Reliability is a problem that exists in every link of product design, manufacturing and use. Simply put, reliability is the degree to which a product is not prone to failure. As we all know, products are usually qualified when they leave the factory, but as time goes by, the functions and performance of the products will gradually change, eventually leading to failures. Although this trend cannot be changed, designing and manufacturing products that do not fail within a specified time is a topic of concern to both companies and consumers. From the frequent communication failures of US fighter planes in the early days of World War II to the quality problems of a certain brand of laptop computers that were repeatedly exposed during the 3.15 period this year, in essence, they are all caused by the failure of product reliability. The reasonable application of reliability analysis can help technical personnel in R&D, engineering, quality and other departments to improve the stability of product quality, reduce product life cycle costs and after-sales service costs, and improve customer satisfaction and loyalty.
What is puzzling is that many companies have realized the importance of reliability analysis, but they still deliberately avoid reliability analysis. Why is this? There are many reasons. One of the main reasons is that most companies feel that conventional statistical quality management is complicated enough, and reliability research requires a lot of more advanced statistical knowledge. This is daunting for people who have not received formal statistical method training. This objectively greatly limits the promotion of reliability methods in companies.
The author has tried to use different software for reliability analysis. SAS's high-end Six Sigma software JMP is one of them. Its interactive visual analysis feature is also very good in terms of reliability. Let's take a typical example to see how to use JMP for reliability analysis.
Example: In order to conduct reliability analysis on an electronic product, a company collected a batch of service life data of the product (as shown in Figure 1, when "Censored" = 0, it means that "Time" is the exact failure time, and when "Censored" = 1, it means that the exact failure time is unknown, but it is definitely greater than the value shown by "Time"). With this set of representative data, let's study the failure characteristics of the product. When the failure probability is 90%, what is the reliable life of the product?
Figure 1 Reliability test original data table (part)
According to the theory of reliability method, to solve these two problems, we need to solve a basic problem first: what distribution does this set of life data obey? To be honest, this is not an easy problem to solve. We have to try, compare and verify one distribution after another, such as Weibull distribution, lognormal distribution, exponential distribution, etc. There are at least a dozen kinds. However, general quality engineers will feel dizzy when they hear these professional statistical terms. In addition, since life data also contains the "missing" feature, it is more complicated to judge. Usually, a series of lengthy statistical analysis reports and statistical indicators are needed to judge.
When I was using JMP software for analysis, I found a command in JMP called "Fit All Distributions". It can fit all conventional reliability distributions one by one in a few seconds, and then automatically select the best distribution fit. For example, in the figure below, the "lognormal" distribution is the best distribution found by JMP after quickly comparing all life distributions. If you don't understand the principles of statistics very well and just want to see what the distribution looks like intuitively, just look at the red curve and the pink confidence interval band around it; if you understand the principles of statistics better and want to have a deeper understanding of the statistical basis for judgment, you can also look at the relevant indicators in the "Model Fitting" table below. In short, you can take what you need to complete the most basic distribution model identification task. [page]
Figure 2 Visualization of reliability distribution model comparison
In addition, while obtaining the best fitting distribution, various reliability characteristics related to the product quality (such as reliable life, failure probability, failure probability density, failure rate, etc.) can also be expressed graphically. For example, in the figure below, the "Distribution Profiler" and "Quantile Profiler" both show the relationship between failure probability and product life (the main difference between the two is that the variables represented by the X-axis and the Y-axis are exactly opposite), the "Hazard Rate Profiler" shows the law of change of hazard rate (commonly known as Hazard Rate) with the change of product life (this can be used to realize the most famous "Bathtub Curve" in reliability theory), and the "Density Profiler" shows the law of change of failure probability density with the change of product life.
Figure 3 Profilers of the main characteristic variables in reliability analysis
These graphs can be used to vividly explain the first question, "What are the failure characteristics of this product?" Now let's answer the second question, "When the failure probability is 90%, what is the reliable life of this product?" Enter "0.9" on the X-axis of the "Quantile Profiler" and you will get the red value "412.0117" and the blue value "[278.675,609.146]" on the Y-axis, indicating that the reliable life at this time should be 412.0117, and its confidence interval should be [278.675,609.146].
