Is it a bit presumptuous to design an autonomous driving chip to challenge Mobileye and Nvidia?
Not necessarily. Currently, the design of autonomous driving system chips, or SoCs, is mostly done in a building block manner. Various third-party IPs are the building blocks. As long as they are properly matched, it is still possible to challenge Mobileye and NVIDIA. The key lies not in technology, but in continuous and massive capital injection. This is a marathon race, and perseverance to the end is victory.
Mobileye's advantages are hardware and software integration, the shortest development cycle, the lowest development cost, and mature and stable technology. Its disadvantages are obvious product homogeneity, which cannot highlight the characteristics of vehicle manufacturers. The MIPS instruction set makes its system closed and has poor upgrade capabilities, which cannot adapt to the rapid evolution of sensor technology, especially the emergence of a large number of lidars.
NVIDIA's advantage is that its AI computing power is extremely powerful, with sufficient computing power redundancy to adapt to various algorithms and meet the algorithm evolution in the next 3-6 years. The GPU's floating-point computing power is also very powerful, with sufficient computing power redundancy and strong visual perception capabilities. NVIDIA provides a complete software stack, and the system is a bit closed, but the computing power is very powerful, so vehicle manufacturers can still make their own characteristics. The disadvantage is that the GPU occupies a large area of the bare crystal, and the hardware cost remains high.
In actual autonomous driving, there is much hype but little action. In 2017 and 2018, many technology companies or vehicle manufacturers vowed to launch L4-level vehicles in 2020 or 2021, without steering wheels and brake pedals, but in the end, nothing came of it. In 2021, many manufacturers launched mass-produced vehicles claiming to be L3/L4, but annual sales are unlikely to exceed a thousand. The main reason is that the cost-effectiveness is too low. The so-called L3/L4 is an enhanced version of adaptive cruise control or automatic following in traffic jams, but the cost is very high. At present, the technical conditions of L3/L4 are far from mature and have not reached the point of mass production. For example, in the field of perception, there are challenges such as the sudden appearance of stationary targets, non-standard obstacles, low-profile detection and recognition of infants and children, and rapid changes in light intensity, as well as the decision-making field of allowing or not allowing congestion. In addition, basic conditions are not available, such as high-precision maps and positioning, and V2X for traffic lights.
In the next 10 years, L2+ will still be the mainstream of the market, accounting for at least 90% of the market. High AI computing power is useless in the L2+ field. The so-called AI computing power is just a convolution accelerator, just a function of visual classification. When encountering sudden static targets, non-standard obstacles, and low targets such as infants and children, even with the highest AI computing power, accidents will still occur. However, the promotion of hardware manufacturers has made consumers mistakenly believe that high computing power means high-level autonomous driving.
Improving safety is not something that can be achieved by simply increasing AI computing power. LiDAR or stereo binocular sensors that can provide native 3D perception can improve safety. At the same time, it must also be based on traditional explainable and predictable algorithms to improve safety. Deep learning is a black box. It is impossible to predict the results, explain, or improve safety. Stereo binocular technology is too difficult to master. Only Mercedes-Benz and Toyota can do it. This is the result of more than ten years of internal talent training. Most companies can only choose LiDAR.
The laser radar's demand for SoC computing resources can be met by the CPU, avoiding the use of high-cost GPUs.
Image source: Internet
LiDAR can measure the distance to an object through laser reflection. Since the vertical angle of the laser is fixed, denoted as a, we can directly calculate the z-axis coordinate as sin(a)*distance. From cos(a)*distance, we can get the projection of distance on the xy plane, denoted as xy_dist. While recording the distance of the reflection point, LiDAR also records the horizontal angle b of the current LiDAR rotation. Based on a simple set transformation, we can get the x-axis coordinate and y-axis coordinate of the point as cos(b)*xy_dist and sin(b)*xy_dist respectively.
Velodyne VLP-16 Data Pack
Image source: Internet
The data processing of LiDAR consists of two parts. The first part is coordinate transformation, including the transformation between polar coordinates and rectangular coordinates XYZ, and the transformation between the LiDAR coordinate system and the vehicle coordinate system, which mainly involves trigonometric function transformation. The second part is point cloud registration. Preprocessing such as noise removal can be considered as part of point cloud registration.
