeat the cycle, continuously predicting along the driving trajectory, and always selecting the optimal solution.
Where is the optimal solution for the intelligent driving big model?
Let's go back to the point that empowering end-to-end through big models has become a consensus to continue to improve the capabilities of intelligent driving systems. However, leading intelligent driving companies NIO, Li Auto and Xpeng have given three different answers on how to deploy big models on the vehicle side.
Previous article:Tesla V4 Supercharger is being tested for higher charging power
Next article:What exactly is “end-to-end” autonomous driving, and which car companies have “end-to-end” capabilities?
- Popular Resources
- Popular amplifiers
- A new chapter in Great Wall Motors R&D: solid-state battery technology leads the future
- Naxin Micro provides full-scenario GaN driver IC solutions
- Interpreting Huawei’s new solid-state battery patent, will it challenge CATL in 2030?
- Are pure electric/plug-in hybrid vehicles going crazy? A Chinese company has launched the world's first -40℃ dischargeable hybrid battery that is not afraid of cold
- How much do you know about intelligent driving domain control: low-end and mid-end models are accelerating their introduction, with integrated driving and parking solutions accounting for the majority
- Foresight Launches Six Advanced Stereo Sensor Suite to Revolutionize Industrial and Automotive 3D Perception
- OPTIMA launches new ORANGETOP QH6 lithium battery to adapt to extreme temperature conditions
- Allegro MicroSystems Introduces Advanced Magnetic and Inductive Position Sensing Solutions
- TDK launches second generation 6-axis IMU for automotive safety applications
- LED chemical incompatibility test to see which chemicals LEDs can be used with
- Application of ARM9 hardware coprocessor on WinCE embedded motherboard
- What are the key points for selecting rotor flowmeter?
- LM317 high power charger circuit
- A brief analysis of Embest's application and development of embedded medical devices
- Single-phase RC protection circuit
- stm32 PVD programmable voltage monitor
- Introduction and measurement of edge trigger and level trigger of 51 single chip microcomputer
- Improved design of Linux system software shell protection technology
- What to do if the ABB robot protection device stops
- ASML predicts that its revenue in 2030 will exceed 457 billion yuan! Gross profit margin 56-60%
- Detailed explanation of intelligent car body perception system
- How to solve the problem that the servo drive is not enabled
- Why does the servo drive not power on?
- What point should I connect to when the servo is turned on?
- How to turn on the internal enable of Panasonic servo drive?
- What is the rigidity setting of Panasonic servo drive?
- How to change the inertia ratio of Panasonic servo drive
- What is the inertia ratio of the servo motor?
- Is it better for the motor to have a large or small moment of inertia?
- Semiconductor Device Physics (Shi Min)
- [Analysis of the topic of the college electronic competition] - 2019 National Competition G "Wireless transceiver system for dual-channel voice simultaneous interpretation"
- USB Type-c charging and music listening two-in-one adapter solution
- Prize-winning quiz | Xuanzhi Technology—High-performance, highly integrated motor control solutions
- Thank you + thank you EEWORLD community
- Medical electronics popular data download collection
- Tailing Micro B91 Development Kit --- Testing Power Consumption in BLE Connections
- Application areas of Bluetooth Low Energy
- During the fight against the epidemic, we will teach you how to have instruments in your mind without having any in your hands.
- Migrating between TMS320F28004x and TMS320F28002x