The development of autonomous driving is inseparable from data. Recently, Hesai Technology and Scale AI jointly released an open source dataset for autonomous driving - PandaSet. PandaSet uses Hesai Technology's advanced LiDAR for data collection and Scale AI's powerful annotation platform for accurate data annotation, providing companies, institutions and individuals engaged in autonomous driving research and development with high-quality free data that is rich in content and dense in objects.
Taking stock of the global artificial intelligence data platform, Scale AI is a well-deserved leader. This company, co-founded by Chinese youth Alexandr Wang at the age of 19, has been favored by investors since its establishment. In just three years, it has become a unicorn company with a market value of over 1 billion US dollars. Relying on strong technical strength, Scale AI combines manual annotation, intelligent tools and annotation quality assurance system to launch a series of annotation products for sensor data, images, videos and texts, providing first-class training and verification data for artificial intelligence applications. As the world's leading lidar manufacturer, Hesai Technology has always led the development direction of sensor innovation with its self-developed micro-vibration mirror and waveform encryption technology. It has currently deployed more than 400 patents and has customers in 70 cities in 21 countries and regions around the world. This time, Hesai Technology and Scale AI have joined hands to create the PandaSet open source data set, which has undoubtedly injected new vitality into the development of the autonomous driving industry.
In the development of autonomous driving, data is the core means of production, representing the core competitiveness of a company and determining whether autonomous driving can be safe and stable. In the past, autonomous driving "players" were generally sensitive about their own data. However, as the difficulty of autonomous driving became increasingly apparent, everyone gradually realized that going it alone would not work and open cooperation was the right way to go. Therefore, open source data sets have become the choice of many autonomous driving companies.
So far, Waymo, Cruise, Baidu, Uber, Lyft, Aptiv and other world-leading autonomous driving companies have successively opened up their own data sets, which has played a pivotal role in promoting the overall development of autonomous driving. However, open source data sets are not the "patent" of autonomous driving companies. Sensor companies are also capable of showing their prowess in this field, and may even do better than autonomous driving companies. The joint release of PandaSet by Hesai Technology and Scale AI is a good example, which has opened up new development ideas for many companies in the autonomous driving industry chain.
Overview of PandaSet open source dataset
PandaSet: A timely help during the epidemic
High-quality labeled data is the "fuel" for training deep learning algorithms. At present, deep learning algorithms used by autonomous driving companies around the world basically need to be trained with labeled data. Only by continuously learning labeled data can deep learning algorithm models help autonomous vehicles better identify obstacles. In addition to autonomous driving companies, other autonomous driving algorithm developers, such as students and academic institutions, also have a continuous and strong demand for high-quality labeled data.
However, this year, due to the impact of the COVID-19 epidemic, a large number of autonomous driving companies have had to suspend road testing, which has directly led to a reduction or even a suspension of available road test data, which has had a serious impact on the training of autonomous driving deep learning algorithm models. Against this background, Hesai Technology and Scale AI recently jointly released the PandaSet open source dataset, which has brought timely relief to many autonomous driving algorithm developers.
The PandaSet dataset uses two LiDARs and six cameras for data collection, including more than 16,000 frames of LiDAR point clouds and more than 48,000 photos, covering more than 100 scenes. In addition to LiDAR point clouds and photos, the dataset also includes GPS (Global Positioning System)/IMU (Inertial Sensor), calibration parameters, annotations, SDK (Software Development Kit) and other information.
PandaSet point cloud and photo annotation comparison
PandaSet uses two laser radars, Pandar64 and PandarGT, for data collection, and is equipped with 6 cameras
It is particularly noteworthy that the PandaSet dataset performs target detection in each of the more than 100 scenes, detecting a total of 28 types of objects; most scenes also perform semantic segmentation, with a total of 37 semantic labels. Target detection uses traditional rectangular annotations. For example, bicycles and cars can be framed by rectangular wireframes. For lidar point cloud data, not every point belongs to a certain target object, so the dataset also accurately annotates the semantic label of each point through the point cloud segmentation tool. Such detailed annotations also provide excellent data for deep learning algorithm models.
