Author: Bernard Ang, Product Marketing Manager, Keysight Technologies
Product testing has always been a key part of the development process to ensure that products meet market expectations in terms of functionality and performance.
However, the traditional product testing process requires a lot of time and resources. On the other hand, modern new product designs are becoming more and more complex, and the requirements for operating conditions are becoming more and more stringent, such as requiring low power consumption, integrating more sensors, and adding more input/output interfaces.
The good news is that artificial intelligence (AI) technology is gradually being applied to modern product testing processes, which is expected to improve the efficiency, accuracy and scalability of testing throughout the product life cycle.
This Keysight article explores the potential of AI to revolutionize product testing, detailing each phase of the product lifecycle, the positive impact of integrating AI into product testing, and various AI technologies applicable to product testing, such as using machine learning (ML) algorithms for predictive maintenance or defect detection, while exploring various real-world application scenarios, challenges, and future development trends.
Product Testing Life Cycle
As we all know, there are several key stages in the product development life cycle. Engineers will perform product testing at each stage to ensure that the entire product development process meets the company's business plans and goals. Over time, most product development processes become more and more complex due to factors such as the emergence of new market demands and fierce competition. Figure 1 shows the various stages of the product development life cycle.
Figure 1: Phases of the product life cycle
Ideation and planning stage
The introduction of the creative stage means starting a new product development project. The main task of this stage is to collect data related to new product ideas from various channels such as customers, scientific research institutions and the field. When planning, it is necessary to conduct relevant research, find potential markets for products, and dig out as much use case information as possible from customers.
All internal and external requirements must be identified and prioritized. Customer needs should be a priority, and research on technical feasibility is also important. This stage lays the foundation for subsequent testing and is therefore critical. At this stage, AI can help engineers by analyzing historical data and market trends to foresee potential problems and optimize test plans.
Design and development phase
During the design and development phase, it is critical to prototype the hardware circuit in steps and perform test verification to ensure that the product is built effectively. During this phase, designers will design, code, and develop firmware or embedded hardware and software.
The initial test validation will also track the performance of each function to ensure that it meets customer requirements. AI technology can automatically generate various test cases and scenarios based on design specifications and use cases, ensuring comprehensive coverage of test cases and reducing the risk of human error.
Test and verification phase
The so-called test verification is to conduct detailed performance testing in the final stage of hardware development. However, most of the final verification tests usually occur in the final product certification stage to ensure that the hardware and embedded software meet the requirements of customers, internal companies, laws and regulations, and regulatory authorities, and obtain corresponding certification.
Testing at this stage must be comprehensive. AI-driven testing tools can significantly enhance the effectiveness of this phase by automating repetitive tasks, analyzing massive amounts of test data, and locking in patterns that may identify potential defects. After completing test verification and product certification, the data needs to be analyzed to ensure that the new product fully meets the various specifications required for product release.
Development and maintenance phase
After the product is released, it will enter the production assembly and testing process to complete the product order. Post-deployment testing and continuous monitoring are essential to always ensure the quality level of the product. AI technology can monitor the performance of the product in real time, detect abnormal conditions and predict maintenance needs. This continuous feedback loop helps to achieve iterative updates of the product.
Integrating AI technology into product testing has many benefits
Many companies around the world are using AI technology in many application areas, including product testing. Launching high-quality products to the market quickly means standing out from the competition and winning more market share. Using AI technology to perform product testing throughout the product life cycle is very beneficial.
Higher efficiency
Product testing can be tedious, especially when testing complex products such as cars or even modern washing machines. These products are often equipped with many sensors, moving parts, input and output interfaces, and graphical display user interfaces. This is where AI comes in, as it can collect data and run it through specific algorithms to develop a set of optimized test cases that can be applied to any environment. Therefore, using AI technology can make the testing process more efficient and significantly reduce the testing time.
