Autonomous driving vehicles usually have multiple sensors installed as perception input sources, and first of all, the coordinate relationship between them needs to be determined. Therefore, sensor calibration is a basic requirement for autonomous driving. In general, the entire calibration work can be divided into two parts: internal parameter calibration and external parameter calibration. The internal parameters determine the mapping relationship inside the sensor, such as the focal length, eccentricity and pixel aspect ratio (+ distortion coefficient) of the camera, while the external parameters determine the conversion relationship between the sensor and an external coordinate system, such as attitude parameters (rotation and translation 6 degrees of freedom).
Sensor calibration is the basis for the robust performance of smart vehicles. The traditional and commonly used calibration method is to conduct electrical inspections on smart vehicles at the factory. Once the calibration is completed, its internal and external parameters will not be automatically optimized and updated after it is delivered to the customer.
As we all know, the entire intelligent driving perception field almost exclusively uses computer vision-related AI processing technologies, such as deep learning, artificial intelligence, and various data-driven optimizations.
Take lane line recognition, the most common perception, as an example. With the advent of the era of autonomous driving, the application scenarios that require lane line recognition are becoming more and more extensive, and the corresponding lane line recognition technology is becoming more and more important. The specific reasons are as follows:
First, it covers a wide range of scenarios. Lane recognition exists in most application scenarios of autonomous driving and is an indispensable basic algorithm for autonomous driving.
Second, as an important safety guarantee, lane line recognition can accurately identify roads and ultimately help machines make decisions, which is also an important guarantee for driving safety.
Third, in the field of high-precision map or light map construction, lanes are the primary key for road association of all high-precision map elements, so lane line elements are of paramount importance in high-precision maps, and lane line recognition algorithms are also crucial.
Based on this, autonomous driving has extremely high requirements for the accuracy of lane recognition results. We have found that in the actual recognition process, many Tier 1 or Tier 2 companies have more or less discovered some unfavorable perception results during actual road tests.
For example, after a vehicle is put on the road for a certain period of time after it is rolled off the production line, it is easy to have lane line jumps, inaccurate target perception and other results. In fact, these algorithm suppliers also have certain analysis and response capabilities for the source of such problems. Because most of these problems come from the projection matrix P or homography matrix H determined by the calibration of internal and external parameters. Usually, the optimization methods they use include the following:
1) If the two lane lines jump intermittently, it may be caused by the perception algorithm itself. In this case, consider preprocessing on the control side. The method is to use the two lane lines as the original input, and the Kalman filter predicts the output of the driving center line;
2) If one of the lane lines jumps intermittently, consider whether some internal and external parameter values of the sensor have undergone calibration deviation over time. The solution is to use the lane line and the target to calculate a better camera pitch value, and then add some parallel assumptions at the back end to solve this kind of problem well;
3) The long-term operation and maintenance-free advantages of the camera system can only be guaranteed by an automatic calibration method, which can update the camera parameters as needed. However, in the natural environment, interference can easily pose challenges to calibration. One possibility is to recalibrate the sensor using natural objects of known shapes. If the sensor is calibrated online in real time using the recognized static targets in the environment, a high-quality Pitch value can be obtained using optical flow or other advanced video tracking detection techniques.
01
Overview of Self-Calibration Methods
Common camera calibration methods require some special reference patterns, such as a chessboard, whose physical size is known in advance. However, these special patterns are not easy to obtain when the vehicle is on the road. In this case, the automatic calibration system needs to extract reference information from its environment.
In summary, the methods of automatic calibration can be classified into the following categories:
1) Extract vanishing points or road corners from the road virtual lines for online calibration.
2) Receive predefined feature points from other cars to build a corresponding model for obtaining traffic camera parameters.
3) Monitor camera calibration based on three vanishing points that define the vehicle flow.
4) Accomplish the same thing by targeting the walking character as a vertical line segment.
In fact, for the self-calibration method, the current strategy considered is to extract feature points from the road line for calibration, but it is impossible to assume that the width of the road line in different areas is the same. In addition, there may be defects along the contour of the road line due to wear. These pseudo-images and image changes will lead to certain calibration errors.
