Qualcomm 8295 is the chip used in General Motors' Ultra Cruise in 2023. It is basically the automotive version of Qualcomm Snapdragon 888. The initial price of Snapdragon 888 was about US$240, and it is currently about US$170 (Qualcomm's public information can be used to check its MSM chip shipments and revenue, with an average price of about US$30-35). Because most of the costs have been covered by mobile phones with shipments of 20 to 30 million, the price of SA8295 can be very low.
However, Qualcomm's automotive chips are generally outsourced to TSMC, which has much higher prices than Samsung (TSMC's operating profit margin is almost 4 times that of Samsung's wafer foundry business). It is estimated that the price of SA8295 is about US$150. If it is Samsung's 5nm, the estimated price is US$120 or US$100. However, Qualcomm wants to add an AI accelerator, but the price of the AI accelerator is not expected to exceed US$50. Taken together, Qualcomm still has a price advantage.
Orin's shipment volume is naturally not comparable to Snapdragon 888, but Samsung's mature technology, plus the fact that there are multiple versions such as cockpit version and game console version to share the cost, the price is estimated to be US$320. However, this unit price is not very meaningful. At present, L3/L4 smart driving vehicles are expensive, and the technology iteration is very fast. The product life cycle is getting shorter and shorter, and the shipment volume throughout the life cycle is negligible. The development cost spread over each vehicle far exceeds the ECU hardware cost. Manufacturers consider the overall cost, especially the software cost and one-time fees, and should not care about the unit price of SoC. Chip manufacturers also promote a full set of solutions, including both hardware and software.
On November 9, 2021, NVIDIA officially launched the module using Orin, namely Jeston AGX Orin, which means that individual users can also buy the top computing module in the field of autonomous driving. The price of Jeston AGX Orin was $1,099 (now the price has dropped to $699, and the domestic price is about 6,000 yuan including tax). The price of Jeston AGX Orin will not be too high, estimated to be $1,499-1,799, and the price is estimated to be $1,299 in three years.
Image source: Internet
The module also includes 32GB of LPDDR5, with a bandwidth of 204.8GB/s and a price of about $105. The price of LPDDR5 has risen recently, and even Apple 13 uses LPDDR4 to save costs. The 64GB eMMC is very cheap, currently priced at $7. Other key chips include a QSPI NOR and a Secure NOR, both of which are not expensive, estimated at $5-8. There is also a power system.
Image source: Internet
The internal framework diagram of Orin can be simply divided into five parts: storage, peripherals, CPU, GPU and accelerator.
Image source: Internet
Orin functional framework diagram
Image source: Internet
Part of the framework of the Orin CPU. The A78 here should be the A78AE (Automotive Enhanced), which is the A78 for the automotive field. ARM recommends that the A78 use a 5nm process and run at a frequency between 2.1GHz and 2.8GHz. Considering the automotive regulations, Nvidia has set the upper limit of the operating frequency to 2GHz. For cost considerations, it did not use the 5nm process, but used Samsung's 8nm process, which is similar to TSMC's 10nm process.
NVIDIA abandoned its self-developed big and small core architecture and switched to ARM's cluster architecture, which is the DSU, DynamIQ Shared Unit (DSU) control unit proposed by ARM in 2017. It allows up to 8 CPU cores to form a cluster, and a single processor can achieve up to 32 clusters. In this way, a processor can have up to 256 cores and can be expanded to 1,000 cores through the CCIX bus.
NVIDIA has not released the CPU framework diagram of Xavier. It should be a cluster of 4 cores, with two clusters. NVIDIA Xavier's cache is still described in detail.
From the cache point of view, Orin seems to be more concerned about the cost. The caches of L2 and L1 are relatively small, but the cache of L3 is not small.
Image source: Internet
The internal framework of A78AE seems to be for memory protection and lockstep, so the L1 cache capacity is not high. DSU can allocate caches at all levels and is also responsible for controlling the switch of each CPU core in the cluster, the frequency, and the voltage. It is the key to controlling CPU performance and power consumption. Therefore, the DSU part has logical control redundancy. This is the main difference from the consumer A78, that is, the addition of DSU-AE.
Image source: Internet
In partition mode, DSU controls each cluster to run at full power. In lock-step mode, one core in each cluster is in sleep mode. Once an abnormality is detected, the backup system is activated.
Image source: Internet
In terms of GPU, each stream processor SM contains 128 CUDA cores, with a total of 16 SMs, a total of 2048 CUDA, and a computing power of 4096 GFLOPS. There are also 64 tensor cores, with a computing power of 131TOPS under sparse INT8 model, or 54TOPS under dense INT8.
