L2.5 and L2.9 have been put into mass production, and L3 and limited-scenario L4 mass production has become an important goal for OEMs in the next stage. In March 2022, the National Highway Traffic Safety Administration (NHTSA) of the United States announced a historic decision - autonomous driving car manufacturers no longer need to equip fully autonomous vehicles with manual driving control systems. In the future, the United States is expected to introduce more important policies in the field of autonomous driving to guide L3/L4 autonomous driving on the road.
Against this backdrop, ADAS/AD autonomous driving chips are also experiencing a wave of upgrades, with many chip manufacturers having launched or planning to launch high-computing chips. In January 2022, Mobileye launched the EyeQ® Ultra™ system-on-chip at CES, which uses a 5-nanometer process and has a single-chip computing power of 176 TOPS. Although it is not as powerful as competitors such as NVIDIA and Qualcomm, its high cost-performance ratio and high energy efficiency may still be favored by OEMs.
Mobileye Autonomous Driving SoC Product Line
Source: 2022 ADAS/AD Autonomous Driving Chip Industry Research Report
In addition to stacking computing power, self-developed core IP is the focus of competition among major SoC manufacturers
SoC chips are mostly heterogeneous designs, including different computing units such as GPU, CPU, acceleration core, NPU, DPU, ISP, etc. Generally speaking, chips cannot be simply evaluated by computing power, but also need to take into account chip bandwidth, peripherals, memory, energy efficiency, cost, etc. At the same time, the development tool chain of SoC chips is crucial, and only by forming a developer ecosystem can long-term sustainable competitiveness be built.
In chip design, the configuration of heterogeneous IP is very important. Autonomous driving SoC chip manufacturers are constantly strengthening the research and development of core IP to maintain key competitiveness. Take NVIDIA as an example. The head of NVIDIA upgraded NVIDIA's existing GPU-based product line to a "three-core" strategy of "GPU+CPU+DPU":
GPU: NVIDIA has a huge advantage in GPU and image processing derived from GPU;
DPU: Nvidia acquired Israeli chip company Mellanox for $7 billion and launched the Bluefield DPU chip, which has evolved to the third generation. DPU is a programmable electronic component that has the versatility and programmability of a central processing unit (CPU) and is dedicated to processing network data packets, storage requests or analysis requests for efficient operation;
In terms of CPU, Nvidia launched the acquisition of IP manufacturer ARM, which was also an extension of Nvidia's "three-core" strategy, but ultimately failed. However, Nvidia still launched the Grace chip based on the ARM architecture, which is aimed at large-scale AI and high-performance computing in data centers. Nvidia's next-generation Atlan SoC also uses the ARM architecture.
Nvidia Bluefield DPU chip evolution path
Source: Internet
As for domestic manufacturers, Heizhima Intelligence has launched two core IPs developed independently - the NeurallQ ISP image signal processor and the deep neural network algorithm platform DynamAI NN engine.
Black Sesame A1000 autonomous driving SoC
Source: Internet
Cross-domain integration and central computing platform chips will lead the evolution of vehicle EEA architecture
The evolution trend of vehicle electronic and electrical architecture is "distributed architecture-domain concentration-cross-domain integration-central computing platform". Tesla's latest version of Model X has achieved a certain degree of central cross-domain integrated computing function. The Model X's on-board central computing platform includes two FSD chips, an AMD Ryzen CPU chip and an AMD RDNA2 GPU. The FSD chip and AMD CPU/GPU chip communicate through the PCIe interface and are isolated from each other.
Looking further, integrating multiple chips such as CPU, GPU, FSD into a SoC chip through Chiplet technology will further shorten the chip communication delay. It is reported that Tesla is working with Samsung Electronics to develop 5nm chips to achieve autonomous driving and cockpit SoC chip integration.
Judging from the trends of industry giants such as NVIDIA and Qualcomm, they have all begun to plan for the cross-domain integration of autonomous driving and cockpits. For example, NVIDIA has launched the DRIVE Concierge and DRIVE Chauffeur software solutions, which are aimed at smart cockpits and autonomous driving respectively. The DRIVE IX software stack can realize the integration of cabin algorithms. Based on powerful software stack tools, NVIDIA may be able to achieve single-chip control of autonomous driving and smart cockpits at the same time.
As for domestic SoC manufacturers, in February 2022, Horizon announced that it will cooperate with United Electronics to achieve mass production of cross-domain integrated in-vehicle computing platforms.
Cross-domain integration/central computing layout of autonomous driving SoC manufacturers
Source: 2022 ADAS/AD Autonomous Driving Chip Industry Research Report
SoC manufacturers accelerate the deployment of autonomous driving AI data training
Autonomous driving data sets are crucial for training deep learning models and improving algorithm reliability. SoC manufacturers have launched their own AI training chips and supercomputing platforms. Tesla has launched the AI training chip D1 and the "Dojo" supercomputing platform, which will be used to train Tesla's autonomous driving neural network.
Not only that, training algorithm model products are becoming increasingly important, including 2D labeling, 3D point cloud labeling, 2D/3D fusion labeling, semantic segmentation, target tracking, etc., such as NVIDIA Drive Sim autonomous driving simulation platform and Horizon's "Eddie" data closed-loop training platform.
