Fingerprint recognition is the most common and reliable technology used in automatic personal identification technology. In general, the implemented technology divides automatic fingerprint identification (AFAS) into two stages performed at different times and under different conditions: enrollment and identification.
During the enrollment process, the user provides the system with a fingerprint, which is then processed through a series of computationally intensive image processing steps to extract all relevant, permanent and unique information, allowing the system to unambiguously identify the true owner of the fingerprint. This set of characteristics constitutes the user ID (identification number), which is stored in the system's database. This process is usually performed offline in a secure environment under the guidance of a professional.
Fingerprint identification is to see if it matches an approved user in the database. The various processing tasks performed during the enrollment process are repeated to extract unique features from the current fingerprint sample. The system then compares these features with the information stored in the database as a template for the user to confirm whether the current fingerprint sample matches the registered template. Depending on the size of the database, identification is divided into two modes: one-to-one or one-to-many matching. Identification is generally completed in a low-security environment and under real-time constraints.
Each step here is broken down into a series of independent tasks to extract unique information about the user from the fingerprint image. To this end, the system will perform a series of specific operations, such as image processing (2D convolution, morphological operations), trigonometric operations (sine, cosine, inverse tangent, square root) [1] or statistics (mean, variance).
Therefore, biometric recognition applications are composed of a series of tasks that are executed in a sequential process. Because the output data of a given task in this chain is the input data of the next task, the start of a task needs to wait for the completion of the previous task. In addition, during the login stage and the recognition stage, many tasks are executed repeatedly.
Figure 1 lists the tasks that take place in the current algorithm. The first is image acquisition. Depending on the size of the sensor, the system can acquire the entire image at once (full image sensor) or in slices (scanned sensor). In the second case, which is the one we are using, an additional image reconstruction phase is required. The complete fingerprint image is composed of continuous and partially overlapping image strips [2].
After we obtain the entire reconstructed image, the next step is to segment the foreground (i.e. the area of interest formed by the convex and concave areas of the fingerprint skin) from the background. We perform image convolution pixel by pixel using a 5x5 pixel Sobel edge detection filter. After completion, we normalize the image with a specific mean and variance.
Next, we enhance the normalized image by isotropic filtering. This step uses 13x13 pixels to recover relevant information from image regions that were previously lost or corrupted by noise during the acquisition phase [3]. The next step after the image enhancement step is to calculate the fingerprint vector map (field orientation map) to determine the main directions of the ridges and valleys in the foreground of the image. The resulting orientation field (eld orientation) is then submitted to a new filtering step (5x5 pixels) to obtain a refined vector map.
At this point, the image is still 8-bit grayscale. In the binarization process, the grayscale image is convolved by a 7x7 pixel Gabor directional filter to improve the clarity of the ridges and valleys and convert each grayscale pixel into a 1-bit binary (black or white) point. The synthesized ridge and valley images are smoothed and redrawn again. Subsequently, the black traces of the black and white image are made one pixel wide by thinning or skeletonization. It is not difficult to extract the characteristics or details of the fingerprint from this image, namely the ridge end points and ridge bifurcation points.
Finally, after obtaining the fingerprint details and direction field data, the fingerprint template and sample can be compared. Here, a relatively direct algorithm is used to achieve the best possible coincidence between the two, taking into account the acceptable errors caused by translation and rotation actions and image deformation caused by skin elasticity during the acquisition phase [4]. The next step is to match the sample and template to obtain the similarity between the two. The automated system can then determine whether the two images belong to the same person based on the similarity [5].
In the whole processing process shown in Figure 3, the fingerprint image resolution used is 500dpi, the grayscale is 8 bits, and the image size is 280x512 pixels. The image acquisition adopts Atmel's thermal fingerprint sensor FingerChip scanning technology, and the calculation adopts Xilinx Virtex-4 XC4VLX25 FPGA device.
System Architecture
The Virtex-4 FPGA device is the computing unit of the AFAS platform, where Flash is used as the system database to store FPGA configuration data and application-specific data such as user fingerprint templates or biometric algorithm configuration settings. In addition, the system uses DDR-SDM memory to temporarily save intermediate data or images obtained from each processing stage. We use serial communication, in our case an RS-232 transceiver connected to a UART controller—the latter can be synthesized in FPGA resources—for debugging purposes. The purpose is to transfer the result image generated by each stage to a PC in order to graphically view the fingerprint image or result of each step. Finally, a scanning fingerprint sensor is used to obtain the user's biometric characteristics and serve as input to the recognition algorithm, as shown in Figure 2.
