Machine learning goes mainstream – NXP’s eIQ™ software development environment is smarter and friendlier
Since the advent of the Internet of Things (IoT), there have been many disruptive innovations, and edge intelligence is one of them. The Internet of Things has brought billions of smart connected devices, which transmit terabytes of massive sensor data for AI-based cloud computing. In addition to this, another revolution is underway: machine learning (ML) on edge devices. As more and more smart devices migrate to the edge of the network, NXP keeps up with the trend and provides processing solutions with superior cost, performance and power to promote machine learning technology in multiple markets and applications, allowing end users to enjoy the advantages of stronger security, higher privacy, and less latency.
Machine learning, deep learning, and neural network applications have traditionally been the exclusive domain of data scientists and AI experts. However, this is changing as more machine learning tools and techniques become available that remove some of the complexity from developing machine learning applications. NXP’s eIQ (“Intelligence at the Edge”) machine learning development environment is a great example. eIQ provides a comprehensive set of workflow tools, inference engines, neural network (NN) compilers, and optimized libraries and technologies to help simplify and accelerate machine learning development. eIQ is accessible to users of all skill levels, from embedded developers starting their first deep learning project to experts working on advanced object recognition, classification, anomaly detection, or speech recognition solutions.
Launched in 2018, eIQ Machine Learning Software has been continuously developed to support system-level application and implementation of machine learning algorithms for the NXP i.MX family, from the low-power i.MX RT crossover microcontrollers (MCUs) to the multi-core i.MX 8 and i.MX 8M applications processors based on Arm® Cortex®-M and Cortex®-A cores.
Today's major update
To help machine learning developers become more proficient and efficient with the NXP i.MX 8 processing platform, we have significantly expanded the eIQ software environment with the addition of the new eIQ Toolkit workflow tool, the GUI-based eIQ portal development environment, and the DeepViewRT™ inference engine optimized for i.MX and i.MX RT devices.
Figure 1. A brief overview of the features and workflow of the eIQ Toolkit and eIQ portal
Below is a detailed look at how these powerful new tools in the eIQ software environment help simplify machine learning development, increase productivity, and provide developers with more options and greater flexibility.
eIQ Toolkit: Making machine learning accessible to everyone
Given the potential complexity of machine learning, neural networks, and deep learning applications, as well as the different needs of machine learning developers, simple "one-size-fits-all" tools are not "available to everyone." A better approach is to provide a flexible, comprehensive tool suite that can scale to meet the needs of machine learning developers of different skill levels and experience levels. To this end, we have added a powerful and easy-to-use eIQ Toolkit to the eIQ machine learning development environment, enabling developers to import datasets and models, and train, quantize, validate, and deploy neural network models and machine learning workloads across NXP's i.MX 8M application processor series and i.MX RT crossover MCU portfolio. Whether you are an embedded developer just starting your first machine learning project, or a skilled data scientist or AI expert, you will find features in this tool suite that match your skill level to help simplify your machine learning projects.
Figure 2. The eIQ portal provides dataset management tools to help you annotate and organize all your training data
The eIQ Toolkit provides simple workflows and machine learning application examples. In addition, the toolkit provides an intuitive GUI-based development option in the eIQ portal, or you can choose to use command-line host tools if you prefer. If you want to use a ready-made development solution or need professional services and support from NXP and our trusted partners, you can easily access a list of options provided by companies such as Au-Zone Technologies in the eIQ marketplace through the toolkit, which will be continuously updated.
Figure 3. The eIQ portal provides a convenient way to validate models and measure accuracy.
Through the eIQ portal, you can easily create, optimize, debug, convert, and export machine learning models, or import datasets and models from TensorFlow, ONNX, and PyTorch machine learning frameworks. You can train models with your own data through the "Bring Your Own Data" (BYOD) process, select models from a database of pre-trained models, or import pre-trained models through the "Bring Your Own Model" (BYOM) process, such as advanced detection models from Au-Zone Technologies. By following a simple BYOM process, you can build a trained model using public or private cloud-based tools, and then transfer the model to the eIQ Toolkit to run on the appropriate chip-optimized inference engine.
Figure 4. The eIQ portal provides a flexible BYOM and BYOD approach
Targeted graph-level profiling capabilities provide developers with runtime insights to fine-tune and optimize system parameters, runtime performance, memory usage, and neural network architectures executing on i.MX devices.
New eIQ inference engine accelerates NXP's development
The inference engine is the core of a machine learning development project and the runtime component of a machine learning application. The eIQ machine learning software development environment supports inference using a variety of community-based open source inference engines optimized for i.MX devices and MCUs such as Glow, ONNX, and TensorFlow Lite, and we have added the DeepViewRT inference engine to it.
DeepViewRT is a proprietary inference engine developed jointly by NXP and its partner Au-Zone Technologies that provides a long-term stable solution maintained by the vendor to complement the community-based open source engine.
Figure 5. DeepViewRT provides a stable and flexible production-ready inference engine for machine learning applications
The DeepViewRT inference engine is available as middleware for Linux™ OS-based development in the NXP MCUXpresso SDK and Yocto BSP releases.
More benefits of eIQ
The eIQ machine learning development environment and all necessary benchmark support, including the new eIQ Toolkit, eIQ Portal, and eIQ Inference via DeepViewRT, are all available at no licensing fee.
