Previous article:Honeywell and Sinovation Medical jointly develop a new pattern of smart medical development
Next article:Strategy Analytics: Cellular IoT device shipments to grow, reaching 350 million by 2025
Recommended ReadingLatest update time:2024-11-17 01:53
Suzhou has introduced a special policy to support artificial intelligence for the first time
On April 19, Suzhou held a conference to promote the construction of "one zone and two centers" (National Biotechnology Innovation Center, National Third Generation Semiconductor Technology Innovation Center, and National New Generation Artificial Intelligence Innovation Development Pilot Zone). At the meeting, "Sever
[Mobile phone portable]
AI empowers Gululu Q smart voice water bottle, focusing on children's smart IoT field
China's high-end children's water cup Gululu series of smart interactive water cups will launch its fifth product Gululu Q smart voice water cup on October 21 at Tmall Xinuo flagship store, and will be officially launched at Tmall Gululu mother and baby flagship store in November. The new product Gululu Q has a built-
[Embedded]
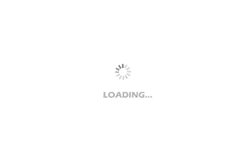
It is reported that Nvidia is pursuing orders for AI chips again, and TSMC is urgently purchasing additional CoWoS packaging equipment.
According to news on September 25, as the demand for NVIDIA AI chips is booming, the foundry TSMC has also been increasing its production capacity. According to the Taiwanese media "Economic Daily", TSMC's CoWoS (IT Home Note: Chip-on-Wafer-on-Substrate) advanced packaging production capacity is full and it is activel
[Semiconductor design/manufacturing]
Explainable AI image recognition startup receives funding from the US Air Force, technology can be used in self-driving cars, etc.
According to foreign media reports, software startup Z Advanced Computing (ZAC) has received funding from the US Air Force, which will use its 3D image detail recognition technology (which is based on explainable AI technology) for aerial image/target recognition by unmanned aerial vehicles (UAVs). (Image source: Z
[Automotive Electronics]
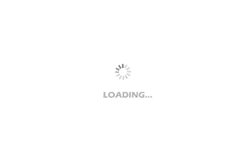
CPC debuts at ODCC and joins hands with NVIDIA to provide liquid cooling technology for GPUs to accelerate AI computing
Arden Hills, Minnesota – September 2, 2024 –
CPC (Colder Products Company) will attend the annual Open Data Center Conference at the Beijing International Convention Center on September 3-4, 2024. With the rapid development of technologies such as artificial intelligence (AI), big data, and the Internet of Thing
[Industrial Control]
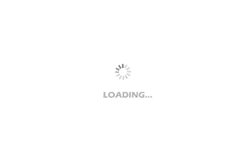
NVIDIA GPU is so weak! The world's first AI chip upgrades to 4 trillion transistors and 900,000 cores
According to news on March 14, Cerebras Systems released their third-generation wafer-level AI acceleration chip "WSE-3" (Wafer Scale Engine 3). The specifications and parameters are even crazier, and the performance remains the same without changing the power consumption and price. Doubled. The first generation WSE-1
[Semiconductor design/manufacturing]
Helm.ai launches VidGen-2, a generative AI platform for enhancing self-driving videos
Generative AI will soon help give self-driving cars perception capabilities. Artificial intelligence (AI) software provider Helm.ai has launched VidGen-2, the next generation of its generative AI model for generating realistic driving video sequences.
Image source: Helm.ai
VidGen-2 has tw
[Automotive Electronics]
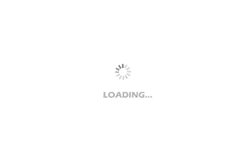
Huawei's Chen Yaxin: 5G, cloud and AI are the three elements of new infrastructure
The main forum of the 9th Global Internet of Things Summit was grandly held on November 16. The summit took "5G empowering enterprise digital transformation" as its entry point, focusing on the digital transformation of enterprises and the innovative applications of 5G+AIOT. Chen Yaxin, deputy director of Huawei's Ch
[Mobile phone portable]
- Popular Resources
- Popular amplifiers
Recommended Content
Latest Internet of Things Articles
- e-Network Community and NXP launch Smart Space Building Automation Challenge
- The Internet of Things helps electric vehicle charging facilities move into the future
- Nordic Semiconductor Launches nRF54L15, nRF54L10 and nRF54L05 Next Generation Wireless SoCs
- Face detection based on camera capture video in OPENCV - Mir NXP i.MX93 development board
- The UK tests drones equipped with nervous systems: no need to frequently land for inspection
- The power of ultra-wideband: reshaping the automotive, mobile and industrial IoT experience
- STMicroelectronics launches highly adaptable and easy-to-connect dual-radio IoT module for metering and asset tracking applications
- This year, the number of IoT connections in my country is expected to exceed 3 billion
- Infineon Technologies SECORA™ Pay Bio Enhances Convenience and Trust in Contactless Biometric Payments
IoT Encyclopedia
MoreSelected Circuit Diagrams
MorePopular Articles
- Innolux's intelligent steer-by-wire solution makes cars smarter and safer
- 8051 MCU - Parity Check
- How to efficiently balance the sensitivity of tactile sensing interfaces
- What should I do if the servo motor shakes? What causes the servo motor to shake quickly?
- 【Brushless Motor】Analysis of three-phase BLDC motor and sharing of two popular development boards
- Midea Industrial Technology's subsidiaries Clou Electronics and Hekang New Energy jointly appeared at the Munich Battery Energy Storage Exhibition and Solar Energy Exhibition
- Guoxin Sichen | Application of ferroelectric memory PB85RS2MC in power battery management, with a capacity of 2M
- Analysis of common faults of frequency converter
- In a head-on competition with Qualcomm, what kind of cockpit products has Intel come up with?
- Dalian Rongke's all-vanadium liquid flow battery energy storage equipment industrialization project has entered the sprint stage before production
MoreDaily News
- Allegro MicroSystems Introduces Advanced Magnetic and Inductive Position Sensing Solutions at Electronica 2024
- Car key in the left hand, liveness detection radar in the right hand, UWB is imperative for cars!
- After a decade of rapid development, domestic CIS has entered the market
- Aegis Dagger Battery + Thor EM-i Super Hybrid, Geely New Energy has thrown out two "king bombs"
- A brief discussion on functional safety - fault, error, and failure
- In the smart car 2.0 cycle, these core industry chains are facing major opportunities!
- Rambus Launches Industry's First HBM 4 Controller IP: What Are the Technical Details Behind It?
- The United States and Japan are developing new batteries. CATL faces challenges? How should China's new energy battery industry respond?
- Murata launches high-precision 6-axis inertial sensor for automobiles
- Ford patents pre-charge alarm to help save costs and respond to emergencies
Guess you like
- Bluetooth Low Energy (BLE) peripheral mode (peripheral) - using BLE as a server
- The problem of the total distortion increasing after the signal passes through the op amp filter circuit
- FPGA implementation of the design of RU algorithm encoder (LDPC encoder)
- [NXP Rapid IoT Review] + Laptop Bluetooth Interaction with Rapid IOT
- GPIO Operation of TMS320C6748
- The data of the differential resistance sensor is fluctuating greatly, with about 11 bits fluctuating.
- TI - MCU - MSP430 User Guide 3 -> SYS System Control
- Is there anything you want to DIY now? What do you lack the most?
- 【Scene Reproduction Project Based on AI Camera】Project Submission
- Weekly highlights: 2018.10.22-10.28