Image source: Internet
The 64 tensor cores use half-precision matrix multiplication and accumulation and integrated multiplication and accumulation instruction sets, HMMA (Half-Precision Matrix Multiply and Accumulate) and IMMA (Integer Matrix Multiple and Accumulate), so that the GPU architecture can also correspond to dense algebraic operations and deep learning reasoning. NVIDIA uses a fine transformation weight system to convert dense training weights into a sparse weight model. The sparse constraint is that for every 4 weights, two cannot be zero. After this transformation, the access space of the weights is greatly reduced, and the tensor processing can also skip zero values, which doubles the speed.
Image source: Internet
The internal framework of NVIDIA's deep learning accelerator. NVIDIA's deep learning accelerator is aimed at inference applications. Perhaps because it is considered to have no technical content, NVIDIA introduced DLA very simply. In just a few words, the GPU, CPU, and PVA are introduced in detail. Indeed, the deep learning accelerator has no technical content, it is just a stack of multiplication and accumulation units. The improvement is the addition of 608KB of buffer, which should actually be the addition of 608KB of SRAM, which improves the operating efficiency and small models do not need to read DRAM frequently. The performance of this DLA is 97TOPs for INT8 sparse model and 194TOPs for two. The previous generation of Xavier is 11.4TOPs, but it is a dense model.
Image source: Internet
The PROGRAMMABLE VISION ACCELERATOR programmable vision accelerator, or PVA, is shown in the figure above. Compared with Xavier's first-generation PVA, 1MB of L2 is added, and the rest remains almost unchanged. PVA is mainly used for vector operations such as filtering, distortion, graphic triangle generation, feature detection, and FFT. The specific applications are mainly stereo binocular, feature detectors, feature tracking, and target tracking. It contains two 7-slot (two scalar, two vector, and three storage) VLIW vector processors, two DMA engines, and a real-time Cortex-R5.
Image source: Internet
The typical application of PVA is stereo binocular disparity pipeline. It is particularly important to point out that NVIDIA has been promoting VPI. Vision Programming Interface (VPI) is NVIDIA's high-performance computer vision/image processing algorithm library interface. VPI provides a unified interface for various hardware, such as CPU, GPU, Programmable Vision Accelerator (PVA), and Video Image Compositor (VIC), and provides convenient GPU parallel functions. Supported algorithms include Gaussian pyramid generator, Laplacian pyramid, separable image compressor, box image filter, Gaussian image filter, bilateral image filter, image rescaling, image remapping, image histogram, histogram equalization, fast Fourier transform, inverse fast Fourier transform, image format converter, perspective warping, background subtraction, lens distortion correction, temporal noise reduction, pyramid LK optical flow, and its own commonly used algorithms. NVIDIA VPI seems to intend to replace OpenCV. On NVIDIA's computing platform, VPI is significantly faster than OpenCV.
Some mobile terminals, such as Separable Convolution, have increased efficiency by 29 times. NVIDIA has monopolized deep learning with CUDA, and its next goal is to monopolize computer vision algorithms with VPI.
Image source: Internet
In terms of interfaces, it provides up to 6 CSI camera interfaces, which may not seem like much, but it can be increased to 16 through virtual channels. Generally, autonomous driving uses dual Orin, and 16 MIPI CSI channels are 4 8-megapixel cameras, while dual Orin is 8 8-megapixel cameras.
Image source: Internet
The interface basically corresponds to the architecture in the above picture, with 16 4-megapixel cameras, 8 lidars, and 1 1G Ethernet. Two 10G Ethernets are connected to the backbone network and switches. Compared with Xavier, Orin's AI computing power mainly comes from DLA, while Xavier is GPU. Judging from the simple bare crystal picture, the next generation of Atlan should return to the Xavier route. The AI computing power mainly comes from the GPU. The area of the GPU is much larger than that of the DLA. Because a DPU module has been added, the area of the DLA has been greatly compressed. The code name of the next-generation GPU architecture may be Ada Lovelace. Ada Lovelace is the first programmer in human history, the daughter of the famous British poet Byron, and a mathematician.
Orin does not seem to be very complete, especially the CPU. With a series of new technologies from ARM after A78, Apple, Samsung, Intel and even MediaTek are capable of challenging Orin. The problem is that the L3/L4 smart car market is too small compared to mobile phones and PCs, and to provide a full set of solutions, latecomers spend a lot on software, which makes Orin almost monopolize the market. If domestic chips want to challenge Orin, they must purchase ARM's most advanced architecture and use at least 5 nanometers of advanced technology, which results in a one-time cost of at least $100 million. The overall development cost of the chip is expected to be more than $200 million. Even if the shipment volume is 100,000 vehicles throughout the life cycle, the cost of a single SoC is $2,000. Obviously, this price is unacceptable to car manufacturers. It is completely impossible for any company to challenge Orin in the automotive market alone.
