Since its launch, Baidu Apollo Open Platform has always maintained innovation and high-frequency self-iteration, from focusing on the construction of basic capabilities at the beginning, to focusing on the development of scenario capabilities, and then upgrading to the pursuit of excellence in platform engineering usability. On December 28, 2022, the Apollo Open Platform officially launched a new upgraded version of the Apollo autonomous driving open platform, Apollo 8.0, for all developers, further consolidating the platform's usability and making it easier for developers to operate.
Apollo 8.0 has been upgraded in the following aspects:
1. Software Package Management
In the latest version 8.0, we have reorganized the dependencies between modules and introduced the concept of software packages to manage and release each module. We have optimized the problems of large download volume, inability to use on demand, and long compilation time during download and installation. On this basis, we have updated and released extension solutions based on application scenarios to allow more developers to use Apollo better and faster. (*About [Package Management]: Package management standardizes the compilation output of Apollo according to the "modular" granularity. On the one hand, it supports the direct use of components by using the output package; on the other hand, it standardizes the dependency relationship and granularity of components, thereby reducing the difficulty of using/reusing components and improving the R&D efficiency of autonomous driving systems. For detailed specifications, please refer to the document Introduce to Package of Apollo.)
1. Clearer module structure, lower learning threshold
Compared with the complicated dependencies between modules in Apollo in the past, developers who want to understand and learn Apollo often fall into the difficulty of code reading. In Apollo 8.0, we reorganized the various modules of Apollo, removed unreasonable dependencies, integrated common modules, and made the overall structure of Apollo clearer and simpler. In addition, we put the clear declaration of the dependencies of each module in the package description file (cyberfile.xml) to facilitate developers to view it uniformly. At the same time, we also provide a quick view tool to facilitate developers to understand and learn related codes, and overall lower the learning threshold for developers.
2. Faster deployment method to improve compilation efficiency
In the past, developers needed to download the source code and then fully compile Apollo to complete the entire deployment. This process was time-consuming and often took days, with a high failure rate, which became the first barrier for developers to use Apollo. In order to improve the compilation efficiency of developers, we introduced the concept of package management in Apollo 8.0. We pre-compiled and released each Apollo module on a modular basis, and provided more fine-grained packages for developers to obtain and use on demand. Whether developers want to quickly experience or use Apollo, they can quickly install and deploy it directly through the tools we provide. The entire process can be completed in less than 30 minutes, greatly reducing the time for downloading and compiling.
3. More convenient expansion solutions, convenient for secondary expansion, verification and practice
Take the example of extending and modifying the Planning module (the code of the Demo project can be found at https://github.com/ApolloAuto/application-demo ). You only need to create the following sample declaration file (Planning is installed as source code), and then execute the compilation command to install the Planning source code into the workspace. At the same time, the necessary modules related to Planning debugging will also be installed as binary packages. Then we can directly modify the Planning source code, compile, and debug in the workspace to build a Planning module suitable for the required scenario. To build a complete end-to-end autonomous driving software system, you only need to add corresponding modules on the basis of the above, such as Perception, Prediction, Control, etc.
For more examples of scenarios, see the Apollo 8.0 Quick Start document.
2. Perception Framework
To help developers improve the development efficiency of perception modules, in Apollo 8.0, we provide a complete end-to-end autonomous driving perception development process. We have made improvements in the four main aspects of data, model, framework, and verification. At the same time, we have iteratively optimized task processes and tools to help developers quickly develop, deploy, and verify autonomous driving perception and improve perception development efficiency.
1. Clear task pipeline and various algorithm plug-ins
In the 8.0 perception framework, developers can create corresponding pipelines according to different perception task types and define pipeline tasks through configuration files. Compared with before, the operation process of each task is clearer and convenient for expansion. In addition, developers can also choose different algorithm plug-ins according to their needs. For example, the Apollo perception module provides 4 types of detectors. Developers can choose different detectors according to the configuration files to verify the detection effect. Through the algorithm plug-in, algorithm engineers can focus more on the algorithm itself without paying too much attention to the implementation of the framework.