As a computing unit, the FPGA is divided into two areas, a static area consisting of a complete multi-processor CoreConnect bus system; and a reconfigurable area for placing customized biometric coprocessors or IP (intellectual property) as needed to perform various sequential tasks of the recognition algorithm and reuse them as the processing progresses. The multi-processor CoreConnect bus system is mainly composed of Xilinx MicroBlaze processors and other standard peripherals, and also has a reconfiguration controller linked to the ICAP (Internal Configuration Access Channel) port.
As shown in Figure 1, all processing tasks are enumerated in the order of sequential execution from 0 (static) to B. The custom hardware coprocessor is responsible for implementing all tasks in the PRR (Partial Reconfiguration Region), except for the fingerprint acquisition process which is done by MicroBlaze in software.
The specific division of hardware and software is due to the fact that the scanning sensor requires an integration time of 5μs to obtain a continuous image strip (SLICE). At this speed, there is no need to use a custom hardware coprocessor. Using MicroBlaze software to acquire and reconstruct the image is not only fast enough, but also simpler and more economical.
Image acquisition is performed at a rate of 5 μs per SLICE, with 100 SLICEs, each SLICE being 280x8 pixels in size. The pixel overlap between two consecutive image SLICEs is detected by the software, thereby completing real-time image reconstruction.
Due to real-time requirements, the remaining tasks are implemented by the custom hardware coprocessor of the FPGA's PRR. Once each specific task is completed, the reconfiguration controller located in the static area of the device loads the working module of the next task under the control of the MicroBlaze processor. The reconfiguration controller completes this task by transferring the configuration data of the new module directly from the DDR-SDM to the internal FPGA configuration memory through the ICAP interface.
It is worth mentioning that we use a standard interface between the static and reconfigurable regions based on FIFO (First In First Out) memories and trigger registers. This allows us to develop a standard biometric coprocessor or IP in the PRR regardless of which multiprocessor bus the system uses, whether it is AMBA, CoreConnect, Wishbone or other, as shown in Figure 2. This is fundamental because it ensures standardization and portability of biometric algorithms across different platforms. Reconfiguration
Controller
Designing an efficient reconfiguration controller is key to successfully deploying a PR (partial reconfiguration) system for a single-environment FPGA. Although the non-reconfiguration area of the FPGA is still in operation during the reconfiguration of the PRR, the PRR resources are not in operation at this time, so the reconfiguration process should be accelerated as much as possible to minimize the overhead. The reconfiguration time depends on three factors: data bus width, reconfiguration frequency, and bitstream size. The first two factors are related to the interface characteristics, while the last one is related to the size of the PRR and the design complexity of the partial reconfiguration module (PRM) in it.
Our work implements a reconfiguration controller that can transfer partial bitstreams from external memory to the on-chip configuration memory of the FPGA at runtime with high bandwidth. The highest reconfiguration bandwidth of Virtex-4 can still be achieved without limiting the size of the partial bitstream and using the external memory as a shared resource that can be accessed simultaneously by various processors through the system bus.
During the system initialization phase, partial bitstreams are downloaded on the fly into the FPGA configuration memory and transferred from external Flash to external DDR-SDRAM. This memory is connected to the Multi-Port Memory Controller (MPMC), making it a resource accessible to any master or slave processor in the system. Different types of buses can be used to connect to the MPMC, such as the CoreConnect PLBv46 bus, which can be used as a general system bus, and the Xilinx Cachelink (XCL) bus for fast instruction and data caches of the CPU. The system CPU (MicroBlaze) is actually connected to both buses.
However, our reconfiguration solution is based on a new bus, the Raw Port Interface (NPI), which is designed to quickly connect the external DDR-SDRAM memory bank and the ICAP interface. As part of our reconfiguration controller, we designed the host system memory management unit (MMU) to handle the NPI protocol. The connection between the external DDR-SDRAM (partial bitstream) and the ICAP raw needs to go through an internal FIFO memory. With this approach, we can implement two different custom interfaces, each with independent data bus size and speed, one coupled with the NPI protocol and the other with the ICAP protocol.