Previous article:IAR Systems supports NXP S32K3 MCU family for next-generation automotive applications
Next article:In-depth analysis of TI's second-generation radar chip
Recommended ReadingLatest update time:2024-11-16 14:55
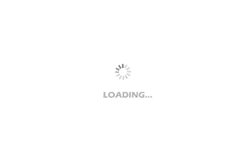
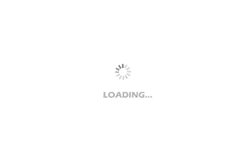
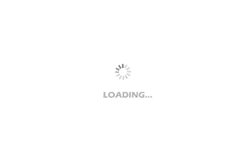
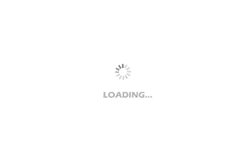
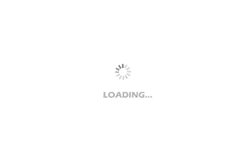
- Popular Resources
- Popular amplifiers
-
Virtualization Technology Practice Guide - High-efficiency and low-cost solutions for small and medium-sized enterprises (Wang Chunhai)
-
A review of deep learning applications in traffic safety analysis
-
Dual Radar: A Dual 4D Radar Multimodal Dataset for Autonomous Driving
-
A review of learning-based camera and lidar simulation methods for autonomous driving systems
- Car key in the left hand, liveness detection radar in the right hand, UWB is imperative for cars!
- After a decade of rapid development, domestic CIS has entered the market
- Aegis Dagger Battery + Thor EM-i Super Hybrid, Geely New Energy has thrown out two "king bombs"
- A brief discussion on functional safety - fault, error, and failure
- In the smart car 2.0 cycle, these core industry chains are facing major opportunities!
- The United States and Japan are developing new batteries. CATL faces challenges? How should China's new energy battery industry respond?
- Murata launches high-precision 6-axis inertial sensor for automobiles
- Ford patents pre-charge alarm to help save costs and respond to emergencies
- New real-time microcontroller system from Texas Instruments enables smarter processing in automotive and industrial applications
- Innolux's intelligent steer-by-wire solution makes cars smarter and safer
- 8051 MCU - Parity Check
- How to efficiently balance the sensitivity of tactile sensing interfaces
- What should I do if the servo motor shakes? What causes the servo motor to shake quickly?
- 【Brushless Motor】Analysis of three-phase BLDC motor and sharing of two popular development boards
- Midea Industrial Technology's subsidiaries Clou Electronics and Hekang New Energy jointly appeared at the Munich Battery Energy Storage Exhibition and Solar Energy Exhibition
- Guoxin Sichen | Application of ferroelectric memory PB85RS2MC in power battery management, with a capacity of 2M
- Analysis of common faults of frequency converter
- In a head-on competition with Qualcomm, what kind of cockpit products has Intel come up with?
- Dalian Rongke's all-vanadium liquid flow battery energy storage equipment industrialization project has entered the sprint stage before production
- Allegro MicroSystems Introduces Advanced Magnetic and Inductive Position Sensing Solutions at Electronica 2024
- Car key in the left hand, liveness detection radar in the right hand, UWB is imperative for cars!
- After a decade of rapid development, domestic CIS has entered the market
- Aegis Dagger Battery + Thor EM-i Super Hybrid, Geely New Energy has thrown out two "king bombs"
- A brief discussion on functional safety - fault, error, and failure
- In the smart car 2.0 cycle, these core industry chains are facing major opportunities!
- The United States and Japan are developing new batteries. CATL faces challenges? How should China's new energy battery industry respond?
- Murata launches high-precision 6-axis inertial sensor for automobiles
- Ford patents pre-charge alarm to help save costs and respond to emergencies
- New real-time microcontroller system from Texas Instruments enables smarter processing in automotive and industrial applications
- CCS variable observer problem
- [NXP Rapid IoT Review] Rapid IoT Studio Simple Programming Step 4 Add Bluetooth RGB Light Control
- EEWORLD University - What is Dynamic Multi-Protocol Manager (DMM)?
- Ordered an MPLAB Snap
- The information on the varactor diode, laser pointer, supercapacitor, TF card and SD card on the list is all here!
- 【 Don't miss it! 9/10@Shenzhen】2019 WPI/TI Latest PoE Solutions Seminar
- Puzhong Technology 51 MCU Development Board v3.0 Dynamic Digital Tube Part
- STM32F103 timer clock not understood
- Why do clocks use 32.768K crystals?
- Problems and Solutions in TMS320F206 Simulation Debugging