2. Detect contour points by color segmentation
This module detects the contour points of the red inner octagon from the stop sign (see Figure b above). In the image preprocessing stage, the red part with threshold can be extracted using the color space conversion from RGB to HSV.
Then, on the HSV image, a conventional edge detection algorithm (such as the Canny/Devernay algorithm) is used to extract the edge, so as to obtain the contour points of the octagon with sub-pixel accuracy. Since the edge detection algorithm is very important in this article, the technical principles of the Canny algorithm and Devernay can be briefly explained here.
A. Canny algorithm
The Canny algorithm actually applies the first-order derivative of the Gaussian filter to image detection, so that the image gradient can be obtained, where the edge point is the local maximum value along the normal direction n.
The direction n orthogonal to the edge can be approximated by the image gradient:
In the above formula, G and I are Gaussian filter and image respectively, and * represents the convolution operator.
B. Devernay sub-pixel correction
Although the basic edge detection process can be achieved through the Canny algorithm, the edge points obtained are at the whole pixel accuracy level. For our system, the processing process requires sub-pixel accuracy, which can be improved by using the Devernay correction algorithm to improve the Canny algorithm. Assume that B is the pixel point obtained by the Canny algorithm (corresponding to the local gradient maximum in the normal direction n). The gradient of two adjacent points of B along n can be approximated by linear interpolation. Then, the sub-pixel position of the edge point is refined to the maximum value of the one-dimensional quadratic interpolation of all three points (along n).
Next, we need to link the contour points of the processed results. To achieve this goal, we need to group the above independent edge detection points into several chains. In order to ensure the uniqueness of the contour detection, we need to use the dense point detection method, and the one containing the most points will be selected as the contour of the inner octagon.
3. Edge line fitting
Although the overall contour of the octagon is detected as above, it is still necessary to rely on a certain edge line fitting algorithm to fit these contour points into a real octagon.
The operation process is to fit eight lines to a set of two-dimensional points X ∈ RN×2 on the stop sign contour above, and the estimation of each line will generate an edge of the octagon. One edge is estimated at a time by finding the line with the most "supporting points". When the distance from the point to the line is within a threshold (in this paper, the threshold is fixed to 0.5 pixel distance to ensure that sub-pixel is accurately estimated), the contour point is considered to "support" the estimation of the corresponding line.
The selection of contour lines is done by running the RANSAC algorithm on the original set of contour points. Note that the number of iterations is limited by N2 by going through all pairs. Once an edge is fitted, all supporting points are removed from the original set so that the same algorithm can be repeated to find different edges.
Combined with the probability p ∈ [0, 1), the probability that the final least squares line is the most supported line, the number of iterations K can be calculated according to the following formula:
where w = 1(8−i)2 , i ∈ {0, ..., 7} is the chance of selecting a point from the support set, assuming that there are few outliers outside the contour in X. Determining p involves considering the trade-off between estimation accuracy and running time. If accuracy is more important than computational efficiency, then p should be set as close to 1 as possible (e.g., 0.999).
4. Vertical search line refinement
The size of the gradient of the RGB/HSV type image can actually indicate the location of the octagon outline, where the color changes most abruptly in the neighborhood. Considering that smart cars may be driving at night or in scenes with insufficient visibility, the original point may deviate from the outline due to insufficient lighting, which may lead to misleading color segmentation in the HSV space. Therefore, line thinning is necessary, where the moved line can be adjusted back to the true octagon edge through local search.
Starting from the estimated line, we search for the local maximum gradient along the perpendicular direction of the line and rotate the line to align with it. In the case of underexposure/overexposure, the gradient peak at the edge may disappear. Therefore, introducing a refinement boundary can solve this problem well. Assuming that the refinement should be subtle, a line will be updated only when more than half of the S refined points are within the boundary.
The refined border can be parameterized by a percentage B ∈ [0, 1], which means that the refined point is at most 100% of the white border width away from the original position in the vertical direction. To estimate the width of the white border of the stop sign, the length of the estimated line segment can be multiplied by the width-to-length ratio of the inner polygon edge recorded in the traffic sign rule.
5. Intersection point estimation
The eight estimated lines have at most 28 intersections, of which only eight are valid octagon corners. A simple, effective and commonly used approach is to select intersections close to the line segment endpoints (for example, within a distance of 10 pixels). Then, any estimate with a corner count not equal to 8 is discarded.