The write port of the FIFO is connected to the NPI and uses a 64-bit data bus, while the read port of the FIFO is connected to the ICAP and uses a 32-bit data width, which is the maximum data width of the ICAP in Virtex-4 devices. The read and write ports of the FIFO (on the NPI side and the ICAP side) run at a frequency of 100MHz. To minimize the transfer latency, the main system MMU is responsible for transferring the configuration data to the internal FIFO in 64-word (32-bit) bursts to complete the module reconfiguration. This is the maximum acceptable burst length, so all reconfiguration data transfers can be completed with the lowest burst latency. On the other side, as long as the FIFO is not empty, the reconfiguration controller can read the stored FIFO data and transfer it to the ICAP interface in 32-bit format. The reconfiguration controller (that is, the main MMU) is responsible for handling direct memory access (DMA) to the large DDR-SDM memory. To achieve this, we customized a slave MMU and set multiple control registers in it. This MMU was hung on the PLBv46 bus and directly controlled by the CPU.
In this way, the CPU only needs to do two things: configure the initial address and size of the partial bitstream downloaded in the PRR; issue an execution instruction to the main system MMU to start the reconfiguration process. The main system MMU then starts to transfer the bitstream directly to the internal FIFO in a DMA (direct memory access) manner, and then transfer it from the FIFO to the ICAP interface. Once the transfer is completed, the reconfiguration controller will notify the CPU.
As a result, we can achieve the maximum throughput of partial bitstream transfers even when the CPU is accessing the DDR-SDRAM through the XCL or PLBv46 bus. The ultimate reason for this is that the CPU runs the program flow in the internal BM (block-M) cache, releasing the access to the external DDS-SDRAM to the reconfiguration controller. It is important to point out that this DDR-SDRAM memory allocated for partial bitstream and software application is not a dedicated resource, but a shared resource. Even so, this scheme has a significant performance improvement over other existing reconfiguration controller schemes because it can achieve the maximum reconfiguration throughput of Virtex-4 (transferring partial bitstreams to ICAP through a 32-bit data bus at a frequency of 100MHz or a rate of 3.2 Gbps).
Experimental Results
Essentially, the embedded automatic fingerprint recognition system described in this article is a high-performance image processing application because it has a large amount of parallelism and requires real-time authentication response. From an ergonomic point of view, this system can achieve an authentication time of no more than 2 or 3 seconds per user.
The design flow involved multiple development loops. First, we developed the algorithm in MATLAB software on a PC platform. We then imported the software code into the embedded software using the C programming language and executed it first on the same PC to confirm that we could get the same results, and then on the MicroBlaze embedded microprocessor synthesized within the FPGA device.
In this way, the Virtex-4 device can implement a pure software solution based on MicroBlaze without using any custom hardware coprocessors and without meeting real-time performance requirements. To reduce the running time, according to the task brief, our next step is to introduce PRR and build various custom biometric coprocessors on it, using a hardware/software co-design solution. At this point, we have completed the development of this system using the C programming language and the VHDL hardware description language.
We performed some recognition tests using 8-bit grayscale fingerprint images of 268x460 pixels. We also performed the same tests on a Virtex-4-based PR system and on a PC with an Intel Core 2 Duo T5600 processor running at 1.83GHz. We then ran the same algorithm, both in software-only and hardware-software hybrid implementations, to compare the performance of the login and recognition phases.
If we ignore the acquisition work (due to the performance limitation of the scanning sensor, we need to acquire 100 slices with a 5ms integration time and reconstruct the image on the fly, so the acquisition time is fixed at 500ms), the PR method can reduce the delay caused by running other processing tasks to 205ms. Compared with the 3,274ms delay of the pure software method running on a PC, the PR method is 16 times faster.
Therefore, Table 1 shows that it is feasible to achieve real-time authentication by using parallel and pipeline technology for hardware and software co-design, and PR technology with low reconfiguration delay. In addition, during dynamic reconfiguration, the frequency at which the module runs can be specified, which is determined by the characteristics of the new module. In our design, all modules run at a frequency of 50MHz or 100MHz.
Furthermore, the reconfiguration process always runs at 100MHz, transferring 32 bits per clock cycle, thus guaranteeing the lowest reconfiguration latency on Virtex-4. Depending on the bitstream complexity of each PRR hardware environment, each reconfiguration process takes between 0.8ms (e.g. normalization) and 1.1ms (e.g. binarization). This reconfiguration time is negligible compared to the overall runtime of the biometric application.
Since we have successfully completed the proof of concept, we are ready to export the prototype to the next generation of Xilinx low-end 28nm FPGA devices with PR capabilities (Artix-7 series). Our goal is to design a high-performance and truly secure biometric system that can be embedded in any consumer electronic product at the lowest cost.
