Based on different working principles, the existing CH4 concentration detection methods mainly include: Ostwald gas detection method, catalytic combustion method, and infrared spectroscopy detection method. At present, the infrared multi-wavelength method is more commonly used to predict the CH4 concentration in biogas. However, this method will also bring new problems. For example: when detecting the CH4 concentration in biogas, the high amount of CO2 in the biogas will affect the output of the CH4 channel and the reference channel, thereby affecting the prediction accuracy of CH4. Therefore, this article discusses the use of data processing methods to improve the prediction accuracy of CH1 concentration.
At present, the commonly used CH4 concentration prediction algorithms include linear interpolation, multiple regression, and neural network (NN). Among them, linear interpolation and multiple regression are traditional algorithms with low accuracy; neural networks require a large number of samples to fit a better model and are prone to fall into local minimum points. The support vector machine (SVM) discussed in this paper specifically solves the small sample problem and seeks the global optimal solution based on the principle of minimizing structural risk.
When predicting the CH4 concentration in biogas, this paper attempts for the first time to use temperature as an important factor affecting the detector output. A temperature chamber is introduced to control the ambient temperature of the detector to obtain 693 samples at different temperatures. The temperature and the detector output are used as the input of SVM to establish a CH4 prediction model and obtain ideal prediction accuracy.
1 Introduction to Support Vector Machine
SVM is a machine learning method that greatly improves the generalization ability of the learning machine based on the principle of structural risk minimization. It transforms the optimization problem into a convex quadratic programming problem. The solution obtained by the quadratic programming is unique and globally optimal, so there is no local extreme value problem of general neural networks.
Regression support vector machine (SVR) is the application of support vector machine in regression learning. Its basic idea is: for a given training sample point {(x1, y1), (x2, y2), ..., (xn, yn)}, a function f(x) is regressed through SVR training, so that the output value of each input sample obtained by the function and the target value corresponding to the input sample do not differ by more than the error e, and the regressed function is as smooth as possible, which can overcome the shortcomings of low prediction accuracy of traditional linear interpolation and multivariate regression methods, solve the impact of high concentration CO2 on the output of CH4 channel and reference channel, and has better generalization ability than NN. In addition, temperature has a certain influence on the output of gas detectors. This paper studies the use of temperature as one of the inputs of the model, and the voltage output of the multi-channel detector as the input of SVM to achieve CH4 concentration prediction.
2. Acquisition of experimental data
2.1 System structure and infrared multi-wavelength detection
The hardware system structure is shown in Figure 1. After the high-pressure standard gas CH4 in the cylinder is reduced in pressure by the pressure reducing valve, it flows to the flow meter 1 in the gas distribution box through the gas pipeline. The gas distribution box further controls and measures the flow rate of the gas in the flow meter 1 through its own computer software. After flowing out of the gas distribution box, it is mixed with CO2 and N2 (dilution effect, non-heteronuclear molecules, and little effect on the CH4 channel) passing through flow meters 2 and 3 in a fixed ratio, and then enters the incubator. The biogas detection circuit board in the incubator includes a multi-channel detector (CH4, CO2), reference end) and the corresponding signal processing circuit. After passing through the detection gas chamber, the gas is discharged to the fume hood.
The above-mentioned biogas detection is based on the principle of infrared absorption, as shown in FIG2 .
Gases with heteronuclear molecular structures (molecules composed of different types of chemical atoms) have selective absorption characteristics for infrared rays in the mid-infrared band. The characteristic absorption peak wavelengths of CH4 are 3.32μm and CO2 are 4.24μm. The front ends of the CH4 and CO2 channels of the detector are equipped with filters for the characteristic absorption of CH4 and CO2, respectively. The detector outputs a voltage after receiving infrared radiation. The greater the gas concentration during detection, the stronger the absorption of infrared light of its characteristic wavelength, and the smaller the corresponding detection voltage. The infrared photons that can pass through the reference end filter are almost not absorbed by the gas, which can best reflect the light source information and the pollution of the gas chamber. In practice, the filter of the detector is limited by the process, and the skirt of its transmission spectrum cannot completely avoid the cross-sensitive area of the two gas absorption zones. Therefore, when the gas concentration is high, interference problems will occur. For example, the characteristic absorption peak wavelength of CH4 in Figure 2 is 3.32μm, but there is also absorption between 4.2 and 4.4μm. This paper uses SVM to solve this cross-interference problem.
2.2 Experimental data acquisition process
(1) Assemble the experimental system according to the structure in Figure 1;
(2) Set the incubator temperature to T1 and wait for 2 hours to allow the temperature to remain constant;
(3) Set the flow rates of the three flow meters to 0, open the host computer data acquisition software, and prepare for data acquisition;
(4) Open the pressure reducing valve of the gas cylinder to allow the gas to flow out;
(5) According to the gas concentration Ci to be configured, set the flow rate of flow meters 1, 2, and 3 respectively; when the sensor output is stable, obtain the output values of CH4, CO2, and the reference end and save them;
(6) The calibration range of CH4 concentration is 0% to 100% (0%, 1%, 2%, ..., 100%, a total of 19 calibration points), and CO2 is 0% to 100% (0%, 0.5%, 1%, ..., 100%, a total of 23 calibration points). During the actual calibration, first fix the CH4 concentration and continuously change the CO2 concentration to obtain a set of measurement values; then change the CH4 concentration to another fixed value, and then continuously change the CO2 concentration to obtain another set of measurement values; repeat (5) to collect the detector output values of different gas concentration combinations;
(7) Change the incubator temperature Ti, repeat (2) to (6), and obtain data at different temperatures.
