The traditional PID regulator has the advantages of simple structure, convenient adjustment and close connection between parameter setting and engineering indicators. It has been widely used in process control. However, the algorithm parameters are difficult to set and the parameters do not have adaptive capabilities. Many scholars have proposed improved PID control algorithms. Zhao Jianhua, Shen Yongliang and others [1] derived an adaptive PID control algorithm. The simulation results show the effectiveness of the control algorithm. Neurons, as the basic units of neural networks, have self-learning and adaptive capabilities. The control system algorithm composed of neurons is simple and easy to implement. Combining neuron technology with traditional PID control can solve the problem that traditional PID controllers are difficult to set online real-time parameters to a certain extent [2].
However, in the current neuron PID system, the gain coefficient, one of the most sensitive coefficients, is only set at the initial stage and does not have an online adjustment function. The learning rate is generally obtained through a large number of simulations and experiments and remains unchanged during the control process. These have affected the control effect to a certain extent. Sun Xia Na et al. [3] used a single neuron adaptive fuzzy PID control strategy for the control of active suspension. Based on reference [4], this paper combines neurons with non-identifiable adaptive control rates and proposes an improved single neuron PID controller that adjusts the gain coefficient and learning rate online to further improve the robustness and adaptability of the control system.
In the actual production process, complex control algorithms have poor reusability and are not easy to implement directly in the monitoring configuration environment. ActiveX technology can be flexibly and efficiently applied to various occasions that are reentrant, reused, fully distributed, and language-independent. ActiveX technology expands the functions of monitoring configuration software and enables monitoring configuration software to complete complex and advanced control algorithms. This paper encapsulates the improved single neuron adaptive PID control algorithm in an ActiveX control and applies the control to the control of dissolved oxygen in the sewage treatment process. The simulation results show the effectiveness and practicality of the improved algorithm.
1 PID controller
1.1 Single Neuron PID Controller
The single neuron adaptive PID controller, which is composed of a single neuron with self-learning and self-adaptive capabilities, has a simple structure, can adapt to environmental changes, and has strong robustness. Neurons, as the basic units of neural networks, have the ability of self-learning and self-adaptation, and are easy to calculate. Traditional PID regulators have the characteristics of easy adjustment and close connection between parameter setting and engineering indicators [2]. Combining the two, the shortcomings of traditional PID regulators that it is difficult to adjust parameters online in real time and difficult to effectively control some complex processes and systems with slow parameter changes can be solved to a certain extent. The block diagram of the adaptive PID controller implemented by neurons is shown in Figure 1.
K is the gain coefficient of the neuron. The larger the K value, the better the rapidity, but the overshoot is large, which may even make the system unstable. When the time delay of the controlled object increases, the K value must be reduced to ensure the stability of the system. If the K value is too small, the rapidity of the system will deteriorate. Therefore, it is necessary to select a suitable K value to obtain good system performance.
It can be seen from formula (1) that the correction speed of each weight coefficient depends on its own learning rate. The learning rate of the currently used neuron adaptive PID controller is obtained through a large number of simulations and experiments, and is kept constant during the control process, which limits the further improvement of the controller quality. The gain coefficient, which is the most sensitive to the neuron system, does not have the function of online learning and automatic adjustment, which also limits the control effect to a certain extent.
1.2 Improved single neuron PID controller
In view of the shortcomings of the above controller, the adaptive control algorithm without identification proposed by Marsik and Strejc is combined to dynamically adjust the gain coefficient and self-adjust the learning rate to further improve the robustness and adaptability of the control system.
The non-identification adaptive control algorithm proposed by Marsik and Strejc can be described as:
2 Control Design
In order to enhance the applicability of the algorithm, this paper encapsulates it into an ActiveX control so that the control algorithm can be reused in configuration software or other software that supports ActiveX to achieve online control or simulation. ActiveX control is a software component that can be embedded in many different programs and used as part of the program itself. ActiveX control has the characteristics of portability, strong extensibility, and wide application [5]. In this paper, in the VS2005 environment, the MFC ActiveX Control wizard is used to create an ActiveX control. It creates a shell for any ActiveX control to be created, generates all necessary files and configures the project. When the project is compiled, the compiler will create an ActiveX control, which will generate an OCX file.
The interface properties added in the control include cycle, expected output, feedback input, various controller parameters, etc.; events include controller output exceeding the upper limit, exceeding the lower limit, feedback input exceeding the upper limit, exceeding the lower limit, and methods include clearing the controller output.
Implement the incremental PID and neuron PID control algorithms in the CPidctrl class. Add the WM_TIMER message, get the value of the period attribute to set the timer, and judge the value of ControlFlag in the OnTimer function, which will change as the desired controller type is selected in the user property page. Add the WM_CREATE message, and dynamically add the "Start" and "Set" buttons in the response function to start the controller and pop up the property setting screen respectively. Draw the control appearance in the OnDraw function, display the current expected output, feedback input, controller output and other process quantities and operation, controller output limit exceeding and other signs.
3 ActiveX control application examples
This control is applied to the control of DO (dissolved oxygen) concentration in the sewage treatment process. A typical sewage treatment process is shown in Figure 2.
An MFC application is designed to simulate the dissolved oxygen control process, and an ActiveX control that encapsulates the improved single neuron PID control strategy is used as the controller. A drawing control NTGraphCtrl is added to the program to intuitively display the response curve of the controlled system.