Hone your skills at the NXP Machine Learning/AI Training Academy
NXP's ML/AI Academy offers self-paced learning modules on various topics related to ML development and provides best practices for using the eIQ tool with NXP i.MX and i.MX RT devices. The ML/AI Academy is open to all NXP customers and offers an ever-expanding set of training modules to help you get started with ML application development.
Get started with eIQ machine learning software and tools today
Click on eIQ Toolkit now to download the eIQ Toolkit including the eIQ Portal.
eIQ machine learning software, including the DeepView RT inference engine for i.MX application processors, is supported on current Yocto Linux releases. eIQ machine learning software for i.MX RT crossover MCUs is fully integrated into NXP’s MCUXpresso SDK release.
For more information, visit www.nxp.com.cn/ai?cid=pr and www.nxp.com.cn/eiq?cid=pr.
Join the eIQ community: eIQ Machine Learning Software.
Click here to learn more about DeepViewRT and Au-Zone’s machine learning development tools.
author:
Ali Ors
Director of Artificial Intelligence and Machine Learning Strategy at NXP Semiconductors Edge Processing Division
Ali has over 23 years of experience in the semiconductor industry and is skilled in leading cross-functional teams to build innovative products and platforms in the fields of machine learning and vision processing. He is currently responsible for the global artificial intelligence machine learning strategy.
Previous article:ANCA Integrated Manufacturing System: Exploring the new future of the “dark factory”
Next article:Element14 Releases: Growing Demand for Low-Cost Single Board Computers for Industrial and IoT Applications
Recommended ReadingLatest update time:2024-11-22 10:31
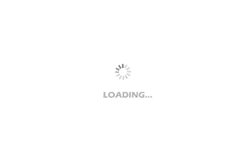
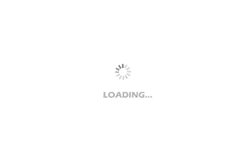
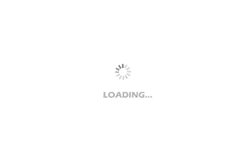
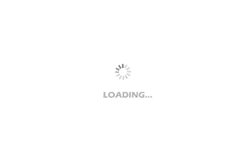
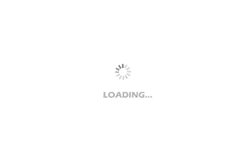
- Popular Resources
- Popular amplifiers
-
Wireless Sensor Network Technology and Applications (Edited by Mou Si, Yin Hong, and Su Xing)
-
Introduction to Internet of Things Engineering 2nd Edition (Gongyi Wu)
-
Sensor Principles and Applications (Yan Xin)
-
Sensor Principle and Application Circuit Design (Edited by Chen Shuwang, Song Lijun, Xu Yunfeng)
- ADLINK Launches AmITX Mini-ITX Motherboard to Power Edge AI and IoT Innovation
- e-Network Community and NXP launch Smart Space Building Automation Challenge
- The Internet of Things helps electric vehicle charging facilities move into the future
- Nordic Semiconductor Launches nRF54L15, nRF54L10 and nRF54L05 Next Generation Wireless SoCs
- Face detection based on camera capture video in OPENCV - Mir NXP i.MX93 development board
- The UK tests drones equipped with nervous systems: no need to frequently land for inspection
- The power of ultra-wideband: reshaping the automotive, mobile and industrial IoT experience
- STMicroelectronics launches highly adaptable and easy-to-connect dual-radio IoT module for metering and asset tracking applications
- This year, the number of IoT connections in my country is expected to exceed 3 billion
- Intel promotes AI with multi-dimensional efforts in technology, application, and ecology
- ChinaJoy Qualcomm Snapdragon Theme Pavilion takes you to experience the new changes in digital entertainment in the 5G era
- Infineon's latest generation IGBT technology platform enables precise control of speed and position
- Two test methods for LED lighting life
- Don't Let Lightning Induced Surges Scare You
- Application of brushless motor controller ML4425/4426
- Easy identification of LED power supply quality
- World's first integrated photovoltaic solar system completed in Israel
- Sliding window mean filter for avr microcontroller AD conversion
- What does call mean in the detailed explanation of ABB robot programming instructions?
- "Cross-chip" quantum entanglement helps build more powerful quantum computing capabilities
- Ultrasound patch can continuously and noninvasively monitor blood pressure
- Ultrasound patch can continuously and noninvasively monitor blood pressure
- Europe's three largest chip giants re-examine their supply chains
- Europe's three largest chip giants re-examine their supply chains
- Breaking through the intelligent competition, Changan Automobile opens the "God's perspective"
- The world's first fully digital chassis, looking forward to the debut of the U7 PHEV and EV versions
- Design of automotive LIN communication simulator based on Renesas MCU
- When will solid-state batteries become popular?
- Adding solid-state batteries, CATL wants to continue to be the "King of Ning"
- Embedded systems and real-time software development
- Summary of the points to note when using CCS8.0 to program MSP430G2553
- picture
- A fire broke out at Foxconn in Shenzhen, with thick smoke billowing from the scene
- PCB copper plating
- Color card combination lock
- There are always a few places in PCB design where impedance cannot be continuous. What should I do?
- Taking a “family-first” approach to op amp design
- Design of smart greenhouse planting control system based on 5G network
- CCS5.5.0 compilation problem: "different version of compiler"