Difficulty in long-term prediction: In long-term prediction (at least 3 seconds), the accumulation of small errors in the early stage may lead to large deviations from the true trajectory, reducing the prediction accuracy.
Adaptability to complex environments: In complex dynamic environments such as intersections, roundabouts, and busy urban areas, the model needs to handle complex interactions between multiple subjects, which increases the difficulty of model design.
Generalization and model limitations: The model must maintain accurate predictions in unseen driving scenarios and vehicle interactions. Although physics-based models perform well in simple scenarios and short-term predictions, they lack the ability to describe complex interactions and strategy diversity, and have difficulty coping with dynamic changes, which limits their predictions in long-term and complex environments.
Robustness: Data noise and uncertainty are also major problems. The inherent tracking errors and positioning deviations in real perception data require the algorithm to be robust and able to effectively handle imperfect information to ensure the reliability of the prediction.
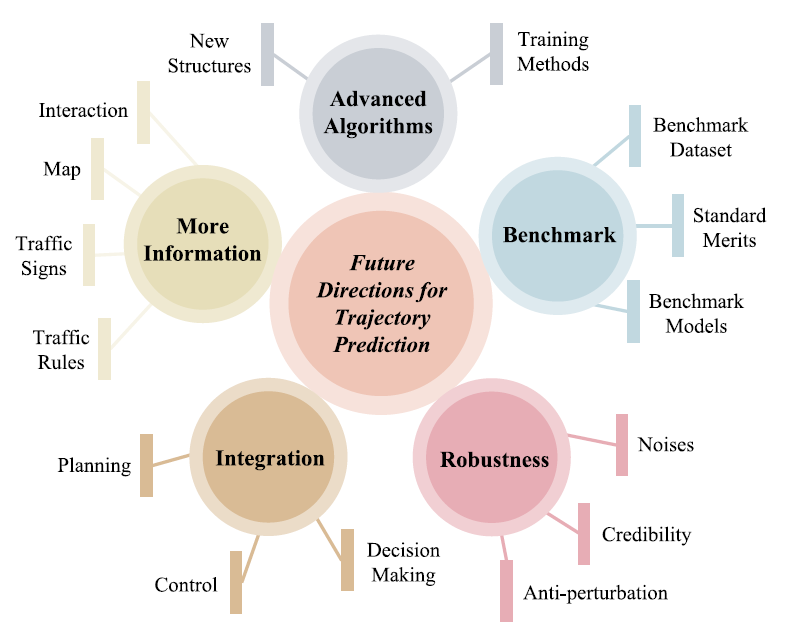
In terms of future research directions, the priority is to enhance the interactive perception and environmental adaptability of the model. By combining deep learning technologies such as graph neural networks (GNNs) and attention mechanisms, we can capture complex interactive features at a deeper level, while taking into account road information and traffic rules to achieve more realistic predictions. The fusion of high-precision maps and vector maps will be the key to improving prediction accuracy, using map information to more accurately reflect road structures, guide predictions, and reduce error rates, especially for long-term predictions.
Secondly, multi-model fusion and hybrid methods are the trend, combining the immediacy of physical models with the generalization ability of learning models, such as policy optimization based on reinforcement learning, to achieve smarter predictions. Online learning and adaptability enable the model to learn new data during operation, adapt to changes, and improve the long-term accuracy of generalization and prediction. At the same time, computing efficiency optimization, lightweight, model compression and hardware acceleration strategies ensure the real-time performance of the algorithm in the vehicle.
Finally, the establishment of a standard evaluation system and data set is crucial. Building a diverse, realistic, and complex benchmark data set, and standardizing evaluation indicators, including accurate metrics for multimodal predictions, will facilitate fair comparisons and advance validation models, and promote technological development. Taking uncertainty metrics into account, such as probability assessment, to reflect the model's credibility in predictions, will be a focus of future research.
References
[1] Bharilya V, Kumar N. Machine learning for autonomous vehicle's trajectory prediction: A comprehensive survey, challenges, and future research directions[J]. Vehicular Communications, 2024: 100733.