There are two solutions to the above problems. One approach is to design a structured road environment (a grid of known size drawn on the road) on the vehicle side, which is detected by the camera when the vehicle approaches. Another approach uses several markers placed on the hood of the car to quickly estimate the relative position and direction of the on-board camera. However, this may distract other drivers, assuming that the hood is within the camera's field of view, and if the hood is not flat, the performance of different vehicles may vary. Therefore, neither of the above two methods is suitable for real driving scenarios.
02
The optimized self-calibration scheme proposed in this paper
The focus of this paper is to study a new optimization scheme for real-time online calibration of sensors. The focus here is on a method based on identifying traffic signs in the environment (such as stop signs, speed limit signs and other common signs) and using them to recalibrate the camera. The method is implemented based on detection, geometry estimation, calibration and recursive update steps. The results of real-time calibration can clearly show its convergence and improved performance.
Unlike design patterns, stop signs are ubiquitous. The physical dimensions of stop signs are standardized by the Department of Transportation. Using traffic signs, especially stop signs, has three advantages over other reference information present in the urban environment:
Sufficient feature points (at least eight starting from the inner octagon of the stop sign) can be detected with sub-pixel accuracy.
Compared to other reference objects (e.g., road lines), the relevant geometric properties of stop signs remain unchanged because they are made of metal that is robust to external forces.
The vehicle slows down when approaching a stop sign, reducing image blur and rolling shutter effects.
Sensor calibration is fundamental for the robust performance of intelligent vehicles. In natural environments, disturbances can easily pose challenges to calibration. One possibility is to use natural objects of known shapes to recalibrate the sensors. Therefore, we propose an approach for automatic calibration using stop signs.
The entire calibration logic flow chart is as follows:
The detection described above focuses on the following important module processing.
1) Traffic sign detection:
For the case of real-time online calibration relying on road signs, it is necessary to detect the traffic sign information in the road in real time while driving. Currently, object detection has been a well-studied field with the help of convolutional neural networks (CNN). General detection architectures (such as YOLO, YOLOv3) can easily run in real time (45 Hz); while proposal-based architectures (such as Faster R-CNN, FPN) require more resources and usually take longer to run, and are usually better than the former in terms of accuracy, especially for small objects and complex scenes. In addition, instance segmentation methods that label object pixels can also be used to find stop sign bounding boxes.
2) Shape matching:
Shape matching is done by checking the similarity of the shapes of the two signs to be compared using a similarity metric. In particular, in our example of online calibration using a stop sign, the shape enclosed by the detected stop sign edge must be an octagon. Here, the polygon can be represented by a rotation function and the Lp distance can be measured. Of course, affine invariance can also be considered (in addition to rotation, translation and scale invariance).
The real-time online calibration system for smart cars can be divided into seven modules (as shown below).
The first five parts generate 2D-3D correspondence pairs of corner points from the city image sequence, and the last two parts are the last two steps to calculate the intrinsic parameters. Here we will explain the process separately.
1. Stop Sign Detection
The sign detection stage processes the image frame using the Mask R-CNN model with a ResNet-50 and Feature Pyramid Network backbone to generate a 2D bounding box (green box in Figure a above). The relatively low speed of the Mask R-CNN model does not affect our current non-real-time system because the overhead is mainly in the edge line fitting module. Therefore, Mask R-CNN has a higher stop sign detection rate compared to Faster R-CNN, which may improve the calibration accuracy of our system by providing more reference candidates.
Previous article:Silergy Automotive Grade Electronic Fuse SA21816
Next article:How to significantly improve the thermal efficiency of automobile engines?