2. Brand-new model training, easy-to-use deep learning model
In Apollo 8.0, Apollo and Paddle3D provide an end-to-end autonomous driving model development solution, covering the entire algorithm development process from autonomous driving data sets to model training, model evaluation, and model export. For the 3D target detection and segmentation tasks that developers are more concerned about in autonomous driving, Apollo provides the latest SOTA algorithm model implementation, including monocular camera detection, lidar point cloud target detection, and multimodal target detection models. Developers can use them out of the box without having to reproduce the model themselves. At the same time, we also provide model benchmarks, including speed, accuracy and other indicators as well as pre-trained models. Developers can track the latest 3D target detection and segmentation model implementations in real time to maintain the advancement of autonomous driving perception algorithms.
Three deep learning models have been introduced in the Apollo 8.0 perception model:
PETR: A representative model in the field of visual BEV in the current autonomous driving direction. The model innovatively integrates 3D coordinate information with image features, uses the Transfomer structure to perform end-to-end 3D target detection, and realizes 360° obstacle perception based on vision. The overall architecture of the model is simple in design, and a good tradeoff is achieved between speed and accuracy. The accuracy on nuScenes reaches 43.52 NDS and 38.35mAP.
CenterPoint: A cutting-edge model in the field of point cloud detection. This model is an Anchor-Free 3D object detector that regresses the size, direction, and speed of an object based on key point detection. Compared with Anchor-Based 3D object detectors, CenterPoint does not require manual setting of the anchor size, and its accuracy is higher when facing scenes with objects of varying sizes. The structural design of the model is simple and efficient, with an accuracy of 61.30 NDS and 50.97 mAP on nuScenes.
CaDDN: A cutting-edge model based on monocular 3D detection. Aiming at the pathological problem of predicting 3D objects from a single image, CaDDN innovatively proposes a solution that uses the predicted classification depth distribution of each pixel to project rich contextual feature information into the appropriate depth interval in the 3D space. It also uses computationally efficient bird's-eye view projection and a single-stage detector to generate the final output bounding box, bringing the model indicators of monocular 3D to a new level and achieving high accuracy indicators in KITTI data (Car category 3D AP 21.45 14.36 12.57).
3. Efficient model management and convenient model verification
In order to deploy trained models to the Apollo system more conveniently and quickly, we introduced model Meta and model management in Apollo 8.0. Model Meta contains basic information about the model, such as name, task type, sensor type, framework, and data set required for training. It also contains the model's standard input, input, pre- and post-processing, and model file storage path. At the same time, Apollo also provides a model management tool, through which developers can download and install models in the model repository, and display the models installed in the system and detailed information about the models. By standardizing the model and using the model management tool, developers can easily install and deploy trained models, and manage these models to improve model deployment efficiency.
In addition, in the perception model verification, we provide a data package (record file) based on the data set, which allows developers to directly verify the detection effect of the model online based on the data set data, ensure that the training and deployment are based on the same set of baselines, and quickly test the model performance. In addition to providing test data packages, Apollo 8.0 also provides a visualization tool chain that displays the raw data of the sensor and the target detection results through a visual graphical interface, making it easier for developers to view the model detection effect and debug the perception model.
3. Toolchain
To further meet the needs of the autonomous driving development process and improve the efficiency of developers, in Apollo 8.0, we provide a more comprehensive and easier-to-use tool chain based on the pain points and demands of developers, accelerate the development process of developers, and help them get started with Apollo better and faster.
