According to foreign media reports, Tesla is unique in the field of autonomous driving. The company is committed to developing computer vision and artificial neural network technologies to solve the challenges associated with autonomous vehicles.
While Toyota, Google, Uber, Ford, and GM are all committed to adopting lidar technology, Tesla has always loudly declared that lidar will never be the solution.
Tesla CEO Elon Musk famously said: “LiDAR is an exercise in futility and any company relying on it is doomed to fail.”
So, what, exactly, are LiDAR’s flaws and computer vision’s biggest advantages?
Achilles' heel - the fatal weakness of lidar
LiDAR is extremely efficient at measuring distances down to the millimeter level, but it’s not as effective when it comes to moving objects.
According to Andrej Karpathy, senior director of Tesla's artificial intelligence department, lidar cannot distinguish between plastic bags and speed bumps on the road, which poses a safety hazard: when driving towards a speed bump, the vehicle needs to slow down before it can easily pass through the plastic speed bump (avoid bumps).
In addition, until now, the product cost of lidar technology is still extremely expensive, which creates a problem: if a car model is equipped with multiple lidars at the same time, the cost-effectiveness of the car model will be low.
Despite its many flaws, the biggest challenge associated with lidar comes from 3D high-definition maps, which are an indispensable and important technology. Without 3D high-definition maps, autonomous driving is out of the question.
The 3D street view (modeling) created by this type of map is also a technology required for autonomous driving and its safety, and can only be used in conjunction with Google's continuously updated 3D HD maps.
However, mapping even one centimeter of street view requires a lot of resources and is expensive from a monetary perspective, which means that vehicles equipped with lidar can only travel in limited areas where maps have been drawn, which greatly limits the coverage of autonomous vehicles.
Tesla's unconventional technology path
Tesla is the world's largest electric car manufacturer, and its technology path is very different from that of its competitors. The company hopes to give vehicles "vision" capabilities so that they can complete driving operations like humans.
Unlike its competitors, Tesla has not adopted lidar. Instead, the company has focused on developing an Advanced Driver Assistance System (ADAS) or semi-auto pilot system that works in conjunction with a camera and radar system. (Recently, Tesla vehicles have been upgraded to use a camera-only system.)
The integration of cameras and radar provides the algorithms with the input data needed to create a map of the vehicle's surroundings, which uses computer vision and AI-based algorithms to make driving decisions, almost like reverse engineering human vision.
Tesla uses a matrix kit of 8 cameras + radar to achieve functions such as autonomous driving, automatic parking, lane centering control, adaptive cruise control and sidewalks.
Tesla's onboard software is based on deep learning algorithms, which attempt to develop advanced neural networks in functions that play a similar role to the human visual system, which requires collecting pure video input from the vehicle's surrounding environment.
The neural networks then analyze the video input of roads, road signs, pedestrians, speed bumps, obstacles and other vehicles.
The main controversy facing pure computer vision is that without the assistance of lidar and radar equipment, we cannot be sure whether the neural network can accurately measure distance and complete depth prediction.
To solve this problem, Tesla has been training its neural network, inputting video data sets collected from Tesla vehicles around the world, and completing the labeling of the above videos with the assistance of automatic algorithms and manual supervision.
Andrei elaborated on the above at the Computer Vision and Pattern Recognition Conference 2021. He revealed to the audience that during the development of the dataset used to train the algorithm, his team identified more than 200 triggers, suggesting that object detection still needs to be fine-tuned.
Such triggers will cause inconsistencies between detection results. For this reason, Tesla had to spend another four months to fix each trigger. Tesla also used supercomputers to train deep learning modeling and achieve optimization adjustments.
Tesla's primary goal is to develop an autonomous computer-generated general-purpose vision system similar to the human visual system and a set of algorithms that can fully utilize the performance of the Autopilot vehicle system.
Today, Tesla is only looking at this as a supervised learning problem. The company has improved the performance of convolutional neural networks, hoping that future vehicles will be able to control and operate the vehicle based on computer vision technology.
On the other hand, the progress of lidar technology is not exciting. In addition to the falling price, lidar has not made much progress in mapping, not to mention other flaws.
So let's wait and see with excitement - can Tesla's technology path perform well in the future? And then we can see whether LiDAR can eliminate its shortcomings through self-technological innovation?
Previous article:AI DAY Interpretation - Tesla Autopilot's Visual Architecture
Next article:BlackBerry and Nobo Technology jointly develop intelligent cockpit domain controller
Recommended ReadingLatest update time:2024-11-22 05:36
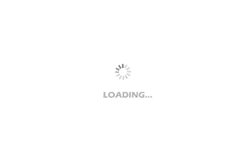
Recommended posts
- Phase-Locked Loop (PLL) Basics
- Phase-LockedLoop(PLL)Basics Aphase-lockedloopisafeedbacksysteminwhichavoltage-controlledoscillator(VCO)andaphasecomparatorareconnectedsothattheoscillatorcanmaintainaconstantphaseanglerelativetoareferencesignal.
