In recent days, the COVID-19 outbreak has escalated again in Beijing, making the overall positive domestic anti-epidemic situation severe. The production and life that had just restarted have been disrupted again. We may have to face the reality of normalization of epidemic prevention for a long time in the future. This week, I started to switch to the state of "cloud office, cloud life" again. Remote office and video conferencing have become habits. Children's schoolwork has been handed over to online education platforms, and the way of consumption and entertainment has also become watching live broadcasts and listening to cloud concerts. In the special scenario of the epidemic, a series of new AI application needs have been fully activated, and AI has truly penetrated into every aspect of our daily life.
As a technology practitioner, I know that the explosion of these new AI applications is not a one-day effort. The process from quantitative change to qualitative change is the result of long-term scientific research layout and digital infrastructure construction. Aiming at long-term goals and values and persisting in long-term scientific research are the only certainties when we face uncertain events such as the "epidemic". This requires not only a forward-looking vision and layout, but also the determination and will not to be shaken by immediate interests.
From the perspective of the development of AI, human exploration of AI has lasted for more than 70 years. Looking back at the development of AI, we can clearly capture several key nodes. The first wave of AI was to make theoretical inferences through various rules formulated by humans. Although it performed well in reasoning, it was limited to a few strictly defined problems, and it had no learning ability and could not handle uncertainty problems. What really made AI gradually better was the second wave of AI triggered by deep learning. The massive data generated by the Internet, mobile Internet, etc., provided machines with objects for learning, mining and trial and error, allowing the system to spontaneously find "rules" and make predictions, judgments and decisions. The growth of data, the increase in computing power and the evolution of algorithms based on deep learning, these three "trump cards" have enabled some typical deep learning applications to reach or even exceed human capabilities. This has convinced more and more optimists that deep learning is a direction that is extremely valuable and worthy of large-scale follow-up by the industry.
However, is deep learning the ultimate answer to AI? As the research on deep learning deepens, we find that there are still some problems that need to be solved. First, "energy consumption" is the biggest challenge. A research report shows that the carbon emissions generated by the electricity consumed by using a server-level CPU plus a GPU cluster to train a large AI model is equivalent to the carbon emissions consumed by 5 American cars in their entire life cycle. Imagine if all walks of life continue to use such an AI computing model, how much damage will be done to the human ecological environment. Then, "data volume" is another big challenge. Current deep learning relies too much on big data. In some scenarios with small data volumes, the use of deep learning will be very limited. AI should learn from small data like the human brain. During the training process, how to significantly reduce energy consumption and reduce the time and amount of data required while ensuring the capabilities of the AI model? This is an important direction for AI to continue to move forward. But now it seems that the method of accelerating deep learning training based on large-scale GPU parallel computing cannot meet this condition.
A truly intelligent system should be an environment-adaptive "natural intelligence". First, it can handle not only deterministic problems, but also uncertain problems. Second, it must not only be able to do things, but also be explainable. Third, it does not rely entirely on big data, and even a small amount of data can achieve more efficient continuous learning. Fourth, it should have high reliability, or conform to the ethics set by humans. This is our outlook on the next stage of development of AI technology - the AI 3.0 era.
At present, we are at the turning point from the AI 2.0 to the AI 3.0 era. So, what is expected to become the "sharp blade" that penetrates the future of AI? From the current perspective, as a cutting-edge computing model, neuromorphic computing is most likely to open up a new track from AI 2.0 to AI 3.0. Neuromorphic computing is an attempt and breakthrough in traditional semiconductor processes and chip architectures. By simulating the structure of human brain neurons and the mechanism of interconnection between neurons, it can continuously self-learn under conditions of low power consumption and a small amount of training data, greatly improving the energy efficiency ratio. Obviously, the characteristics of neuromorphic computing are very consistent with the development needs of AI3.0. Therefore, neuromorphic computing is also expected to play an important role in the process of human beings entering the next generation of AI.
Intel is a company that focuses on the long term and promotes innovation in underlying technologies to help customers achieve success in commercial applications. To this end, we continue to increase research in cutting-edge technology fields, even if these fields cannot see practical results in the short term. Focusing on neuromorphic computing, we have been actively exploring this new computing model from a very early stage and have achieved remarkable results. Intel's neuromorphic computing chip Loihi already has the ability to smell, and the neuromorphic system Pohoiki Springs already has the computing power of 100 million neurons, which is equivalent to the brain of a small mammal.
