summary
Improvement and optimization in manufacturing processes require clear definition of quantifiable metrics. Yield, cycle time, cost, on-time delivery, and output – this set of manufacturing metrics supports meeting business needs and customer expectations. In many cases, production goals can conflict with each other. This article discusses different metrics and provides corresponding guidance on how to keep in mind customer expectations for on-time delivery.
introduction
Improvement and optimization efforts require clearly defined metrics to be optimized or co-optimized that are measurable within certain constraints. To track and assess the health of a project, we often come up with multiple sets of manufacturing metrics or key performance indicators (KPIs), ranging from as few as 15 to more than 301,2,3. For modeling and optimization, it is best to focus on a few important metrics. A dual standard of “necessary” and “sufficient” can be used to ensure that the expectations of key stakeholders for manufacturing, primarily internal business expectations and external customer expectations, can be assessed and met with a smaller set of important metrics. Focusing on these quantitative metrics helps us generate concise and easy-to-understand dashboards to quickly assess and respond to changing project health indicators. Qualitative metrics for improving these important metrics will be discussed in the second article.
Understand expectations and relevant key indicators
Internal business expectations are usually financially driven, meaning that manufacturing operations must meet the financial expectations of the business in terms of revenue and profitability. External customer expectations are primarily about quality and delivery, i.e. delivering the final product in the promised quantity and expected quality within the promised time.
Starting with internal business expectations, revenue is closely tied to manufacturing output. Eliyahu Goldratt proposed a business approach that emphasizes this connection, output accounting. 4 Similarly, business profit is closely tied to manufacturing output multiplied by the difference between unit price and cost. Manufacturing yield also has a significant impact on unit cost because scrapped product affects the financial health of the business but does not provide revenue. The connection between these financial indicators suggests that the three key manufacturing indicators are output, unit cost, and yield.
External customer expectations of quality are also related to manufacturing yield. Assuming that we have translated customer expectations into product requirements and that products are tested, inspected, or otherwise evaluated based on product requirements that reflect customer expectations, products that do not meet these requirements will be scrapped. Therefore, manufacturing yield is closely related to quality.
Customer expectations for delivery are tied to manufacturing yield and manufacturing cycle time. Delivering the promised number of products depends on the line consistently meeting yield expectations. Delivering the products on the promised time depends on the line meeting cycle time expectations. These customer expectations add two metrics to produce five key manufacturing metrics: output, unit cost, yield, cycle time, and on-time delivery.
Based on the above discussion, the five proposed manufacturing metrics may be sufficient to satisfy business and customer metrics; it is reasonable to question whether each metric is necessary or whether any are redundant. For example, since on-time delivery is a function of both output and cycle time, can on-time delivery be eliminated as a production metric? Alternatively, can cycle time be eliminated since it appears to be related to on-time delivery?
A quick thought experiment can be used to explore these questions: Is it possible to achieve good yields and cycle times in production, but not be able to deliver products to customers on time? Yes, this can happen if the manufacturing world overestimates yields and underestimates cycle times when promising delivery dates. Is it possible to deliver goods to customers on time, but still have customers or the business be unhappy with long lead times? Again, the answer is yes - if manufacturing cycle times are long and prevent them from meeting promised dates, and they can only meet extended delivery dates most of the time, then the business will disappoint customers with long delivery delays and delay revenue, especially if competitors have shorter cycle times and faster delivery.
Therefore, we can assume that this set of manufacturing indicators is a necessary and sufficient condition to meet the focus and co-optimization goals:
► Yield
►Production cycle
► Cost
►On-time delivery
► Output
Industrial engineering and manufacturing engineering personnel can model each metric or KPI separately; however, this can lead to suboptimization, where optimization of one metric can have an adverse impact on another. The ideal approach, then, is to optimize together, perhaps focusing primarily on the metrics that the business considers most important, while setting constraints to limit the reasonable impact on other metrics.
Projects can be set up to achieve this collaborative optimization; for example, Lean Six Sigma and/or Design for Six Sigma initiatives can develop a charter for projects involving manufacturing processes. These metrics are closely related to the Define and Measure phases of the Define, Measure, Analyze, Improve, and Control (DMAIC) tool and help determine if the project, team, and manufacturing process are successful. Not only do metrics inspire behavior and accountability, they can also help teams assess the financial benefits of projects.