Foreign chip manufacturers:
Tesla launched the Dojo supercomputing training platform: using Tesla's self-developed 7nm AI training chip D1, relying on a large customer base to collect autonomous driving data, so as to achieve model training for deep learning systems. Currently, Tesla Autopilot mainly uses 2D images + annotations for training and algorithm iteration. Through the Dojo supercomputing platform, Autopilot can be trained in the form of 3D images + timestamps (4D Autopilot system). The 4D Autopilot system will have predictability and mark the 3D movement trajectory of road objects to enhance the reliability of the autonomous driving function;
NVIDIA launched an autonomous driving simulation platform: Drive Sim Omniverse Replicator, an engine that generates synthetic data with correct annotations for training AI networks. NVIDIA also has the most powerful training processor at this stage, NVIDIA A100;
Mobileye's REM's map data has covered the world. In China, Mobileye has established a joint venture with China's Tsinghua Unigroup to solve compliance issues in map data collection in China. At the same time, Intel acquired Moovit to enhance REM's strength and data differentiation, and extend traditional high-definition map data from the road side to the user side, from perceptual redundancy for assisted autonomous driving to improving the efficiency of path planning. Intel has launched its self-developed top AI chip Ponte Vecchio. Ponte Vecchio's technology will also be extended to Mobileye's EyeQ6 (expected to be launched in 2023). In the fields of AI and servers, Intel will challenge Nvidia's position with CO-EMIB technology.
Domestic chip manufacturers:
To solve the long-tail problem of autonomous driving, Horizon Robotics has built a complete data closed-loop platform to iterate algorithms and improve system capabilities. Horizon Robotics launched the "Aidi" data closed-loop training platform;
Huawei launched the "Huawei Octopus" autonomous driving open platform, focusing on the four most critical elements of autonomous driving development - hardware, data, algorithms and high-precision maps, to build an open platform with data as the core to drive the closed-loop iteration of autonomous driving. At the same time, Huawei Ascend 910 is also the world's top AI training chip, directly benchmarking NVIDIA A100, and launched the AI training cluster Atlas 900.
Data training products of some autonomous driving SoC chip manufacturers
Source: 2022 ADAS/AD Autonomous Driving Chip Industry Research Report
The world's leading autonomous driving AI training chips include: Intel Ponte Vecchio, NVIDIA A100, Tesla D1, Huawei Ascend 910, Google TPU (v1, v2, v3), Cerebras Wafer-Scale Engine, Graphcore IPU, etc.
Comparison of some autonomous driving AI training chip products
Source: 2022 ADAS/AD Autonomous Driving Chip Industry Research Report
Previous article:Andes Technology and IAR Systems Join Forces to Help Leading Automotive Chip Designers Accelerate Time-to-Market
Next article:Yuanrong Qixing adopts NVIDIA Drive Orin chip to create automotive-grade solutions for L4 autonomous driving pre-installation mass production
Recommended ReadingLatest update time:2024-11-16 21:53
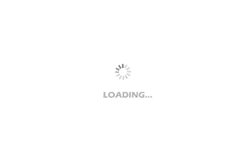
- Popular Resources
- Popular amplifiers
- Car key in the left hand, liveness detection radar in the right hand, UWB is imperative for cars!
- After a decade of rapid development, domestic CIS has entered the market
- Aegis Dagger Battery + Thor EM-i Super Hybrid, Geely New Energy has thrown out two "king bombs"
- A brief discussion on functional safety - fault, error, and failure
- In the smart car 2.0 cycle, these core industry chains are facing major opportunities!
- The United States and Japan are developing new batteries. CATL faces challenges? How should China's new energy battery industry respond?
- Murata launches high-precision 6-axis inertial sensor for automobiles
- Ford patents pre-charge alarm to help save costs and respond to emergencies
- New real-time microcontroller system from Texas Instruments enables smarter processing in automotive and industrial applications
- Innolux's intelligent steer-by-wire solution makes cars smarter and safer
- 8051 MCU - Parity Check
- How to efficiently balance the sensitivity of tactile sensing interfaces
- What should I do if the servo motor shakes? What causes the servo motor to shake quickly?
- 【Brushless Motor】Analysis of three-phase BLDC motor and sharing of two popular development boards
- Midea Industrial Technology's subsidiaries Clou Electronics and Hekang New Energy jointly appeared at the Munich Battery Energy Storage Exhibition and Solar Energy Exhibition
- Guoxin Sichen | Application of ferroelectric memory PB85RS2MC in power battery management, with a capacity of 2M
- Analysis of common faults of frequency converter
- In a head-on competition with Qualcomm, what kind of cockpit products has Intel come up with?
- Dalian Rongke's all-vanadium liquid flow battery energy storage equipment industrialization project has entered the sprint stage before production
- Allegro MicroSystems Introduces Advanced Magnetic and Inductive Position Sensing Solutions at Electronica 2024
- Car key in the left hand, liveness detection radar in the right hand, UWB is imperative for cars!
- After a decade of rapid development, domestic CIS has entered the market
- Aegis Dagger Battery + Thor EM-i Super Hybrid, Geely New Energy has thrown out two "king bombs"
- A brief discussion on functional safety - fault, error, and failure
- In the smart car 2.0 cycle, these core industry chains are facing major opportunities!
- The United States and Japan are developing new batteries. CATL faces challenges? How should China's new energy battery industry respond?
- Murata launches high-precision 6-axis inertial sensor for automobiles
- Ford patents pre-charge alarm to help save costs and respond to emergencies
- New real-time microcontroller system from Texas Instruments enables smarter processing in automotive and industrial applications
- EEWORLD University ----3
- What development environment is used for bare metal programming of imax6ull
- SparkRoad serial port to localbus to achieve command control
- EEWORLD University Hall----Live Replay: STMicroelectronics SiC Products and Industrial Application Guide
- Pre-registration for the prize-winning live broadcast | Explore the high-tech of network cameras with Infineon
- msp430f149 baud rate setting
- Topmicro Smart Screen: Remote update tools download
- According to this IPC-7351 software, how to select the pad type.
- Changed the layout again
- National Award-winning Works: Temperature Automatic Control System