Through the above data collection process, 693 sets of test data at 0℃, 25℃, 40℃, and 50℃ were finally obtained to prepare for SVR. The experimental data are shown in Table 1. The U1, U2, and Ur rows represent the A/D values of CH4, CO2, and the reference end, respectively.
3 CH4 concentration prediction algorithm based on SVM
3.1 Establishment of SVM prediction model
From the above analysis, we can see that the reference end (Ref detection end) can best reflect the light source information; U1/Ur, U2/Ur can better reflect the degree of absorption of CH4 and CO2 to their characteristic photons compared with U1 and U2; for the same component of biogas mixture, when the temperature is different, the outputs of several channels of the sensor are not in the same number domain. In view of the fact that SVM is good at solving small sample and nonlinear problems and has good generalization ability, the temperature T, U1/Ur, U2/Ur are used as the three-dimensional input of the support vector machine to establish the SVM prediction block diagram of CH4 concentration, as shown in Figure 3. The output of SVM is the predicted value of CH4 concentration.
Common kernel functions of SVM include polynomial, radial basis function (RBF), Sigmoid, etc. Among them, Gauss radial basis function can better simulate the characteristic information of spectral signal such as spectral peak, so RBF kernel function is selected. The parameters of SVM are σ2 and γ. If σ2 is too large, the model will converge prematurely; γ controls the degree of punishment for samples that exceed the error. (σ2, γ) is selected by repeated trials. After the model is established, T, U1/Ur, and U2/Ur of the test sample are substituted into the model for calculation, and the model prediction value is compared with the experimental calibration value until the model prediction accuracy requirements are met. This paper finally determines σ2=10 000 and γ=1.
3.2 Results and Analysis
Among the 693 samples obtained, 543 were randomly selected as training samples and 150 as test samples. The proposed method was compared with several methods including Linear Interpolation (LI), Multiple Regression (MR), BP neural network, RBF neural network, and SVM-noT without temperature as input. The specific methods are as follows:
(1) Linear interpolation method: Based on the known points (x01, y01), (x02, y02), …, (xon, y0n), a y = A x model (A is the coefficient matrix) is established according to the spatial linear relationship to solve for y11, y12, …, y1n corresponding to x11, x12, …, x1n;
(2) Polynomial regression method: Based on the known points (x01, y01), (x02, y02), ..., (x0n, y0n), a polynomial model y = f(x) is established according to the least squares principle, and then y11, y12, ..., y1n corresponding to x11, x12, ..., x1n are solved;
(3) BP neural network method: A multi-layer forward neural network based on the error back propagation algorithm is used to establish the input and output model of known samples. In this paper, the BP neural network model is established with temperature T, U1/Ur, and U2/Ur as input and the predicted concentration of CH4 as output;
(4) RBF neural network method: a type of forward neural network based on function approximation theory and with radial basis function as the transfer function, which can fit the best model in multidimensional space. In this paper, the RBF neural network model is established with temperature T, U1/Ur, U2/Ur as input and the predicted concentration of CH4 as output;
(5) SVM-noT method: The influence of temperature factor is not considered. That is, the “temperature T” input at the front end of the “SVM module” in FIG3 is removed, and only the two-element inputs U1/Ur and U2/Ur are retained to establish the input and output model.
During the test, x1j is used as the input of the model established by the above five methods, and the output is the predicted concentration of CH4, which can verify the accuracy of these models. The vector xij=[T, U1/Ur, U2/Ur], i=0,1;j=1,2,…,n;x0j is the training sample, x1j is the test sample,
To predict the concentration. When comparing the SVM method considering temperature in this paper with the first five methods, the maximum absolute error and the absolute average error of the prediction results are used for comparison. The simulation results of the six methods are shown in Figure 4.
As can be seen from the figure: SVM is better than MR, RBF, BP, LI and SVM-not methods, with the smallest error and the best prediction accuracy. The kernel function of the SVM model can better simulate the characteristic information such as the spectral peak of the spectral signal, fully consider the impact of other components in biogas on the CH4 channel output, and achieve better nonlinear mapping of U1/Ur, U2/Ur, temperature T to CH4 concentration values, and has better generalization ability.
4 Conclusion
Aiming at the problem of predicting CH4 concentration in biogas, an experimental system was built, a large amount of experimental data was obtained, and a method of predicting CH4 concentration by taking temperature T, the output of CH4 and CO2 detectors as the input of SVM was discussed. This method was compared with the five commonly used methods of LI, MR, BP, RBF, and SVM-noT. The experimental results show that the proposed method has high prediction accuracy and provides a good idea for predicting CH4 concentration in biogas.