"Good quality, fast speed, low price - choose two"
Three of the manufacturing metrics, yield, cycle time, and cost, correspond to the famous motto: "Good quality, fast speed, low price - pick two". This humorous motto implies that it is difficult to make trade-offs between these manufacturing metrics. The last metric, "cost" or "low price", is often considered to have many problems, but the goal of an enterprise is usually more inclined to maximize profits rather than minimize costs. Therefore, a more appropriate goal is to optimize yield, cycle time, and profitability in a coordinated manner - all three are indispensable.
“Good quality” or yield
Yield is a critical parameter in most manufacturing processes, tied to financial results, delivery, and quality. Yield modeling allows teams to predict future yields and prioritize opportunities to improve yield. Yield models combine the predicted yields for each process step into a predicted yield distribution for the entire manufacturing or assembly process. 5 See Table 1.
Table 1. Yield modeling by manufacturing process step
Each process step has a probability of success between 0% and 100% and can therefore be modeled using a statistical distribution that ranges between 0% and 100%, such as the Beta distribution. The overall probability of process success also ranges between 0% and 100% and can likewise be represented by a Beta distribution. Fortunately, combining the probabilities of success for each step by multiplying their Beta distributions together produces another Beta distribution that represents the probability of process success.
If a step in a process has been attempted a number of times (n) and has succeeded s times and failed f = n – s times, then the probability of success can be estimated by α with (s + 1) and β with (f + 1)β, described as β (s + 1, f + 1). This practical approach allows the Beta distribution to be used to model the probability of success of a single step in a manufacturing process, task, or procedure.
If the success of a step in the process is based on a continuous parameter rather than a discrete pass/fail parameter, the probability of success can also be converted to a Beta distribution. Goodness of fit measures for continuous parameters such as Cpk, z-score, or yield can be used to estimate the pass probability p. However, estimating the two parameters of the Beta distribution requires two values, and the pass probability p must be complemented by a second value.
The second value can be the number of samples, n, or it can be assumed that the value of n reflects the degree of uncertainty in the parameter distribution, Cpk, z-score, or yield.
Alpha and beta values for the probability of success can be estimated for each step, whether based on discrete parameters such as actual or predicted number of passes and failures, or on continuous parameters such as Cpk, z-score, or yield.
These α and β values for each step can be combined into an overall probability of success for the entire process, which is equivalent to the overall yield of the manufacturing process. One way to combine these values is to use Monte Carlo simulation, and another method is based on generating system moments. With both methods, sensitivity analysis can be performed to prioritize opportunities to improve yield. See Figure 1.
Figure 1. Histogram of yield distribution.
"Fast" or production cycle
The production cycle is directly related to the ability to respond to customer requests for products in a timely manner, and indirectly related to the probability of on-time delivery: the manufacturer starts putting materials into the production line on a specific date and delivers the product after a delay corresponding to the manufacturing cycle time. The distribution of the production cycle can be approximated by a gamma distribution, with the lower threshold corresponding to the theoretical minimum cycle time. Factors that affect the production cycle are also the percentage (%) of bottleneck steps (as described by Kingman's equation6, see Figure 3), as well as the output of the production line and the work-in-progress (WIP) inventory (as described by Little's law7,8). The cycle time can be effectively modeled and optimized using discrete event simulation. See Figures 2 and 4.