[2] Huang Y, Du J, Yang Z, et al. A survey on trajectory-prediction methods for autonomous driving[J]. IEEE Transactions on Intelligent Vehicles, 2022, 7(3): 652-674.
[3] Liu J, Mao X, Fang Y, et al. A survey on deep-learning approaches for vehicle trajectory prediction in autonomous driving[C]//2021 IEEE International Conference on Robotics and Biomimetics (ROBIO). IEEE, 2021: 978-985.
[4] Singh A. Trajectory-Prediction with Vision: A Survey[C]//Proceedings of the IEEE/CVF International Conference on Computer Vision. 2023: 3318-3323.
[5] Leon F, Gavrilescu M. A review of tracking, prediction and decision making methods for autonomous driving[J]. arxiv preprint arxiv:1909.07707, 2019.
Previous article:With large investments pouring in, where will sensors go next?
Next article:In this field, the whole machine manufacturers are in a frenzy of internal competition, while the chip manufacturers are constantly raising funds
- Popular Resources
- Popular amplifiers
- Car key in the left hand, liveness detection radar in the right hand, UWB is imperative for cars!
- After a decade of rapid development, domestic CIS has entered the market
- Aegis Dagger Battery + Thor EM-i Super Hybrid, Geely New Energy has thrown out two "king bombs"
- A brief discussion on functional safety - fault, error, and failure
- In the smart car 2.0 cycle, these core industry chains are facing major opportunities!
- The United States and Japan are developing new batteries. CATL faces challenges? How should China's new energy battery industry respond?
- Murata launches high-precision 6-axis inertial sensor for automobiles
- Ford patents pre-charge alarm to help save costs and respond to emergencies
- New real-time microcontroller system from Texas Instruments enables smarter processing in automotive and industrial applications
- Innolux's intelligent steer-by-wire solution makes cars smarter and safer
- 8051 MCU - Parity Check
- How to efficiently balance the sensitivity of tactile sensing interfaces
- What should I do if the servo motor shakes? What causes the servo motor to shake quickly?
- 【Brushless Motor】Analysis of three-phase BLDC motor and sharing of two popular development boards
- Midea Industrial Technology's subsidiaries Clou Electronics and Hekang New Energy jointly appeared at the Munich Battery Energy Storage Exhibition and Solar Energy Exhibition
- Guoxin Sichen | Application of ferroelectric memory PB85RS2MC in power battery management, with a capacity of 2M
- Analysis of common faults of frequency converter
- In a head-on competition with Qualcomm, what kind of cockpit products has Intel come up with?
- Dalian Rongke's all-vanadium liquid flow battery energy storage equipment industrialization project has entered the sprint stage before production
- Allegro MicroSystems Introduces Advanced Magnetic and Inductive Position Sensing Solutions at Electronica 2024
- Car key in the left hand, liveness detection radar in the right hand, UWB is imperative for cars!
- After a decade of rapid development, domestic CIS has entered the market
- Aegis Dagger Battery + Thor EM-i Super Hybrid, Geely New Energy has thrown out two "king bombs"
- A brief discussion on functional safety - fault, error, and failure
- In the smart car 2.0 cycle, these core industry chains are facing major opportunities!
- The United States and Japan are developing new batteries. CATL faces challenges? How should China's new energy battery industry respond?
- Murata launches high-precision 6-axis inertial sensor for automobiles
- Ford patents pre-charge alarm to help save costs and respond to emergencies
- New real-time microcontroller system from Texas Instruments enables smarter processing in automotive and industrial applications
- Share msp430f5529 pwm programming example
- STM32F765@216MHz output I2S test ES9023P audio listening experience [code] [video] [firmware]
- MSP430F1101A MCU button and LED detection program
- 【Badminton Training Monitor Project】--Project Introduction and R&D Plan
- Application example of using 555 to realize timing function
- How does TI's battery management chip complete the development process and obtain mass production documents?
- 【Mil MYX-1028X】(IV) Network Communication Demo
- Today's live broadcast at 10:00: Introduction to ST machine learning, bone vibration, and SensorTile.box sensor development kit
- Network Camera Data/Port Forwarding Hardware Design Solution
- [Comic] Confession of a PCB layout engineer!