Previous article:Comparison and analysis of exploratory testing and manual testing
Next article:Linear prediction and its implementation in Matlab
Recommended ReadingLatest update time:2024-11-16 15:04
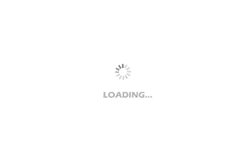
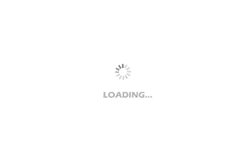
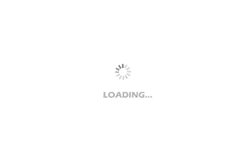
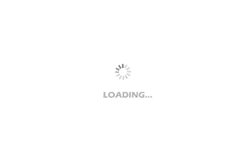
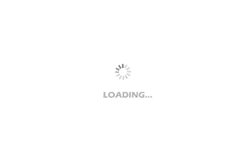
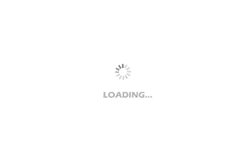
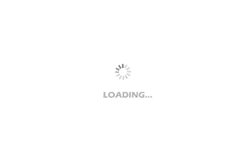
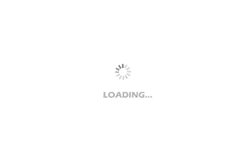
- Popular Resources
- Popular amplifiers
-
Operational Amplifier Practical Reference Handbook (Edited by Liu Changsheng, Zhao Mingying, Liu Xu, etc.)
-
Detailed explanation of big data technology system: principles, architecture and practice (Dong Xicheng)
-
Power Electronics System Modeling and Control, edited by Xu Dehong, a teaching book for postgraduates published by the Machinery Industry Press
-
Practice of Classic Machine Learning Algorithms
- Keysight Technologies Helps Samsung Electronics Successfully Validate FiRa® 2.0 Safe Distance Measurement Test Case
- From probes to power supplies, Tektronix is leading the way in comprehensive innovation in power electronics testing
- Seizing the Opportunities in the Chinese Application Market: NI's Challenges and Answers
- Tektronix Launches Breakthrough Power Measurement Tools to Accelerate Innovation as Global Electrification Accelerates
- Not all oscilloscopes are created equal: Why ADCs and low noise floor matter
- Enable TekHSI high-speed interface function to accelerate the remote transmission of waveform data
- How to measure the quality of soft start thyristor
- How to use a multimeter to judge whether a soft starter is good or bad
- What are the advantages and disadvantages of non-contact temperature sensors?
- Innolux's intelligent steer-by-wire solution makes cars smarter and safer
- 8051 MCU - Parity Check
- How to efficiently balance the sensitivity of tactile sensing interfaces
- What should I do if the servo motor shakes? What causes the servo motor to shake quickly?
- 【Brushless Motor】Analysis of three-phase BLDC motor and sharing of two popular development boards
- Midea Industrial Technology's subsidiaries Clou Electronics and Hekang New Energy jointly appeared at the Munich Battery Energy Storage Exhibition and Solar Energy Exhibition
- Guoxin Sichen | Application of ferroelectric memory PB85RS2MC in power battery management, with a capacity of 2M
- Analysis of common faults of frequency converter
- In a head-on competition with Qualcomm, what kind of cockpit products has Intel come up with?
- Dalian Rongke's all-vanadium liquid flow battery energy storage equipment industrialization project has entered the sprint stage before production
- Allegro MicroSystems Introduces Advanced Magnetic and Inductive Position Sensing Solutions at Electronica 2024
- Car key in the left hand, liveness detection radar in the right hand, UWB is imperative for cars!
- After a decade of rapid development, domestic CIS has entered the market
- Aegis Dagger Battery + Thor EM-i Super Hybrid, Geely New Energy has thrown out two "king bombs"
- A brief discussion on functional safety - fault, error, and failure
- In the smart car 2.0 cycle, these core industry chains are facing major opportunities!
- The United States and Japan are developing new batteries. CATL faces challenges? How should China's new energy battery industry respond?
- Murata launches high-precision 6-axis inertial sensor for automobiles
- Ford patents pre-charge alarm to help save costs and respond to emergencies
- New real-time microcontroller system from Texas Instruments enables smarter processing in automotive and industrial applications
- MSP430 MCU Development Record (12)
- EEWORLD University Hall----Choosing the latest boost converter and Class D amplifier from Texas Instruments to significantly increase the battery life of the trolley speakers
- Award-winning live broadcast | TI Embedded Live Broadcast Month is waiting for you [Low-power Wi-Fi MCU, Sitara AM57X platform, machine learning]
- SPI settings for msp430f149
- Please recommend a board
- Streamlining mobile phone system design
- EEWORLD University - High-Speed Transimpedence Amplifier Design Process
- PCB
- Please help analyze this statement IS_RCC_APB2_PERIPH(PERIPH)
- At the age of thirty, I am penniless and have nothing to lose. I have to start all over again!!!