For example, sorting the corner points in clockwise order (relative to the geometric center of the points) helps to obtain 2D-3D correspondences. In addition, the first corner can always be set to the top left corner of the character "S" because stop signs are usually upright.
In order to further verify the validity of the corner points, the Hausdorff distance method is used to ensure that the final set of eight points forms an octagon under affine transformation.
6. Planar Object Calibration
Usually such standard models are calibrated using the pinhole camera model. That is, a 3D point (X, Y, Z) in world coordinates is projected into the image as a pixel (u, v). (u, v) can be related to (X, Y, Z) by the following equation:
Where s is an unknown scalar, A ∈ R 3×3 is the camera intrinsic matrix (assuming no skew constraints). r1, r2, r3 are the columns of the rotation matrix, and t is the translation vector. They are often called camera extrinsic parameters because they have the function of transforming world coordinate points into the camera coordinate system.
During calibration, if the 3D coordinate system is fixed to a flat object such as a chessboard or a stop sign, the Z component in Equation 3 is always zero, resulting in a simplified equation:
We use M = (X, Y, 1)T and m = (u, v, 1)T to represent the position of a point on a plane object and its position in the image, respectively. Their relationship is defined by the homography matrix H as follows:
Obviously, H is a 3 × 3 matrix up to a scaling factor. The homography matrix H can be computed using N ≥ 4 points.
In our system, after detecting the 8 corner points of the stop sign, H can be represented by H = [h1 h2 h3]. From formula 5, we can get:
Based on the knowledge that r1 and r2 are orthogonal, we obtain the two constraints required to calculate the intrinsic parameter matrix A as follows:
In this way, the two constraints required to calculate the intrinsic parameter matrix A are obtained.
In our system, the principal point is assumed to be fixed at the center of the image plane and only the focal lengths fx and fy are calculated and updated iteratively, so for the chessboard calibration we also need to enforce this assumption for comparison purposes.
The closed-form solution can be improved by maximum likelihood inference: the original calibration needs to be used as a nonlinear optimization problem for initial estimation, which can also be solved using the Levenberg-Marquardt algorithm. Currently, this part is not included in our system.
7. Time update of Kalman filter calibration
The optimization update target here is that some other noise may be generated over time, so it is necessary to integrate the camera intrinsic parameter matrix through continuous optimization. We generally assume that the inference process will introduce some standard Gaussian noise, so we can use Kalman filters with different noise covariance matrices for optimization and update.
The standard Kalman filter prediction formula is as follows:
where xt, xt+1 are the camera intrinsic parameter vectors estimated at different times. A basic assumption of the study is that if the intrinsic parameters are assumed to be constant in the short-term estimation, then F can become the identity matrix.
At this point, the entire update step is performed by the following formula:
The process noise and measurement noise δ are Gaussian random vectors with zero mean. Their covariance is represented by the (diagonal) matrices Q = E( T ) and R = E(δδT ), respectively. Q specifies how much we believe the intrinsic parameters to vary over time and should be lower for shorter estimation periods. R determines how much noise is in one estimate. Intuitively, this measurement noise should decrease from a high value as the process progresses, because the more images used, the more accurate the calibration will be.
03
in conclusion
Advanced driver assistance systems have very strict requirements on the robust performance of their sensors, because this is the key to whether the system can achieve accurate environmental perception. From the current development trend, the most promising sensing mode is the imaging mode, because low-cost and rich environmental information can be calculated from the image. Since camera systems are often used for distance perception, speed reasoning and target detection. For such applications, camera parameters are usually crucial for reconstructing part of the scene and affecting the measurement accuracy. The accuracy of the parameters often comes from the precise calibration and adaptive optimization process of the original camera. However, when the camera is installed on the vehicle, the inherent parameters of the camera due to mechanical vibration caused by road bumps or temperature fluctuations caused by weather will change. Therefore, it will also be a challenge to repeatedly calibrate the camera for long-term use.
Previous article:Silergy Automotive Grade Electronic Fuse SA21816
Next article:How to significantly improve the thermal efficiency of automobile engines?
