Machine vision has grown significantly in recent years. In 2015, the global machine vision market size was about 4.2 billion US dollars, an increase of 10.5%. In 2015, the market size of my country was about 350 million US dollars, but the growth rate was the highest in the world, about 22.2%. From 2016 to 2020, machine vision continued to maintain a growth rate of about 20%, far higher than the global average of 8.4%. Among them, semiconductor and electronic manufacturing accounted for about 46.4% of machine vision, while automobiles and pharmaceuticals accounted for 10.9% and 9.7% respectively.
According to the communication with many parties in the industry, the current market size of machine vision is about tens of billions, but only 5% of the industry applications should and do use machine vision, so the market space is huge. The top priority for the development of machine vision is to find more scenarios where it can be applied and further promote its popularization. This requires collaboration among the industry chain, truly hitting the pain points of the industry and embracing specific needs. At the same time, it also requires cost-effective technologies and solutions.
Tuyang
How to remove the obstacles to the large-scale popularization of 3D machine vision?
How to popularize 3D machine vision on a large scale? Fei Zeping, CEO of Shanghai Tuyang Information Technology Co., Ltd., believes that the most critical issue at present is the hardware problem. Only by solving the fundamental hardware problem can the future software business opportunities be real.
The key obstacles to the popularization of 3D machine vision are currently mainly in the hardware aspect, including the following three points:
First, the core hardware specifications, including optical accuracy, resolution, range, and electrical speed, interface, transmission, etc.; second, the physical indicators of the hardware, including size, power consumption, structure, etc., as well as the adaptability and stability of working conditions. Third, the system implementation cost, including the camera price, host computer cost, software cost, and use and maintenance cost.
Traditional machine vision has been developing steadily for many years and is currently at a stage where the development of hardware and software is basically matched. Currently, more than 95% of the needs can be solved by hardware + algorithms. Only in scenes such as appearance inspection and defect detection, deep learning is needed due to the presence of a certain degree of randomness. Although deep learning will eventually be adopted in the future, the basic hardware must be ahead of the software.
Machine vision is a mature industry, but its development scale and speed have lagged behind expectations in recent years. Fei Zeping believes that the main reasons are: first, the user objects belong to traditional industries, and the industry's own prosperity index and transformation cycle have a certain impact; in addition, for traditional industries, technology upgrades, cost reduction and efficiency improvement, refined management, etc., are all itchy points rather than pain points in the industry.
So, what are the pain points of the industry?
Fei Zeping believes that from the perspective of hardware prices and system costs, it is necessary to break through the traditional two-year investment recovery cycle calculation dilemma, and gradually form large-scale popular applications from a small number of essential nodes, which is more focused on the scene of machine replacement.
The common knowledge of two-year recovery costs can no longer leverage industry demand, and only a period of one year will really make small and medium-sized enterprises willing to pay. The maximization of technological value should be achieved by improving the productivity level and economic benefits of the industry on a large scale, and it must be profitable by bringing value to customers. This is a relatively difficult process and needs to be slowly promoted.
From the objective status quo of machine vision development, there is no shortage of demand, but only 10% of all demand is available for good products that can be put into use and bring real economic benefits. How can we break this "curse" and promote more practical applications?
"It is most urgent to solve the ultimate cost-effectiveness of core components and make the system mature, stable and reliable. At present, microscopic high-precision cameras only account for about 5% of machine vision applications. As long as the cost-effectiveness is maximized, more than 90% of the application requirements in industrial machine vision can be met." Fei Zeping said, "This is reflected in the applicability and degree of standardization of the product on the one hand, and in the cost reduction and efficiency increase that the machine can bring on the other. This also requires the real rigid demand for visual applications to gradually emerge. For example, when labor costs become a major problem, the demand for machines will turn into a demand."
How to achieve the ultimate cost-effectiveness? During the conversation, Fei Zeping conveyed a simple pragmatic idea that there is no need to use 3D if it can be solved with 2D vision. For industrial cameras, the largest cost units are image sensor chips, lenses, computing units, CPUs or GPUs, etc. Tuyang has reduced the high computing power cost by dozens of times by jointly optimizing optics, electronics and software, and implementing efficient FPGA hardening of the core algorithm.