In the early days, multiplication and floating-point computing resources were extremely scarce, so J. Volder proposed a fast algorithm in 1959, called CORDIC (COordinate Rotation DIgital Computer) coordinate rotation digital calculation algorithm. This algorithm can calculate the values of common trigonometric functions, such as Sin, Cos, Sinh, Cosh, etc., using only shift and addition and subtraction operations. J. Walther further improved this algorithm in 1974, making it possible to calculate a variety of transcendental functions, further expanding the application of the Cordic algorithm. Because the Cordic algorithm only uses shift and addition, it is easy to implement with the CPU. The Cordic algorithm was first used in navigation systems, so that the rotation and orientation operations of vectors do not require complex operations such as looking up trigonometric tables, multiplication, square root, and inverse trigonometric functions.
CORDIC uses continuous rotation to find the corresponding sine and cosine values, which is an approximate solution. The rotation angle is very particular, and the angle of each rotation must make the tangent value approximately equal to 1/(2^N). The purpose of the rotation is to make the Y axis approach 0. Accumulate the angle of each rotation, that is, the sum of the rotation angles is the tangent value. For example, if the Y axis rotates 45 degrees, the value decreases by 1/2, and then rotates 26.56505°, and then decreases by 1/4, and then rotates by an angle of 14.03624º, and then decreases by 1/8, and then decreases by 1/16, 1/32..., and finally the value of the Y axis is infinitely small and approaches 0. It is also very simple to avoid floating-point operations. We use 256 to represent 1 degree (that is, 8 bits). In this way, it can be accurate to 1/256=0.00390625 degrees, which is accurate enough for most cases. 256 represents 1 degree, so 45 degrees is 45*256 = 115200. Other degrees are similar. Only integer operations are performed, avoiding floating-point operations.
The second part has a relatively large amount of calculation and is also of concern to programmers. Generally, trigonometric function calculation and coordinate transformation are provided by the SDK provided by the lidar manufacturer.
When it comes to point cloud registration, the PCL library is indispensable. The PCL library is derived from ROS, the robot operating system. The robot system often uses laser radar 3D images. In order to reduce repeated development and connect various platforms, ROS launched the PCL library. PCL (Point Cloud Library) is a large cross-platform open source C++ programming library built on the basis of previous point cloud related research. It implements a large number of general point cloud-related algorithms and efficient data structures, involving point cloud acquisition, filtering, segmentation, registration, retrieval, feature extraction, recognition, tracking, surface reconstruction, visualization, etc. It supports multiple operating system platforms and can run on Windows, Linux, Android, MacOSX, and some embedded real-time systems. If OpenCV is the crystallization of 2D information acquisition and processing, then PCL has the same status in 3D information acquisition and processing. PCL is a BSD license method and can be used for commercial and academic applications free of charge. The main people behind it are the University of Munich (TUM - Technische Universität München) and Stanford University. In the industrial field, in addition to all lidar manufacturers supporting the PCL library, Toyota and Honda are also sponsors of the PCL library.
The PCL library was established in 2013. At that time, the CPU was the main computing resource, so the PCL library was optimized for the CPU. The underlying data structures in PCL make extensive use of SSE (which can be seen as SIMID) optimization. Most of its mathematical operations are implemented based on Eigen (an open source C++ linear algebra library). In addition, PCL also provides support for OpenMP and Intel Thread Building Blocks (TBB) libraries to achieve multi-core parallelization. The backbone of the fast nearest neighbor search algorithm is provided by FLANN (a library that performs fast approximate nearest neighbor search). All modules and algorithms in PCL pass data using Boost shared pointers, thus avoiding re-copying data that already exists in the system.
The two most commonly used methods for point cloud registration are the iterative closest point algorithm ICP (Iterative Closest Point), the KD tree can be seen as a high-dimensional version of ICP, and the normal distribution transform NDT (Normal Distribution Transform). These two algorithms are naturally in the PCL library. Intel launched the Open3D open source library similar to the PCL library in 2020. Needless to say, there are also optimizations for the CPU.
Previous article:Zadar Labs Launches Software-Defined 4D Millimeter-Wave Imaging Radar with 0.4° Resolution
Next article:How good is the “first stock in automotive chips”?