Previous article:PepsiCo Announces Top 10 Finalists for Second Asia Pacific Green Accelerator Program
Next article:10BASE-T1S Technology Overview
Recommended ReadingLatest update time:2024-11-16 10:34
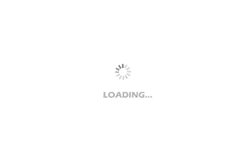
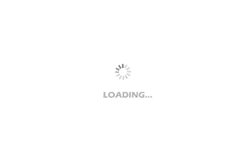
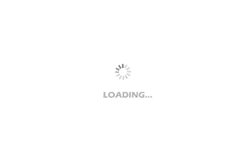
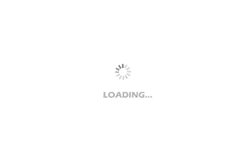
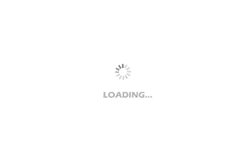
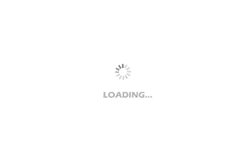
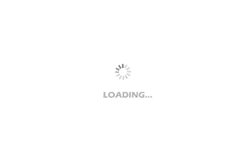
- Popular Resources
- Popular amplifiers
-
Virtualization Technology Practice Guide - High-efficiency and low-cost solutions for small and medium-sized enterprises (Wang Chunhai)
-
New concept analog circuit 1-5
-
\"New Concept Analog Circuit\" - Transistor [Text Version] (Yang Jianguo)
-
ADI Technical Tutorial Collection - Circuit Simulation and PCB Design
- Molex leverages SAP solutions to drive smart supply chain collaboration
- Pickering Launches New Future-Proof PXIe Single-Slot Controller for High-Performance Test and Measurement Applications
- CGD and Qorvo to jointly revolutionize motor control solutions
- Advanced gameplay, Harting takes your PCB board connection to a new level!
- Nidec Intelligent Motion is the first to launch an electric clutch ECU for two-wheeled vehicles
- Bosch and Tsinghua University renew cooperation agreement on artificial intelligence research to jointly promote the development of artificial intelligence in the industrial field
- GigaDevice unveils new MCU products, deeply unlocking industrial application scenarios with diversified products and solutions
- Advantech: Investing in Edge AI Innovation to Drive an Intelligent Future
- CGD and QORVO will revolutionize motor control solutions
- Innolux's intelligent steer-by-wire solution makes cars smarter and safer
- 8051 MCU - Parity Check
- How to efficiently balance the sensitivity of tactile sensing interfaces
- What should I do if the servo motor shakes? What causes the servo motor to shake quickly?
- 【Brushless Motor】Analysis of three-phase BLDC motor and sharing of two popular development boards
- Midea Industrial Technology's subsidiaries Clou Electronics and Hekang New Energy jointly appeared at the Munich Battery Energy Storage Exhibition and Solar Energy Exhibition
- Guoxin Sichen | Application of ferroelectric memory PB85RS2MC in power battery management, with a capacity of 2M
- Analysis of common faults of frequency converter
- In a head-on competition with Qualcomm, what kind of cockpit products has Intel come up with?
- Dalian Rongke's all-vanadium liquid flow battery energy storage equipment industrialization project has entered the sprint stage before production
- Allegro MicroSystems Introduces Advanced Magnetic and Inductive Position Sensing Solutions at Electronica 2024
- Car key in the left hand, liveness detection radar in the right hand, UWB is imperative for cars!
- After a decade of rapid development, domestic CIS has entered the market
- Aegis Dagger Battery + Thor EM-i Super Hybrid, Geely New Energy has thrown out two "king bombs"
- A brief discussion on functional safety - fault, error, and failure
- In the smart car 2.0 cycle, these core industry chains are facing major opportunities!
- The United States and Japan are developing new batteries. CATL faces challenges? How should China's new energy battery industry respond?
- Murata launches high-precision 6-axis inertial sensor for automobiles
- Ford patents pre-charge alarm to help save costs and respond to emergencies
- New real-time microcontroller system from Texas Instruments enables smarter processing in automotive and industrial applications
- How to sign in on the mobile version?
- [Reprint] Summary of Zynq7010 NAND startup
- Free sharing of LDO and DCDC basic principle explanation video
- No response when controlling GPIO in user mode?
- Logistics POS machine with serial port expansion IC
- EEWORLD University Hall----Live Replay: Infineon system solutions make electric motorcycle design more reliable and efficient!
- EEWORLD University Hall----Live Replay: ADI's smoke detector integrated solution based on ADPD188BI
- How to use 4G base stations and 5G base stations in rural areas
- [Popular Science] Why is there an error in the output voltage of a power amplifier?
- [Evaluation of SGP40] 3. UART control test