The PandaSet dataset also accurately annotates the semantic labels of each point through the point cloud segmentation tool
For an autonomous driving dataset, the diversity and complexity of the scenes are one of the important criteria for measuring its quality. All data in the PandaSet dataset are collected from urban roads in San Francisco and suburban roads in Silicon Valley. These roads cover a variety of traffic information such as cars, bicycles, traffic lights, pedestrians, buildings, etc., which are the most challenging application scenarios for autonomous driving. In addition, the data in the PandaSet dataset covers both daytime and nighttime, which also makes it highly applicable.
3D box annotation of night scene
Don’t be fooled by unreliable datasets
For autonomous driving developers, if they want to train excellent deep learning algorithm models, they must be extra careful when choosing data sets. Because some unreliable data sets not only cannot train the algorithm well, but will bring great harm to the algorithm and have a counterproductive effect. So, what kind of data sets are unreliable? Simply put, inaccurate and incomplete data sets are unreliable data sets.
Some inaccurate and incomplete datasets are leading self-driving cars into trouble, including well-known datasets. A widely used open source dataset of 15,000 images found thousands of images that lacked annotations, hundreds of which did not even have any annotations, but these images did contain cars, trucks, bicycles, street lights or pedestrians. Not only that, the dataset also contained false annotations, copy-paste, and some annotation boxes were significantly larger than the standard.
“Thousands of students are using open source datasets to support their autonomous driving projects, but datasets of poor quality can easily mislead algorithm models, causing autonomous vehicles to make bad decisions, which is disastrous for the development of autonomous driving.”
In fact, the accuracy and completeness of the data set are closely related to the process of data collection and data labeling. For example, in data collection, if the performance of the sensor carried by the collection vehicle is very poor, then the quality of the collected data will definitely be very poor, which will directly affect the subsequent labeling and final use. In data labeling, if there is no complete set of labeling methods, it is easy to have various wrong labels, such as: not marking the objects that exist in the picture, but marking the non-existent objects, or the labeling box does not fit the actual object, or even deviates significantly from the actual object.
PandaSet is an excellent example of how to create a high-quality dataset. In data collection, the two laser radars used by PandaSet for data collection are both industry-leading products. These two laser radars are independently developed by Hesai Technology. One is the forward-looking laser radar PandarGT with image-level resolution, and the other is the 64-line mechanical rotating laser radar Pandar64, which ensures that the collected point cloud is accurate, clear, and delicate enough - the existing open source datasets in the world are generally collected at an early stage, and few use high-performance laser radars such as Pandar64 and PandarGT to collect data.
In addition, in data labeling, Scale AI, which is responsible for this part and is a leader in the labeling field, has a very strict labeling system, including how to label, how to check, how to review, how to re-label unqualified labels, how to manage and evaluate the employees responsible for labeling, etc. In the entire labeling process, Scale AI mainly relies on manual work, combined with computer assistance, to fully ensure the integrity and accuracy of data labeling.
Open source datasets are the trend
As a leader in the autonomous driving industry, Waymo also released its own open source dataset, Waymo Open Dataset, last year. The dataset contains 200,000 frames, 12 million 3D annotations, and 1.2 million 2D annotations. Waymo hopes that its dataset can help developers make progress in 2D and 3D perception, scene understanding, behavior prediction, etc., thereby continuously improving the performance of autonomous vehicles and promoting the application of other related fields such as computer vision and robotics.
Before Waymo released its open source dataset, leading autonomous driving companies such as Cruise, Baidu, Uber, and Aptiv had already released their own open source datasets. After Waymo released its open source dataset, several other companies released open source datasets for autonomous driving, such as Lyft, Ford, and Audi.