Recommended ReadingLatest update time:2024-11-16 12:54
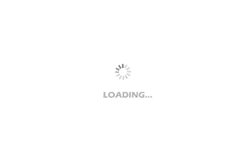
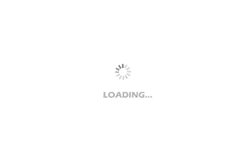
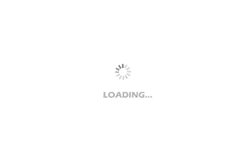
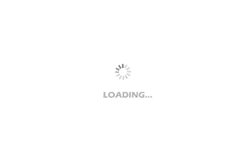
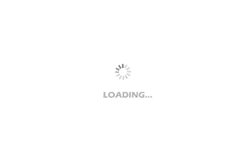
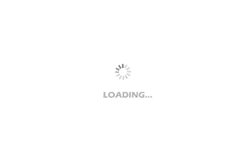
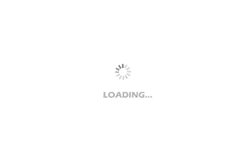
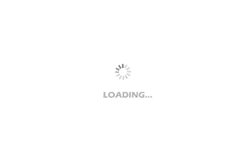
- Popular Resources
- Popular amplifiers
-
Analysis and Implementation of MAC Protocol for Wireless Sensor Networks (by Yang Zhijun, Xie Xianjie, and Ding Hongwei)
-
Introduction to Internet of Things Engineering 2nd Edition (Gongyi Wu)
-
西门子S7-12001500 PLC SCL语言编程从入门到精通 (北岛李工)
-
Modern Motor Control Technology (Wang Chengyuan, Xia Jiakuan, Sun Yibiao)
- Huawei's Strategic Department Director Gai Gang: The cumulative installed base of open source Euler operating system exceeds 10 million sets
- Analysis of the application of several common contact parts in high-voltage connectors of new energy vehicles
- Wiring harness durability test and contact voltage drop test method
- Sn-doped CuO nanostructure-based ethanol gas sensor for real-time drunk driving detection in vehicles
- Design considerations for automotive battery wiring harness
- Do you know all the various motors commonly used in automotive electronics?
- What are the functions of the Internet of Vehicles? What are the uses and benefits of the Internet of Vehicles?
- Power Inverter - A critical safety system for electric vehicles
- Analysis of the information security mechanism of AUTOSAR, the automotive embedded software framework
Professor at Beihang University, dedicated to promoting microcontrollers and embedded systems for over 20 years.
- Innolux's intelligent steer-by-wire solution makes cars smarter and safer
- 8051 MCU - Parity Check
- How to efficiently balance the sensitivity of tactile sensing interfaces
- What should I do if the servo motor shakes? What causes the servo motor to shake quickly?
- 【Brushless Motor】Analysis of three-phase BLDC motor and sharing of two popular development boards
- Midea Industrial Technology's subsidiaries Clou Electronics and Hekang New Energy jointly appeared at the Munich Battery Energy Storage Exhibition and Solar Energy Exhibition
- Guoxin Sichen | Application of ferroelectric memory PB85RS2MC in power battery management, with a capacity of 2M
- Analysis of common faults of frequency converter
- In a head-on competition with Qualcomm, what kind of cockpit products has Intel come up with?
- Dalian Rongke's all-vanadium liquid flow battery energy storage equipment industrialization project has entered the sprint stage before production
- Allegro MicroSystems Introduces Advanced Magnetic and Inductive Position Sensing Solutions at Electronica 2024
- Car key in the left hand, liveness detection radar in the right hand, UWB is imperative for cars!
- After a decade of rapid development, domestic CIS has entered the market
- Aegis Dagger Battery + Thor EM-i Super Hybrid, Geely New Energy has thrown out two "king bombs"
- A brief discussion on functional safety - fault, error, and failure
- In the smart car 2.0 cycle, these core industry chains are facing major opportunities!
- The United States and Japan are developing new batteries. CATL faces challenges? How should China's new energy battery industry respond?
- Murata launches high-precision 6-axis inertial sensor for automobiles
- Ford patents pre-charge alarm to help save costs and respond to emergencies
- New real-time microcontroller system from Texas Instruments enables smarter processing in automotive and industrial applications
- Things to note when using C/C++ to write programs based on TMS320 series DSP
- Generate sine wave data using microcontroller DAC and C language
- RISC-V RVB2601 First Experience--Section 4--Sensor Module Interface
- Based on micropython-1.9.4 ESP8266 motor control firmware
- MATLAB reads txt file data and processes it
- Easy to use, LiChuang EDA. Haha!
- [Start at 10:30] Interpretation of TI's latest smart lock, visual doorbell, and network camera solutions, and recommendations for its core components
- C6678 multi-core DSP development - connected domain marking of vlib application
- Nanjing MIIT certified enterprise [urgently hiring IC engineers with high salary]
- CCS 6 New TMS320F2812 Project