Recommended ReadingLatest update time:2024-11-16 10:30
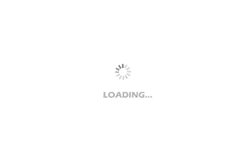
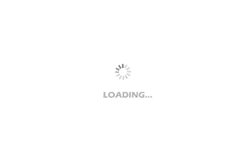
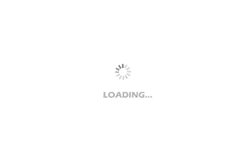
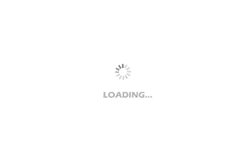
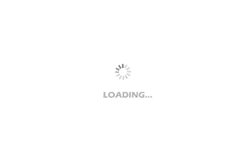
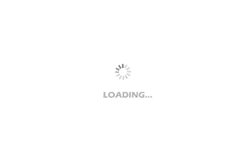
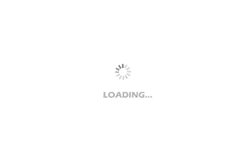
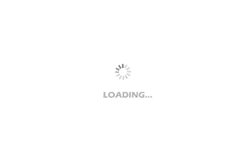
- Popular Resources
- Popular amplifiers
-
Analysis and Implementation of MAC Protocol for Wireless Sensor Networks (by Yang Zhijun, Xie Xianjie, and Ding Hongwei)
-
Introduction to Internet of Things Engineering 2nd Edition (Gongyi Wu)
-
西门子S7-12001500 PLC SCL语言编程从入门到精通 (北岛李工)
-
Modern Motor Control Technology (Wang Chengyuan, Xia Jiakuan, Sun Yibiao)
- Huawei's Strategic Department Director Gai Gang: The cumulative installed base of open source Euler operating system exceeds 10 million sets
- Analysis of the application of several common contact parts in high-voltage connectors of new energy vehicles
- Wiring harness durability test and contact voltage drop test method
- Sn-doped CuO nanostructure-based ethanol gas sensor for real-time drunk driving detection in vehicles
- Design considerations for automotive battery wiring harness
- Do you know all the various motors commonly used in automotive electronics?
- What are the functions of the Internet of Vehicles? What are the uses and benefits of the Internet of Vehicles?
- Power Inverter - A critical safety system for electric vehicles
- Analysis of the information security mechanism of AUTOSAR, the automotive embedded software framework
Professor at Beihang University, dedicated to promoting microcontrollers and embedded systems for over 20 years.
- Innolux's intelligent steer-by-wire solution makes cars smarter and safer
- 8051 MCU - Parity Check
- How to efficiently balance the sensitivity of tactile sensing interfaces
- What should I do if the servo motor shakes? What causes the servo motor to shake quickly?
- 【Brushless Motor】Analysis of three-phase BLDC motor and sharing of two popular development boards
- Midea Industrial Technology's subsidiaries Clou Electronics and Hekang New Energy jointly appeared at the Munich Battery Energy Storage Exhibition and Solar Energy Exhibition
- Guoxin Sichen | Application of ferroelectric memory PB85RS2MC in power battery management, with a capacity of 2M
- Analysis of common faults of frequency converter
- In a head-on competition with Qualcomm, what kind of cockpit products has Intel come up with?
- Dalian Rongke's all-vanadium liquid flow battery energy storage equipment industrialization project has entered the sprint stage before production
- Allegro MicroSystems Introduces Advanced Magnetic and Inductive Position Sensing Solutions at Electronica 2024
- Car key in the left hand, liveness detection radar in the right hand, UWB is imperative for cars!
- After a decade of rapid development, domestic CIS has entered the market
- Aegis Dagger Battery + Thor EM-i Super Hybrid, Geely New Energy has thrown out two "king bombs"
- A brief discussion on functional safety - fault, error, and failure
- In the smart car 2.0 cycle, these core industry chains are facing major opportunities!
- The United States and Japan are developing new batteries. CATL faces challenges? How should China's new energy battery industry respond?
- Murata launches high-precision 6-axis inertial sensor for automobiles
- Ford patents pre-charge alarm to help save costs and respond to emergencies
- New real-time microcontroller system from Texas Instruments enables smarter processing in automotive and industrial applications
- Power Switch Device Selection
- MSP430 MCU Development Record (9)
- Follow and comment to win gifts! Micron 2022 Taipei International Computer Show Keynote Speech Selection: Intelligent Edge and Smart Manufacturing Special
- Qorvo announced the acquisition of Active-Semi. Will Qorvo take off in areas such as 5G?
- Sun goods + 2 sets of Wei Dongshan suits
- I am currently debugging the display board and encountered a problem
- I drew a draft of the micro-bit
- [Bluesun AB32VG1 RISC-V board "meets" RTT] + unpacking and environmental installation
- Analog electronics elective test + DC and AC parameters
- The ADC example of the underlying driver code of esp32 is added to my own project and compiled unsuccessfully