The logistics industry is Tuyang’s largest target market at present, and it is currently focusing on the new blue ocean of volume measurement. Since the transportation cost, which is the key to determining the gross profit of logistics, is determined by the volume, there is a strong demand for volume measurement.
By targeting the core of demand and matching it with good products and services, we can firmly grasp the market and take the lead in building a competitive "moat" - this is a major feature of Tuyang in choosing landing scenarios.
In terms of business model, Tuyang is a to B model that directly faces system integrators and equipment manufacturers. Fei Zeping further explained that he hopes to enhance the value of the system through the industry chain. For Tuyang itself, the price of standardized core components can be relatively transparent, while inventions, expert knowledge, technical services and other links that create value for customers and partners must have reasonable returns. Tuyang hopes to lower the threshold for customers to use through highly standardized hardware and continuously integrated algorithm resources, thereby further expanding the application coverage.
In general, the machine vision ecosystem is currently relatively mature. From the perspective of the supply chain, it is not special compared to the consumer market, and its development is relatively complete. But one significant difference is that the software algorithms in different industries of industrial applications and even different customer sites in the same industry are very diverse. This poses a great challenge to the capabilities of developers, mainly including the capabilities of open source algorithms, the level and number of practitioners, etc., which directly affects the coverage of applications. This also verifies what was said at the beginning, that the more machine vision develops, the greater the proportion of software in each landing application will be. Tuyang is committed to standardizing hardware and integrating the standardized software at the same time, lowering the threshold for system integrators and equipment suppliers, thereby promoting rapid popularization.
Hikvision
How does AI enable smart manufacturing?
How does AI empower smart manufacturing? Cai Hua, general manager of Hikvision Robotics Technology Co., Ltd. in North China, believes that there are three key links: first, strong perception capabilities, which require machine vision for perception; second, the data that is perceived and collected is managed through a platform to achieve one-stop service; and third, scenario-based AI.
To truly realize intelligence requires a huge system engineering. In 2017, Hikvision launched the AI Cloud platform to deeply integrate the needs of intelligent manufacturing. The platform is divided into three layers. The first layer is the infrastructure layer, which emphasizes the multi-dimensional perception and collection of the front end. The second layer is the resource layer, also called the edge layer. After the perception of part of the data at the front end is completed, it will be managed in an edge manner, and the unstructured data at the front end will be stored in the middle layer to become semi-structured data; the top layer is the service layer, which is also the center of the entire platform. It embodies the training and application services of the algorithm, corresponding to the business layer. Therefore, the basic architecture of the entire platform emphasizes the combination of cloud and edge.
From the perspective of implementation, the multi-dimensional sensing system collected by the front end can aggregate, store and process data, and then use edge computing to reduce the pressure on the center. There are three trends worth noting:
The first significant trend is that the intelligence level of the front end is constantly improving, and the pressure on the center is relieved through edge computing. The front end is no longer limited to data collection, and will also perform some structured processing work. There are already many smart cameras and smart sensors in industrial applications, which perform some edge computing work. They are more suitable for applications such as: judging right or wrong/existence, or measuring perceived dimensions, etc., to perform the first step of reading or recognition. At present, deep learning at the front end is a method that many companies are trying, that is, putting the front-end algorithm into an achievable embedded device for reading/recognition, thereby reducing the pressure on the center.
Previous article:Alibaba’s thorny road to artificial intelligence: What does it feel like to sit on a mountain of gold and eat steamed buns?
Next article:Artificial intelligence is sweeping the technology world in the form of a revolution. What hidden dangers still exist?