Previous article:New energy vehicle prototype benchmarking technology development and design
Next article:Comparison of thermal management technology routes between Tesla and Huawei
Recommended ReadingLatest update time:2024-11-16 17:43
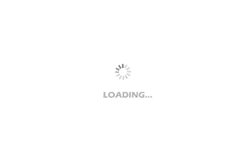
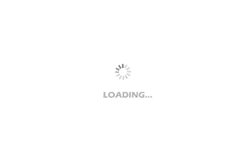
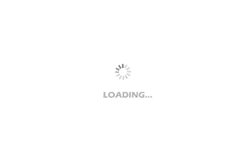
- Popular Resources
- Popular amplifiers
-
Network Operating System (Edited by Li Zhixi)
-
Microgrid Stability Analysis and Control Microgrid Modeling Stability Analysis and Control to Improve Power Distribution and Power Flow Control (
-
MATLAB and FPGA implementation of wireless communication
-
Introduction to Internet of Things Engineering 2nd Edition (Gongyi Wu)
- Huawei's Strategic Department Director Gai Gang: The cumulative installed base of open source Euler operating system exceeds 10 million sets
- Analysis of the application of several common contact parts in high-voltage connectors of new energy vehicles
- Wiring harness durability test and contact voltage drop test method
- Sn-doped CuO nanostructure-based ethanol gas sensor for real-time drunk driving detection in vehicles
- Design considerations for automotive battery wiring harness
- Do you know all the various motors commonly used in automotive electronics?
- What are the functions of the Internet of Vehicles? What are the uses and benefits of the Internet of Vehicles?
- Power Inverter - A critical safety system for electric vehicles
- Analysis of the information security mechanism of AUTOSAR, the automotive embedded software framework
Professor at Beihang University, dedicated to promoting microcontrollers and embedded systems for over 20 years.
- Innolux's intelligent steer-by-wire solution makes cars smarter and safer
- 8051 MCU - Parity Check
- How to efficiently balance the sensitivity of tactile sensing interfaces
- What should I do if the servo motor shakes? What causes the servo motor to shake quickly?
- 【Brushless Motor】Analysis of three-phase BLDC motor and sharing of two popular development boards
- Midea Industrial Technology's subsidiaries Clou Electronics and Hekang New Energy jointly appeared at the Munich Battery Energy Storage Exhibition and Solar Energy Exhibition
- Guoxin Sichen | Application of ferroelectric memory PB85RS2MC in power battery management, with a capacity of 2M
- Analysis of common faults of frequency converter
- In a head-on competition with Qualcomm, what kind of cockpit products has Intel come up with?
- Dalian Rongke's all-vanadium liquid flow battery energy storage equipment industrialization project has entered the sprint stage before production
- Allegro MicroSystems Introduces Advanced Magnetic and Inductive Position Sensing Solutions at Electronica 2024
- Car key in the left hand, liveness detection radar in the right hand, UWB is imperative for cars!
- After a decade of rapid development, domestic CIS has entered the market
- Aegis Dagger Battery + Thor EM-i Super Hybrid, Geely New Energy has thrown out two "king bombs"
- A brief discussion on functional safety - fault, error, and failure
- In the smart car 2.0 cycle, these core industry chains are facing major opportunities!
- The United States and Japan are developing new batteries. CATL faces challenges? How should China's new energy battery industry respond?
- Murata launches high-precision 6-axis inertial sensor for automobiles
- Ford patents pre-charge alarm to help save costs and respond to emergencies
- New real-time microcontroller system from Texas Instruments enables smarter processing in automotive and industrial applications
- Altium Designer 19.1.6, Reports BOM does not generate the BOM table, and AD19 will be stuck. What is the reason?
- Do you really know the impact of AC coupling capacitors?
- [Digi-Key Innovation Design Competition] Material Unboxing + ESP32-S3-BOX
- EEWORLD University - Code reuse demonstration using TI SimpleLink? MCU platform
- STM32 RTC registers
- Inductance calculation formula
- [NXP Rapid IoT Review] + Recommendation for NXP Rapid IoT to connect the differential ADC input port of KW64 to the expansion port
- [Allwinner V853 heterogeneous AI intelligent vision development board review] "mkimage command not found" error solution...
- Live broadcast with prizes | Sign up for TI's June Embedded Processing Live Theme Week to get a glimpse of new products in industrial scenarios!
- [MSP430] Practical ADC use, internal temperature measurement use