Of course, neuromorphic computing is still in its very early stages, and we still have a long way to go to truly apply this technology to AI. But I believe that innovation in underlying technologies must adhere to long-termism, focus on one direction and track for a long time, and use this certainty to fight against all uncertainties in the development process, in order to ultimately succeed.
Previous article:Advantech won the title of "Top 20 Edge Computing Companies in China in 2020"
Next article:Four Team USA athletes join Intel for the Olympics
Recommended ReadingLatest update time:2024-11-15 14:38
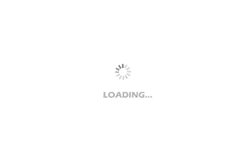
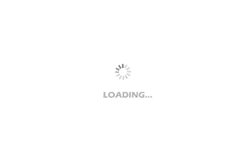
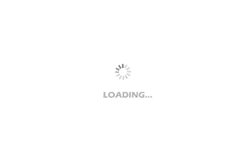
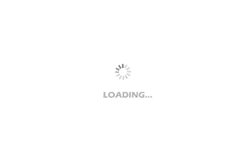
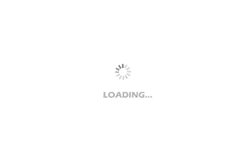
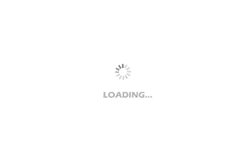
- Popular Resources
- Popular amplifiers
- e-Network Community and NXP launch Smart Space Building Automation Challenge
- The Internet of Things helps electric vehicle charging facilities move into the future
- Nordic Semiconductor Launches nRF54L15, nRF54L10 and nRF54L05 Next Generation Wireless SoCs
- Face detection based on camera capture video in OPENCV - Mir NXP i.MX93 development board
- The UK tests drones equipped with nervous systems: no need to frequently land for inspection
- The power of ultra-wideband: reshaping the automotive, mobile and industrial IoT experience
- STMicroelectronics launches highly adaptable and easy-to-connect dual-radio IoT module for metering and asset tracking applications
- This year, the number of IoT connections in my country is expected to exceed 3 billion
- Infineon Technologies SECORA™ Pay Bio Enhances Convenience and Trust in Contactless Biometric Payments
- LED chemical incompatibility test to see which chemicals LEDs can be used with
- Application of ARM9 hardware coprocessor on WinCE embedded motherboard
- What are the key points for selecting rotor flowmeter?
- LM317 high power charger circuit
- A brief analysis of Embest's application and development of embedded medical devices
- Single-phase RC protection circuit
- stm32 PVD programmable voltage monitor
- Introduction and measurement of edge trigger and level trigger of 51 single chip microcomputer
- Improved design of Linux system software shell protection technology
- What to do if the ABB robot protection device stops
- Analysis of the application of several common contact parts in high-voltage connectors of new energy vehicles
- Wiring harness durability test and contact voltage drop test method
- From probes to power supplies, Tektronix is leading the way in comprehensive innovation in power electronics testing
- From probes to power supplies, Tektronix is leading the way in comprehensive innovation in power electronics testing
- Sn-doped CuO nanostructure-based ethanol gas sensor for real-time drunk driving detection in vehicles
- Design considerations for automotive battery wiring harness
- Do you know all the various motors commonly used in automotive electronics?
- What are the functions of the Internet of Vehicles? What are the uses and benefits of the Internet of Vehicles?
- Power Inverter - A critical safety system for electric vehicles
- Analysis of the information security mechanism of AUTOSAR, the automotive embedded software framework
- [Help] What happens if PT100 is used at over-temperature?
- Design of frequency sweeper using digital frequency synthesis technology, FPGA and single chip microcomputer
- Bluetooth MESH technology makes up for the shortcomings of networking
- Some Problems with Differential Circuits
- Common base amplifier circuit problems
- Analog input
- [Sipeed LicheeRV 86 Panel Review] 10-Video Playback Test
- [Silicon Labs BG22-EK4108A Bluetooth Development Review] VII. WeChat applet controls LED and receives button status 2
- May Day event is online! Let's do a "labor" transformation of old things together!
- Design a PCB diagram based on FPGA electronic piano