As part of the transition from older to newer technologies in modern vehicles, inductive position sensors are designed to replace Hall-effect sensors, a shift that is essentially about better managing issues related to automotive sensor degradation.
For example, Microchip has introduced inductive position sensors for automotive applications such as automotive throttle bodies, transmission gear sensing, electronic power steering, and accelerator pedals. Position measurements need to be unaffected by stray magnetic fields and no external magnetic devices are required.
While engineers want to ensure that the sensor will operate over a wide range of temperatures, they worry about changes in the mechanical structure and degradation of the magnetic properties that could affect accuracy. But inductive position sensors use metal instead of a magnet, which does not degrade over time.
“It’s an important component to look at sensor degradation, whether it occurs inside the IC or outside,” said Mark Smith, senior marketing manager at Microchip. “When it comes to sensor degradation, the primary concern for engineers using inductive position sensors should be the longevity of the PCB.”
This is also important because sensor ICs serving automotive applications increasingly require ASIL certification. Microchip's inductive position sensors, the LX3301A, LX3302A and LX34050, are ASIL-B certified, allowing system designers to detect more than 90% of single-point failures.
Figure 1: The LX3302A inductive position sensor uses a larger EEPROM to facilitate eight calibration points to ensure sensor measurement accuracy.
Sensor degradation management
Currently, the industry is managing sensor degradation issues from scratch to meet ASIL certification. What if this transistor is broken, or that circuit fails? If the sensor output is shorted, what can engineers do? "It's a force majeure and time-consuming." Smith said.
Specific experiments must be performed to check or prove certain numbers, also known as coverage. Automotive engineers can create a fault and ensure it can be detected while using industry-standard reliability charts. "It's a relatively simple system that engineers can work on effectively," Smith added.
Today’s vehicles use approximately 50 position sensors, so the transition from Hall Effect sensors to inductive position sensors is critical in managing automotive sensor degradation. In addition to selecting sensors with materials that are less susceptible to degradation, what else can be considered in effectively managing sensor degradation in vehicles? Smith believes that machine learning is the way forward.
Smith said machine learning models can enable pattern recognition in automotive sensors before they fail. “Automotive engineers can analyze five different sensors and detect system-level failures as well as higher levels of degradation.”
Machine Learning is the Future
The automotive industry is starting to take sensor degradation seriously, but over time, there are ample opportunities to leverage machine learning for degradation-related analysis using some advanced computing techniques. However, the idea of using machine learning to manage vehicle sensor degradation is currently in its infancy and requires greater computing power.
Figure 2: Machine learning, taken up to the sensor level, can be used to create models to measure and mitigate automotive sensor degradation. Source: Mathworks
This approach enables engineers to collect large amounts of data, put it into a machine learning model, and then look for possible degradation. This is what is currently being done in the design of autonomous vehicles (AVs). "Machine learning is emerging at the sensor level, and it can be used to simplify the degradation measurement process and make the diagnostic process more efficient," Smith said.
The study of automotive sensor degradation is a natural fit for machine learning. The fact that machine learning takes large amounts of data and puts it into a model to detect sensor failures can lead to significant improvements in reliability and cost savings.
Previous article:Vishay Intertechnology Launches New Automotive-Grade Proximity Sensor with Pressure Sensing Resolution up to 20 µm
Next article:Ouster and CronAI collaborate to accelerate creation of 3D perception systems
Recommended ReadingLatest update time:2024-11-17 08:28
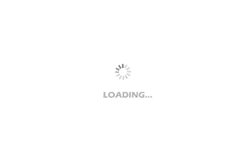
- Popular Resources
- Popular amplifiers
- Car key in the left hand, liveness detection radar in the right hand, UWB is imperative for cars!
- After a decade of rapid development, domestic CIS has entered the market
- Aegis Dagger Battery + Thor EM-i Super Hybrid, Geely New Energy has thrown out two "king bombs"
- A brief discussion on functional safety - fault, error, and failure
- In the smart car 2.0 cycle, these core industry chains are facing major opportunities!
- The United States and Japan are developing new batteries. CATL faces challenges? How should China's new energy battery industry respond?
- Murata launches high-precision 6-axis inertial sensor for automobiles
- Ford patents pre-charge alarm to help save costs and respond to emergencies
- New real-time microcontroller system from Texas Instruments enables smarter processing in automotive and industrial applications
- Innolux's intelligent steer-by-wire solution makes cars smarter and safer
- 8051 MCU - Parity Check
- How to efficiently balance the sensitivity of tactile sensing interfaces
- What should I do if the servo motor shakes? What causes the servo motor to shake quickly?
- 【Brushless Motor】Analysis of three-phase BLDC motor and sharing of two popular development boards
- Midea Industrial Technology's subsidiaries Clou Electronics and Hekang New Energy jointly appeared at the Munich Battery Energy Storage Exhibition and Solar Energy Exhibition
- Guoxin Sichen | Application of ferroelectric memory PB85RS2MC in power battery management, with a capacity of 2M
- Analysis of common faults of frequency converter
- In a head-on competition with Qualcomm, what kind of cockpit products has Intel come up with?
- Dalian Rongke's all-vanadium liquid flow battery energy storage equipment industrialization project has entered the sprint stage before production
- Allegro MicroSystems Introduces Advanced Magnetic and Inductive Position Sensing Solutions at Electronica 2024
- Car key in the left hand, liveness detection radar in the right hand, UWB is imperative for cars!
- After a decade of rapid development, domestic CIS has entered the market
- Aegis Dagger Battery + Thor EM-i Super Hybrid, Geely New Energy has thrown out two "king bombs"
- A brief discussion on functional safety - fault, error, and failure
- In the smart car 2.0 cycle, these core industry chains are facing major opportunities!
- Rambus Launches Industry's First HBM 4 Controller IP: What Are the Technical Details Behind It?
- The United States and Japan are developing new batteries. CATL faces challenges? How should China's new energy battery industry respond?
- Murata launches high-precision 6-axis inertial sensor for automobiles
- Ford patents pre-charge alarm to help save costs and respond to emergencies
- [Analysis of College Student Electronic Competition Topic] —— 2022 TI Cup Invitational Topic X "Lissajous Figure Demonstration Device"
- Effects of Differential Input Clamping on Operational Amplifiers
- ADI New Chinese Information
- Does anyone know what this board is used for?
- RISC-V core chip trial
- Two-way BUCK
- GD32 wireless thermostat receiver
- Passive RFID tag structure and principle
- MSP430 Classic Textbook Recommendations
- GaN Technology Then and Now