Previous article:With FOTA, can cars really do whatever they want?
Next article:Research on the design of charger for new energy vehicles based on three-level LLC resonant converter
Recommended ReadingLatest update time:2024-11-23 11:37
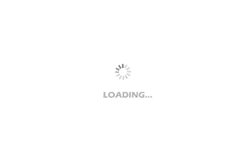
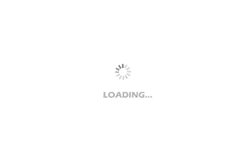
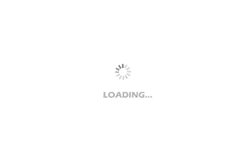
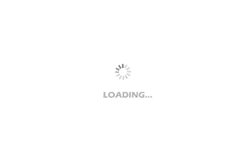
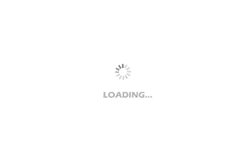
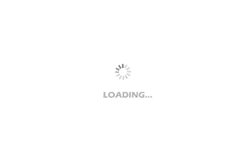
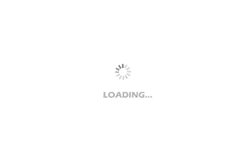
- 2024 China Automotive Charging and Battery Swapping Ecosystem Conference held in Taiyuan
- State-owned enterprises team up to invest in solid-state battery giant
- The evolution of electronic and electrical architecture is accelerating
- The first! National Automotive Chip Quality Inspection Center established
- BYD releases self-developed automotive chip using 4nm process, with a running score of up to 1.15 million
- GEODNET launches GEO-PULSE, a car GPS navigation device
- Should Chinese car companies develop their own high-computing chips?
- Infineon and Siemens combine embedded automotive software platform with microcontrollers to provide the necessary functions for next-generation SDVs
- Continental launches invisible biometric sensor display to monitor passengers' vital signs
- Intel promotes AI with multi-dimensional efforts in technology, application, and ecology
- ChinaJoy Qualcomm Snapdragon Theme Pavilion takes you to experience the new changes in digital entertainment in the 5G era
- Infineon's latest generation IGBT technology platform enables precise control of speed and position
- Two test methods for LED lighting life
- Don't Let Lightning Induced Surges Scare You
- Application of brushless motor controller ML4425/4426
- Easy identification of LED power supply quality
- World's first integrated photovoltaic solar system completed in Israel
- Sliding window mean filter for avr microcontroller AD conversion
- What does call mean in the detailed explanation of ABB robot programming instructions?
- STMicroelectronics discloses its 2027-2028 financial model and path to achieve its 2030 goals
- 2024 China Automotive Charging and Battery Swapping Ecosystem Conference held in Taiyuan
- State-owned enterprises team up to invest in solid-state battery giant
- The evolution of electronic and electrical architecture is accelerating
- The first! National Automotive Chip Quality Inspection Center established
- BYD releases self-developed automotive chip using 4nm process, with a running score of up to 1.15 million
- GEODNET launches GEO-PULSE, a car GPS navigation device
- Should Chinese car companies develop their own high-computing chips?
- Infineon and Siemens combine embedded automotive software platform with microcontrollers to provide the necessary functions for next-generation SDVs
- Continental launches invisible biometric sensor display to monitor passengers' vital signs
- In-depth article: Thermal resistor soft breakdown failure analysis
- Qualcomm adds power management chips to cover all market segments
- Sincerely recruiting embedded software and hardware engineers
- Characteristics, functions and precautions of chopper
- Copper plating under the DC conversion power inductor
- Development of a performance tester for three-disc DVD/CD rack modules
- Regarding FPGA development, compared with several major companies' EDA design tools, Xilinx's Vivado is still the best
- Three in and three out, my ten-year power supply journey
- How to simulate I2C communication using GPIO on C2000
- Never forget the teacher's kindness, teachers, happy holidays~~