The single neuron PID control strategy before improvement uses a learning rate of ηp=1.4, ηi=1.35, ηd=1.2, and a gain coefficient K=2. The initial values of the learning rate and gain coefficient of the improved single neuron PID controller are the same as those before improvement. The simulation results are shown in Figures 3 and 4. From the above simulation results, it can be seen that the overshoot of the simulation curve of the single neuron PID control before improvement is 6.8%, while the simulation curve controlled by the improved single neuron PID regulator can achieve basically no overshoot, with better control performance. After a large number of simulation experiments, it is found that the control effect of the single neuron PID controller is ideal when the parameters are set appropriately, but the requirements for parameters are relatively sensitive, while the parameters of the improved neuron PID controller have self-adjusting characteristics, showing stronger adaptability and robustness.
In this paper, an improved single neuron PID control strategy with dynamic adjustment of gain coefficient and adaptive learning rate is proposed by combining the adaptive control algorithm without identification. The improved single neuron adaptive PID control algorithm is encapsulated in the ActiveX control by using ActiveX technology, so that the control algorithm can be easily and repeatedly applied to configuration software supporting ActiveX and other application software supporting ActiveX control. In the VS2005 environment, an MFC application is designed to simulate the control of dissolved oxygen in the sewage treatment process. The results show that the improved single neuron PID has better adaptability and stronger robustness than the unimproved single neuron PID control method. The developed ActiveX control can be reused and has good practical value.
Previous article:Analysis of the technical principles of electric bicycle controllers
Next article:Application of digital video optical transceiver in urban traffic monitoring
Recommended ReadingLatest update time:2024-11-16 16:18
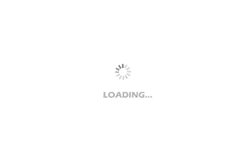
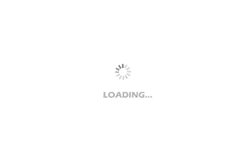
- Popular Resources
- Popular amplifiers
- Molex leverages SAP solutions to drive smart supply chain collaboration
- Pickering Launches New Future-Proof PXIe Single-Slot Controller for High-Performance Test and Measurement Applications
- CGD and Qorvo to jointly revolutionize motor control solutions
- Advanced gameplay, Harting takes your PCB board connection to a new level!
- Nidec Intelligent Motion is the first to launch an electric clutch ECU for two-wheeled vehicles
- Bosch and Tsinghua University renew cooperation agreement on artificial intelligence research to jointly promote the development of artificial intelligence in the industrial field
- GigaDevice unveils new MCU products, deeply unlocking industrial application scenarios with diversified products and solutions
- Advantech: Investing in Edge AI Innovation to Drive an Intelligent Future
- CGD and QORVO will revolutionize motor control solutions
- Innolux's intelligent steer-by-wire solution makes cars smarter and safer
- 8051 MCU - Parity Check
- How to efficiently balance the sensitivity of tactile sensing interfaces
- What should I do if the servo motor shakes? What causes the servo motor to shake quickly?
- 【Brushless Motor】Analysis of three-phase BLDC motor and sharing of two popular development boards
- Midea Industrial Technology's subsidiaries Clou Electronics and Hekang New Energy jointly appeared at the Munich Battery Energy Storage Exhibition and Solar Energy Exhibition
- Guoxin Sichen | Application of ferroelectric memory PB85RS2MC in power battery management, with a capacity of 2M
- Analysis of common faults of frequency converter
- In a head-on competition with Qualcomm, what kind of cockpit products has Intel come up with?
- Dalian Rongke's all-vanadium liquid flow battery energy storage equipment industrialization project has entered the sprint stage before production
- Allegro MicroSystems Introduces Advanced Magnetic and Inductive Position Sensing Solutions at Electronica 2024
- Car key in the left hand, liveness detection radar in the right hand, UWB is imperative for cars!
- After a decade of rapid development, domestic CIS has entered the market
- Aegis Dagger Battery + Thor EM-i Super Hybrid, Geely New Energy has thrown out two "king bombs"
- A brief discussion on functional safety - fault, error, and failure
- In the smart car 2.0 cycle, these core industry chains are facing major opportunities!
- The United States and Japan are developing new batteries. CATL faces challenges? How should China's new energy battery industry respond?
- Murata launches high-precision 6-axis inertial sensor for automobiles
- Ford patents pre-charge alarm to help save costs and respond to emergencies
- New real-time microcontroller system from Texas Instruments enables smarter processing in automotive and industrial applications
- [Raspberry Pi Pico Review] - Unboxing + Download
- Hydrogen escape problem
- [Review of the RTT of the Bluesight AB32VG1 RISC-V board] 5: Tragedy caused by manually replacing the Studio file
- MSP430G2553 study notes DAY1 Knowledge reserve and device initialization
- 2018 Autumn Recruitment of Texas Instruments Reliability Engineer Interview and Written Examination Experience
- Simplicity Studio consultation on energy profile related issues
- C language compilation process
- Correctly analyze the role of each discrete component in the circuit - resistors, capacitors, inductors, diodes, transistors,
- SensorTile.box V431 firmware, suitable for some mobile phones that cannot be OTA upgraded
- Knowledge popularization! "Common algorithms in embedded system software design"