Higher accuracy
Product testing can be used to measure the performance of digital or analog electrical signals, sound, vibration, mechanics, or visual information. AI can process this data if the machine learning algorithm can interpret the data, learn and understand the expected results, and then take action to improve the data to improve the accuracy of the inspection. Compared with the more traditional, manually operated visual inspection test process, AI technology can significantly enhance defect detection capabilities and reduce human errors, and the advantages are very obvious.
Reduce costs
AI-driven testing tools can help humans by intelligently managing repetitive and time-consuming tasks. They can perform many offline tasks for engineers, such as test scheduling, batch testing, maximizing test equipment utilization, automatic test configuration and execution, data processing, etc. By automating various tasks, AI can greatly reduce the testing work that originally required manual execution, thereby reducing costs.
Enhanced scalability
Product testing usually requires the collection of a large amount of test data, which, if used improperly, often results in information waste. One of the great advantages of AI is that it can be scaled accordingly based on the complexity of the product and the application scenarios of the end user. In other words, AI can be expanded to manage large amounts of test data and dynamically adapt to complex test scenarios. One of the results of this is the creation of value for end users by providing high-quality products.
Using AI technology for product testing
During product testing, test engineers can tailor a variety of AI technologies and algorithms based on their specific use cases and the architecture of existing test data. If it is a new project, test engineers can also flexibly create models and data architectures from scratch. These AI algorithms can generally be divided into three categories: supervised learning algorithms, unsupervised learning algorithms, and reinforcement learning algorithms.
Supervised Learning Algorithms
As the name implies, the algorithm is a supervised machine learning and training method that can receive labeled data and train it to predict results that can be derived from other data. In this process, experts who are familiar with the original data and understand good results are needed to help the AI algorithm catch some false information.
These well-known supervised learning algorithms include decision tree algorithm, naive Bayes algorithm and support vector machine algorithm. Users can use these algorithms to get classification results. Classification means the result is yes or no, one or two, pass or fail. These algorithms can also classify data points into multiple categories.
Like the linear regression algorithm, the supervised learning algorithm also constructs a linear regression equation by setting the X-axis and Y-axis on the graph to obtain the data series to best explain the relationship between the independent variable and the dependent variable. The X-axis is the independent variable and the Y-axis is the dependent variable. The linear regression equation generated by the algorithm can help people deduce and predict possible data in the future.
Unsupervised learning algorithms
Some algorithms, such as K-Means clustering and Gaussian mixture models, do not require supervised learning. They do not require labeled data, but instead classify data into pre-defined clusters based on proximity, thereby creating models, analyzing relationships between data, and deriving valuable insights and insights from them.
Reinforcement Learning Algorithms
Reinforcement learning algorithms are the third type of machine learning algorithms that use the received reward signal as feedback to evaluate the impact of their actions in the environment. This type of machine learning algorithm is a repetitive learning process that requires an agent to perform actions and an external execution environment.
The cyclical learning process begins when the environment sends a state signal to the agent, prompting it to perform a specific action. After the agent performs the action, the external environment will send a reward signal to tell the agent what the result is, so that it can update and evaluate the previous action. With this new information, the agent can take new actions again. This cycle repeats until the environment sends a stop signal.