There are many other contents about reliability analysis, such as accelerated life analysis, regression analysis of life data, and maintainability analysis, etc. These have corresponding graphical intelligent analysis platforms in JMP software. One direct benefit is that it allows engineers with non-statistical backgrounds to quickly overcome the barriers of statistics and directly analyze and study technical problems, thereby improving product reliability and reducing customer complaints and after-sales service costs.
Previous article:Automatic detection and fault diagnosis system of air conditioning equipment based on PXI bus
Next article:Design of remote monitoring system for tobacco fields based on virtual instrument technology
Recommended ReadingLatest update time:2024-11-16 19:47
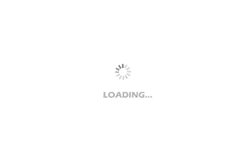
- Keysight Technologies Helps Samsung Electronics Successfully Validate FiRa® 2.0 Safe Distance Measurement Test Case
- From probes to power supplies, Tektronix is leading the way in comprehensive innovation in power electronics testing
- Seizing the Opportunities in the Chinese Application Market: NI's Challenges and Answers
- Tektronix Launches Breakthrough Power Measurement Tools to Accelerate Innovation as Global Electrification Accelerates
- Not all oscilloscopes are created equal: Why ADCs and low noise floor matter
- Enable TekHSI high-speed interface function to accelerate the remote transmission of waveform data
- How to measure the quality of soft start thyristor
- How to use a multimeter to judge whether a soft starter is good or bad
- What are the advantages and disadvantages of non-contact temperature sensors?
- Innolux's intelligent steer-by-wire solution makes cars smarter and safer
- 8051 MCU - Parity Check
- How to efficiently balance the sensitivity of tactile sensing interfaces
- What should I do if the servo motor shakes? What causes the servo motor to shake quickly?
- 【Brushless Motor】Analysis of three-phase BLDC motor and sharing of two popular development boards
- Midea Industrial Technology's subsidiaries Clou Electronics and Hekang New Energy jointly appeared at the Munich Battery Energy Storage Exhibition and Solar Energy Exhibition
- Guoxin Sichen | Application of ferroelectric memory PB85RS2MC in power battery management, with a capacity of 2M
- Analysis of common faults of frequency converter
- In a head-on competition with Qualcomm, what kind of cockpit products has Intel come up with?
- Dalian Rongke's all-vanadium liquid flow battery energy storage equipment industrialization project has entered the sprint stage before production
- Allegro MicroSystems Introduces Advanced Magnetic and Inductive Position Sensing Solutions at Electronica 2024
- Car key in the left hand, liveness detection radar in the right hand, UWB is imperative for cars!
- After a decade of rapid development, domestic CIS has entered the market
- Aegis Dagger Battery + Thor EM-i Super Hybrid, Geely New Energy has thrown out two "king bombs"
- A brief discussion on functional safety - fault, error, and failure
- In the smart car 2.0 cycle, these core industry chains are facing major opportunities!
- The United States and Japan are developing new batteries. CATL faces challenges? How should China's new energy battery industry respond?
- Murata launches high-precision 6-axis inertial sensor for automobiles
- Ford patents pre-charge alarm to help save costs and respond to emergencies
- New real-time microcontroller system from Texas Instruments enables smarter processing in automotive and industrial applications
- LTE Cat 1 STM32 4G EC200S
- Single-ended to balanced conversion, the actual situation is so different from the simulation, why?
- [NXP Rapid IoT Review] + Preliminary Study on Power Consumption of Some Devices
- Embedded Linux Learning Route Planning
- Today is the summer solstice. I heard that those who went out at noon had no shadow.
- Panta STM32H750 Part 6 (Using timer synchronization to generate a specified number of PWM pulses)
- The TI Cup is coming soon. Which editions have you participated in?
- The topology of the switching power supply in the electric competition must be solved according to the problem-solving ideas
- I encountered a problem. Before the program was burned, the voltage obtained by AIO4 was half of the battery voltage. After the program was burned...
- Qorvo breaks power barrier with 10W Ka-band GaN amplifier