When we first contacted Great Wall two years ago, they made such a request. Therefore, the parameters of IbeoNEXT are completely designed to match the autonomous driving function. Its future cost can be predicted. It will be cheaper than mechanical ones. As long as the volume is increased, we think the cost will not be a problem.
Q: OEMs also have corresponding testing links. In comparison, what is the difference in the positioning of Liangdao Smart?
Ju Xueming: In the traditional automotive industry, there are various specialized testing and verification service providers for testing and verification, such as those specializing in engines and electronic modules. Especially in Germany, there are many engineering service companies with thousands or even tens of thousands of employees to undertake many OEM testing and verification tasks.
In fact, we believe that Liangdao Intelligence is targeting a new market. Since there is no testing for intelligent sensing modules in the traditional industry, this is currently a new component in the industry and is a test and verification of intelligent components.
Compared with OEMs and Tier 1, our own positioning is to help OEMs and sensor suppliers, and the three parties work together to improve the safety and reliability of autonomous driving. Because if our tool chain is powerful enough, we can have a more objective feedback on KPIs in a shorter time. This value feedback to OEMs or sensor suppliers is actually an acceleration engine for its algorithm iteration. In this way, the three parties work together to achieve the mass production of autonomous driving. As for the ownership of the data, it needs to be determined based on the specific project.
Q: What specific areas does the Liangdao Intelligent team’s R&D work focus on?
Ju Xueming: Algorithm development based on various sensors, development of tool chains, including the establishment of a big data center.
Q: What are the barriers for testing and verification service providers?
Xueming Ju: The core entrepreneurial team also had relevant experience in German OEM and Tier 1, especially in mass production and development of LiDAR. We have the best understanding of sensors themselves. In addition, we have been targeting this market since 2017. We believe that the mass production of autonomous driving in the future must be tested and verified, so we have been building our tool chain since 2017, whether it is to provide automated annotation or scene capture. Now this tool chain can serve the Great Wall project.
The barriers mentioned here actually require extremely high comprehensive capabilities, even as high as those of algorithm development suppliers. Building this test system is the same as building an environmental perception hardware system and supporting algorithms. You need to understand the normalization and standardization of data collection for the entire test, and you need to work with the OEM and suppliers to complete it.
At the same time, the industry has also put forward new requirements. The current laser radar, especially Flash laser radar, generates a large amount of data per unit time. We need to collect a large amount of mileage data, establish a data platform and data center, and apply various rich tool chains in the data center to perform automatic labeling and automatic scene capture.
Of course, a rich tool chain is also one of the core barriers to meet the requirements of different customers and different scenarios in the future. We hope to establish a complete tool chain and finally develop data mining products based on data.
Q: How is the autonomous driving test verification of multiple vehicles conducted?
Ju Xueming: At present, the test verification of our current products is mainly based on bicycles. We use bicycles as carriers, equipped with sensors to be tested and evaluation systems, to collect data in traffic or other scenarios we need. We are also considering the future model. We have established a team to consider adding various sensors to the facility side to establish the true value. This is equivalent to capturing all the information in the traffic flow as long as you can monitor it. This is more used for driving behavior analysis in the future.
Previous article:Tesla may launch battery products with a lifespan of 1.6 million kilometers in 2020
Next article:Alternative test and verification route for automotive-grade LiDAR
Recommended ReadingLatest update time:2024-11-16 17:27
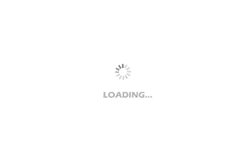
- Popular Resources
- Popular amplifiers
-
A review of deep learning applications in traffic safety analysis
-
Dual Radar: A Dual 4D Radar Multimodal Dataset for Autonomous Driving
-
A review of learning-based camera and lidar simulation methods for autonomous driving systems
-
Multimodal perception parameterized decision making for autonomous driving
- Car key in the left hand, liveness detection radar in the right hand, UWB is imperative for cars!
- After a decade of rapid development, domestic CIS has entered the market
- Aegis Dagger Battery + Thor EM-i Super Hybrid, Geely New Energy has thrown out two "king bombs"
- A brief discussion on functional safety - fault, error, and failure
- In the smart car 2.0 cycle, these core industry chains are facing major opportunities!
- The United States and Japan are developing new batteries. CATL faces challenges? How should China's new energy battery industry respond?
- Murata launches high-precision 6-axis inertial sensor for automobiles
- Ford patents pre-charge alarm to help save costs and respond to emergencies
- New real-time microcontroller system from Texas Instruments enables smarter processing in automotive and industrial applications
- Innolux's intelligent steer-by-wire solution makes cars smarter and safer
- 8051 MCU - Parity Check
- How to efficiently balance the sensitivity of tactile sensing interfaces
- What should I do if the servo motor shakes? What causes the servo motor to shake quickly?
- 【Brushless Motor】Analysis of three-phase BLDC motor and sharing of two popular development boards
- Midea Industrial Technology's subsidiaries Clou Electronics and Hekang New Energy jointly appeared at the Munich Battery Energy Storage Exhibition and Solar Energy Exhibition
- Guoxin Sichen | Application of ferroelectric memory PB85RS2MC in power battery management, with a capacity of 2M
- Analysis of common faults of frequency converter
- In a head-on competition with Qualcomm, what kind of cockpit products has Intel come up with?
- Dalian Rongke's all-vanadium liquid flow battery energy storage equipment industrialization project has entered the sprint stage before production
- Allegro MicroSystems Introduces Advanced Magnetic and Inductive Position Sensing Solutions at Electronica 2024
- Car key in the left hand, liveness detection radar in the right hand, UWB is imperative for cars!
- After a decade of rapid development, domestic CIS has entered the market
- Aegis Dagger Battery + Thor EM-i Super Hybrid, Geely New Energy has thrown out two "king bombs"
- A brief discussion on functional safety - fault, error, and failure
- In the smart car 2.0 cycle, these core industry chains are facing major opportunities!
- The United States and Japan are developing new batteries. CATL faces challenges? How should China's new energy battery industry respond?
- Murata launches high-precision 6-axis inertial sensor for automobiles
- Ford patents pre-charge alarm to help save costs and respond to emergencies
- New real-time microcontroller system from Texas Instruments enables smarter processing in automotive and industrial applications
- EEWORLD University ---- RS-485 Overview
- PicoVGA (1) VGA/TV driver library for Pico
- How to set up the serial port of 51 microcontroller to communicate with PC
- Step-by-step considerations for designing wide-bandwidth, multichannel systems
- DSP Digital Image Design Report
- Digital Integrated Circuits—Circuits, Systems, and Design (Second Edition)
- Two-color graphic details capacitors and application circuits
- JY02A(居逸電子)馬達驅動IC動作原理
- What is Q
- [Construction Monitoring and Security System] XI. Kaluga MQTT connects to OneNET