Previous article:Advanced training and demonstration of signal analysis software imc FAMOS (9.24)
Next article:[PSIJ Test Application Solution] Exploring the Mystery of PSIJ—High-Speed Signal Jitter Caused by Power Supply
Recommended ReadingLatest update time:2024-11-16 09:34
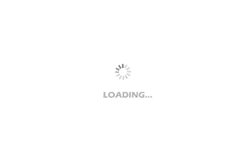
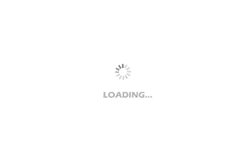
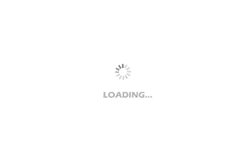
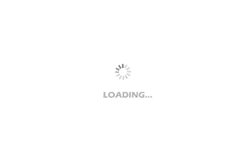
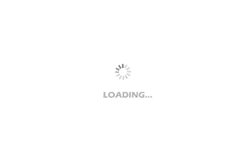
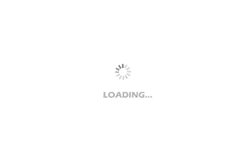
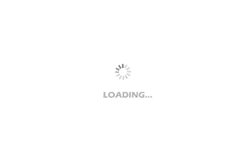
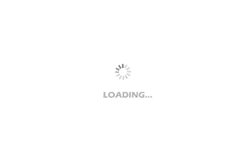
- Popular Resources
- Popular amplifiers
- Keysight Technologies Helps Samsung Electronics Successfully Validate FiRa® 2.0 Safe Distance Measurement Test Case
- From probes to power supplies, Tektronix is leading the way in comprehensive innovation in power electronics testing
- Seizing the Opportunities in the Chinese Application Market: NI's Challenges and Answers
- Tektronix Launches Breakthrough Power Measurement Tools to Accelerate Innovation as Global Electrification Accelerates
- Not all oscilloscopes are created equal: Why ADCs and low noise floor matter
- Enable TekHSI high-speed interface function to accelerate the remote transmission of waveform data
- How to measure the quality of soft start thyristor
- How to use a multimeter to judge whether a soft starter is good or bad
- What are the advantages and disadvantages of non-contact temperature sensors?
- Innolux's intelligent steer-by-wire solution makes cars smarter and safer
- 8051 MCU - Parity Check
- How to efficiently balance the sensitivity of tactile sensing interfaces
- What should I do if the servo motor shakes? What causes the servo motor to shake quickly?
- 【Brushless Motor】Analysis of three-phase BLDC motor and sharing of two popular development boards
- Midea Industrial Technology's subsidiaries Clou Electronics and Hekang New Energy jointly appeared at the Munich Battery Energy Storage Exhibition and Solar Energy Exhibition
- Guoxin Sichen | Application of ferroelectric memory PB85RS2MC in power battery management, with a capacity of 2M
- Analysis of common faults of frequency converter
- In a head-on competition with Qualcomm, what kind of cockpit products has Intel come up with?
- Dalian Rongke's all-vanadium liquid flow battery energy storage equipment industrialization project has entered the sprint stage before production
- Allegro MicroSystems Introduces Advanced Magnetic and Inductive Position Sensing Solutions at Electronica 2024
- Car key in the left hand, liveness detection radar in the right hand, UWB is imperative for cars!
- After a decade of rapid development, domestic CIS has entered the market
- Aegis Dagger Battery + Thor EM-i Super Hybrid, Geely New Energy has thrown out two "king bombs"
- A brief discussion on functional safety - fault, error, and failure
- In the smart car 2.0 cycle, these core industry chains are facing major opportunities!
- The United States and Japan are developing new batteries. CATL faces challenges? How should China's new energy battery industry respond?
- Murata launches high-precision 6-axis inertial sensor for automobiles
- Ford patents pre-charge alarm to help save costs and respond to emergencies
- New real-time microcontroller system from Texas Instruments enables smarter processing in automotive and industrial applications
- [Lazy self-care fish tank control system] Control GPIO pins in RTE mode of KEIL environment
- msp430f5529lp driver problem
- [Evaluation of domestic FPGA Gaoyun GW1N-4 series development board]——3. Turn on a lamp hello_led
- When opening the project, it prompts that there is no RAA305190GBM_common.xml
- View the sea - View southern Zhejiang - View Cangnan
- Could you please help me with how to calculate the VGS of this current mirror? Thank you very much!
- GigaDevice's new GD32F470xx series with TFT and Ethernet port
- MicroPython adds support for operator @
- I would like to ask you about the ODB protocol dedicated chip
- 【GD32L233C-START Review】1. Unboxing