-
qwqwqw2088
Analogue and Mixed Signal
- GaN finishing station 1
- GaNGoesMobile GaNandapplicationunderstanding GaNtechnologydetailedexplanationishere DifferencebetweenGaNandSiC GaNReferenceDesigns UnderstandingGaNThermalAnalysis UnderstandingThermalAnalysisofGaN GaNRFCircui
-
btty038
RF/Wirelessly
- [GD32L233C-START Review] + Unboxing and Building a Development Environment
- Preface Firstofall,IwouldliketothankeeworldandGigaDeviceforprovidingthistrialopportunity.IhaveusedGD32F103CBT6inpreviousprojects,andthedevelopmentwasquitesmooth,butpowerconsumptionhasalwaysbeenarela
-
laocuo1142
GD32 MCU
- MicroPython drives Weixue 2.13-inch ink screen (electronic paper)
- 原帖:https://forum.micropython.org/viewtopic.php?t=6319&p=35965 https://www.waveshare.com/w/thumb.php?f=2.13inch-e-paper-hat-b-5_1.jpg&width=300""" Examplefor2.13inchblack&white&redWaveshare2.13BE-inkscreen RunonESP32Wavesha
-
dcexpert
MicroPython Open Source section
- 【MSPM0L1306 LaunchPad】Add FinSH component to rt-thread_nano system
- AddFinSHcomponentbasedonthepreviousarticlehttps://bbs.eeworld.com.cn/thread-1259877-1-1.html. 1.Addserialportdriver 1.1.Enabletheserialport 1.2.Modifytheserialportdriver Modifyboard.casfollows Mod
-
TL-LED
MSPM0 MCU
- 【2024 DigiKey Creative Contest】February Catkins Campaign Project-08 Project Demonstration Effect
- Systemcomposition: Pollendetection: PM2.5smogdetection: Becauseofsomeproblemswiththeproject,itwasdelayedforafewdays.Althoughtheeffectisalittledifferentfromtheexpectation,thebasicfunctionshavebe
-
Maker_kun
DigiKey Technology Zone
- Popular Resources
- Popular amplifiers
-
LiDAR point cloud tracking method based on 3D sparse convolutional structure and spatial...
-
GenMM - Geometrically and temporally consistent multimodal data generation from video and LiDAR
-
Comparative Study on 3D Object Detection Frameworks Based on LiDAR Data and Sensor Fusion Technology
-
Dual Radar: A Dual 4D Radar Multimodal Dataset for Autonomous Driving
- Breaking through the intelligent competition, Changan Automobile opens the "God's perspective"
- The world's first fully digital chassis, looking forward to the debut of the U7 PHEV and EV versions
- Design of automotive LIN communication simulator based on Renesas MCU
- When will solid-state batteries become popular?
- Adding solid-state batteries, CATL wants to continue to be the "King of Ning"
- The agency predicts that my country's public electric vehicle charging piles will reach 3.6 million this year, accounting for nearly 70% of the world
- U.S. senators urge NHTSA to issue new vehicle safety rules
- Giants step up investment, accelerating the application of solid-state batteries
- Guangzhou Auto Show: End-to-end competition accelerates, autonomous driving fully impacts luxury...
- Intel promotes AI with multi-dimensional efforts in technology, application, and ecology
- ChinaJoy Qualcomm Snapdragon Theme Pavilion takes you to experience the new changes in digital entertainment in the 5G era
- Infineon's latest generation IGBT technology platform enables precise control of speed and position
- Two test methods for LED lighting life
- Don't Let Lightning Induced Surges Scare You
- Application of brushless motor controller ML4425/4426
- Easy identification of LED power supply quality
- World's first integrated photovoltaic solar system completed in Israel
- Sliding window mean filter for avr microcontroller AD conversion
- What does call mean in the detailed explanation of ABB robot programming instructions?
- Europe's three largest chip giants re-examine their supply chains
- Breaking through the intelligent competition, Changan Automobile opens the "God's perspective"
- The world's first fully digital chassis, looking forward to the debut of the U7 PHEV and EV versions
- Design of automotive LIN communication simulator based on Renesas MCU
- When will solid-state batteries become popular?
- Adding solid-state batteries, CATL wants to continue to be the "King of Ning"
- The agency predicts that my country's public electric vehicle charging piles will reach 3.6 million this year, accounting for nearly 70% of the world
- U.S. senators urge NHTSA to issue new vehicle safety rules
- Giants step up investment, accelerating the application of solid-state batteries
- Guangzhou Auto Show: End-to-end competition accelerates, autonomous driving fully impacts luxury...
- Low-noise electronic design for broadband amplifiers
- Capacitive sensor measurement system module circuit design precision amplifier circuit
- Google Trends VS Baidu Index, both are not willing to be outdone
- EEWORLD University Hall----Remote Bubble Maker
- Last week: Pick up i.MX crossover processors and win NXP AI-IoT series books (32 books left in the prize pool)
- [Repost] A brief analysis of LC resonance circuit and LC oscillation circuit
- Discussing the importance of driverless cars from the fight between a driver and a passenger
- GD32-Colibri-F350Rx Development Board Related Resources
- TBS 1102 Oscilloscope Use
- Introduction to 5G millimeter wave mobile phone antennas - Will metal frames be replaced?