Recommended ReadingLatest update time:2024-11-16 14:39
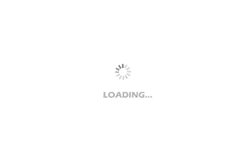
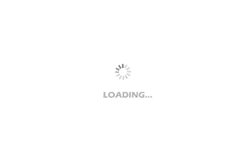
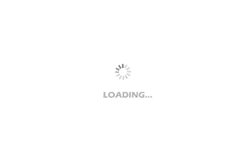
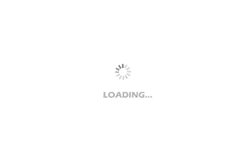
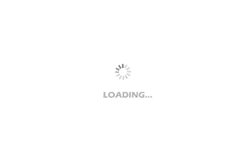
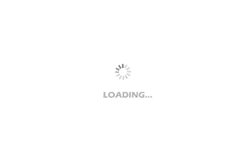
- Popular Resources
- Popular amplifiers
-
Virtualization Technology Practice Guide - High-efficiency and low-cost solutions for small and medium-sized enterprises (Wang Chunhai)
-
Semantic Segmentation for Autonomous Driving: Model Evaluation, Dataset Generation, Viewpoint Comparison, and Real-time Performance
-
Design and application of autonomous driving system (Yu Guizhen, Zhou Bin, Wang Yang, Zhou Yiwei)
-
ASPEN: High-throughput LoRA fine-tuning of large language models using a single GPU
- Car key in the left hand, liveness detection radar in the right hand, UWB is imperative for cars!
- After a decade of rapid development, domestic CIS has entered the market
- Aegis Dagger Battery + Thor EM-i Super Hybrid, Geely New Energy has thrown out two "king bombs"
- A brief discussion on functional safety - fault, error, and failure
- In the smart car 2.0 cycle, these core industry chains are facing major opportunities!
- The United States and Japan are developing new batteries. CATL faces challenges? How should China's new energy battery industry respond?
- Murata launches high-precision 6-axis inertial sensor for automobiles
- Ford patents pre-charge alarm to help save costs and respond to emergencies
- New real-time microcontroller system from Texas Instruments enables smarter processing in automotive and industrial applications
- Innolux's intelligent steer-by-wire solution makes cars smarter and safer
- 8051 MCU - Parity Check
- How to efficiently balance the sensitivity of tactile sensing interfaces
- What should I do if the servo motor shakes? What causes the servo motor to shake quickly?
- 【Brushless Motor】Analysis of three-phase BLDC motor and sharing of two popular development boards
- Midea Industrial Technology's subsidiaries Clou Electronics and Hekang New Energy jointly appeared at the Munich Battery Energy Storage Exhibition and Solar Energy Exhibition
- Guoxin Sichen | Application of ferroelectric memory PB85RS2MC in power battery management, with a capacity of 2M
- Analysis of common faults of frequency converter
- In a head-on competition with Qualcomm, what kind of cockpit products has Intel come up with?
- Dalian Rongke's all-vanadium liquid flow battery energy storage equipment industrialization project has entered the sprint stage before production
- Allegro MicroSystems Introduces Advanced Magnetic and Inductive Position Sensing Solutions at Electronica 2024
- Car key in the left hand, liveness detection radar in the right hand, UWB is imperative for cars!
- After a decade of rapid development, domestic CIS has entered the market
- Aegis Dagger Battery + Thor EM-i Super Hybrid, Geely New Energy has thrown out two "king bombs"
- A brief discussion on functional safety - fault, error, and failure
- In the smart car 2.0 cycle, these core industry chains are facing major opportunities!
- The United States and Japan are developing new batteries. CATL faces challenges? How should China's new energy battery industry respond?
- Murata launches high-precision 6-axis inertial sensor for automobiles
- Ford patents pre-charge alarm to help save costs and respond to emergencies
- New real-time microcontroller system from Texas Instruments enables smarter processing in automotive and industrial applications
- CH340 circuit to prevent current backflow
- Bluetooth module responds incorrectly
- 【RT-Thread Reading Notes】Reflections on RT-Thread Learning Chapter 6
- 【ST NUCLEO-H743ZI Review】——by supermiao123
- How to Reduce the Temperature in Your Car's Fuse Box
- STM32 supports VCP+MSC+HID mode
- How does AD20 perform multi-person collaboration? (Multiple picture warning
- 13. [Learning LPC1768 library functions] ADC experiment
- Application of RPA in the customer service industry
- Efficiency Programming of Single Chip Microcomputer Active Buzzer Driver