Recommended ReadingLatest update time:2024-11-16 11:25
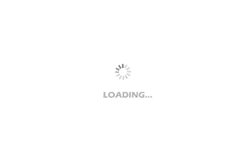
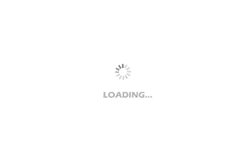
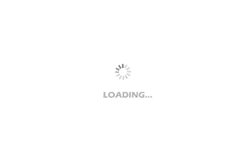
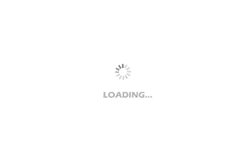
- Popular Resources
- Popular amplifiers
-
Introduction to Internet of Things Engineering 2nd Edition (Gongyi Wu)
-
Permanent Magnet Synchronous Motor Speed Control System Control Technology (by Hou Limin and Wang Wei)
-
Implementing a Deep Learning Framework with Python (Zhang Juefei, Chen Zhen)
-
Yousan AI Visual Algorithm Engineer Growth Guide
- Huawei's Strategic Department Director Gai Gang: The cumulative installed base of open source Euler operating system exceeds 10 million sets
- Analysis of the application of several common contact parts in high-voltage connectors of new energy vehicles
- Wiring harness durability test and contact voltage drop test method
- Sn-doped CuO nanostructure-based ethanol gas sensor for real-time drunk driving detection in vehicles
- Design considerations for automotive battery wiring harness
- Do you know all the various motors commonly used in automotive electronics?
- What are the functions of the Internet of Vehicles? What are the uses and benefits of the Internet of Vehicles?
- Power Inverter - A critical safety system for electric vehicles
- Analysis of the information security mechanism of AUTOSAR, the automotive embedded software framework
Professor at Beihang University, dedicated to promoting microcontrollers and embedded systems for over 20 years.
- Innolux's intelligent steer-by-wire solution makes cars smarter and safer
- 8051 MCU - Parity Check
- How to efficiently balance the sensitivity of tactile sensing interfaces
- What should I do if the servo motor shakes? What causes the servo motor to shake quickly?
- 【Brushless Motor】Analysis of three-phase BLDC motor and sharing of two popular development boards
- Midea Industrial Technology's subsidiaries Clou Electronics and Hekang New Energy jointly appeared at the Munich Battery Energy Storage Exhibition and Solar Energy Exhibition
- Guoxin Sichen | Application of ferroelectric memory PB85RS2MC in power battery management, with a capacity of 2M
- Analysis of common faults of frequency converter
- In a head-on competition with Qualcomm, what kind of cockpit products has Intel come up with?
- Dalian Rongke's all-vanadium liquid flow battery energy storage equipment industrialization project has entered the sprint stage before production
- Allegro MicroSystems Introduces Advanced Magnetic and Inductive Position Sensing Solutions at Electronica 2024
- Car key in the left hand, liveness detection radar in the right hand, UWB is imperative for cars!
- After a decade of rapid development, domestic CIS has entered the market
- Aegis Dagger Battery + Thor EM-i Super Hybrid, Geely New Energy has thrown out two "king bombs"
- A brief discussion on functional safety - fault, error, and failure
- In the smart car 2.0 cycle, these core industry chains are facing major opportunities!
- The United States and Japan are developing new batteries. CATL faces challenges? How should China's new energy battery industry respond?
- Murata launches high-precision 6-axis inertial sensor for automobiles
- Ford patents pre-charge alarm to help save costs and respond to emergencies
- New real-time microcontroller system from Texas Instruments enables smarter processing in automotive and industrial applications
- Regarding MOS tube driver IC, can it only work in PWM mode?
- PCB printed circuit board design technology and practice
- [Top Micro Smart Display Screen Review] 1. Screen interface introduction and power consumption test and serial communication test
- Temperature Problems Solved for You (III) High-Performance Processor Mold Temperature Monitoring
- AD13 This box can't be found
- I wish you all success in the competition and good results!
- [NXP Rapid IoT Review] Week 1: Get familiar with kit resources
- Looking to buy ATSAMC21 series or ATSAME70 series evaluation board
- Solve the process of WIN10 being constantly woken up
- bigbat's orcad study notes