Previous article:FPGA Implementation of Root Raised Cosine Pulse Shaping Filter
Next article:Principle Design of Multimedia Advertising System Based on Nios II
Recommended ReadingLatest update time:2024-11-17 07:54
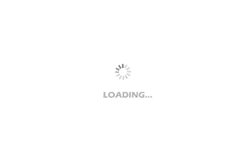
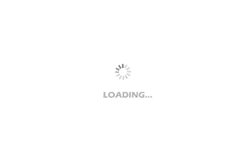
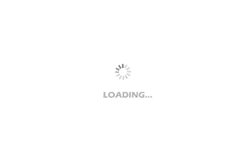
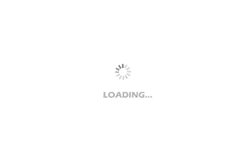
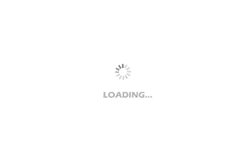
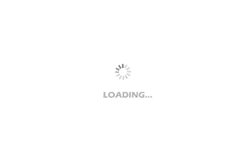
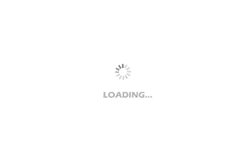
- Popular Resources
- Popular amplifiers
-
Analysis and Implementation of MAC Protocol for Wireless Sensor Networks (by Yang Zhijun, Xie Xianjie, and Ding Hongwei)
-
MATLAB and FPGA implementation of wireless communication
-
Intelligent computing systems (Chen Yunji, Li Ling, Li Wei, Guo Qi, Du Zidong)
-
Summary of non-synthesizable statements in FPGA
- Huawei's Strategic Department Director Gai Gang: The cumulative installed base of open source Euler operating system exceeds 10 million sets
- Analysis of the application of several common contact parts in high-voltage connectors of new energy vehicles
- Wiring harness durability test and contact voltage drop test method
- Sn-doped CuO nanostructure-based ethanol gas sensor for real-time drunk driving detection in vehicles
- Design considerations for automotive battery wiring harness
- Do you know all the various motors commonly used in automotive electronics?
- What are the functions of the Internet of Vehicles? What are the uses and benefits of the Internet of Vehicles?
- Power Inverter - A critical safety system for electric vehicles
- Analysis of the information security mechanism of AUTOSAR, the automotive embedded software framework
Professor at Beihang University, dedicated to promoting microcontrollers and embedded systems for over 20 years.
- Innolux's intelligent steer-by-wire solution makes cars smarter and safer
- 8051 MCU - Parity Check
- How to efficiently balance the sensitivity of tactile sensing interfaces
- What should I do if the servo motor shakes? What causes the servo motor to shake quickly?
- 【Brushless Motor】Analysis of three-phase BLDC motor and sharing of two popular development boards
- Midea Industrial Technology's subsidiaries Clou Electronics and Hekang New Energy jointly appeared at the Munich Battery Energy Storage Exhibition and Solar Energy Exhibition
- Guoxin Sichen | Application of ferroelectric memory PB85RS2MC in power battery management, with a capacity of 2M
- Analysis of common faults of frequency converter
- In a head-on competition with Qualcomm, what kind of cockpit products has Intel come up with?
- Dalian Rongke's all-vanadium liquid flow battery energy storage equipment industrialization project has entered the sprint stage before production
- Allegro MicroSystems Introduces Advanced Magnetic and Inductive Position Sensing Solutions at Electronica 2024
- Car key in the left hand, liveness detection radar in the right hand, UWB is imperative for cars!
- After a decade of rapid development, domestic CIS has entered the market
- Aegis Dagger Battery + Thor EM-i Super Hybrid, Geely New Energy has thrown out two "king bombs"
- A brief discussion on functional safety - fault, error, and failure
- In the smart car 2.0 cycle, these core industry chains are facing major opportunities!
- Rambus Launches Industry's First HBM 4 Controller IP: What Are the Technical Details Behind It?
- The United States and Japan are developing new batteries. CATL faces challenges? How should China's new energy battery industry respond?
- Murata launches high-precision 6-axis inertial sensor for automobiles
- Ford patents pre-charge alarm to help save costs and respond to emergencies
- msp430fr5992 runtime problem
- LabView Note 4: Temperature Monitoring System Based on LabView Password Login
- Introduction to IC chip types
- [15th Anniversary] Wireless Serial Port PCB Arrived
- The command configurations in the manual are all expressed in hexadecimal. How should they be used in the code?
- I just used MPLAB X IDE, and I get a compilation error. I don't understand why.
- Show off the new products that have just been launched
- Getting Started Manual for Oscilloscopes Written by Tektronix
- Learn “how to arrange” and “how to connect”, PCB design specifications are so easy!
- Here are some questions about the ground insulation detection circuit